Social Graph Transformer Networks for Pedestrian Trajectory Prediction in Complex Social Scenarios
Conference on Information and Knowledge Management(2022)
摘要
ABSTRACTPedestrian trajectory prediction is essential for many modern applications, such as abnormal motion analysis and collision avoidance for improved traffic safety. Previous studies still face challenges in embracing high social interaction, dynamics, and multi-modality for achieving high accuracy with long-time predictions. We propose Social Graph Transformer Networks for multi-modal prediction of pedestrian trajectories, where we combine Graph Convolutional Network and Transformer Network by generating stable resolution pseudo-images from Spatio-temporal graphs through a designed stacking and interception method. Specifically, we adopt adjacency matrices to obtain Spatio-temporal features and Transformer for long-time trajectory predictions. As such, we retrain the advantages of both, i.e., the ability to aggregate information over an arbitrary number of neighbors and to conduct complex time-dependent data processing. Our experimental results show that our model reduces the final displacement error and achieves state-of-the-art in multiple metrics. The module's effectiveness is demonstrated through ablation experiments.
更多查看译文
关键词
pedestrian trajectory prediction,networks,social
AI 理解论文
溯源树
样例
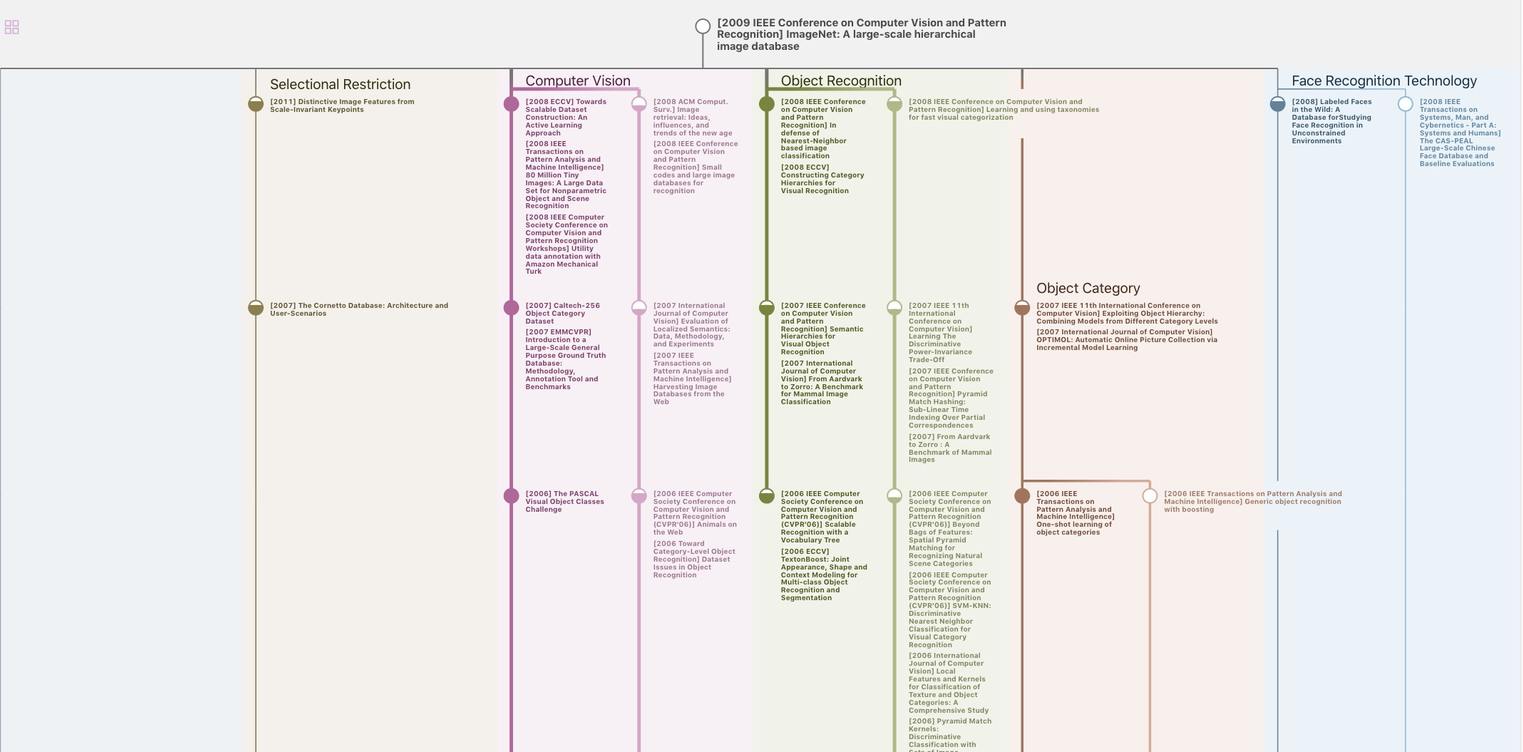
生成溯源树,研究论文发展脉络
Chat Paper
正在生成论文摘要