Mining the factor zoo: Estimation of latent factor models with sufficient proxies
arxiv(2023)
摘要
Latent factor model estimation typically relies on either using domain knowledge to manually pick several observed covariates as factor proxies, or purely conducting multivariate analysis such as principal component analysis. However, the former approach may suffer from the bias while the latter cannot incorporate additional information. We propose to bridge these two approaches while allowing the number of factor proxies to diverge, and hence make the latent factor model estimation robust, flexible, and statistically more accurate. As a bonus, the number of factors is also allowed to grow. At the heart of our method is a penalized reduced rank regression to combine information. To further deal with heavy-tailed data, a computationally attractive penalized robust reduced rank regression method is proposed. We establish faster rates of convergence compared with the benchmark. Extensive simulations and real examples are used to illustrate the advantages.
更多查看译文
关键词
C13,C55,C58,C38
AI 理解论文
溯源树
样例
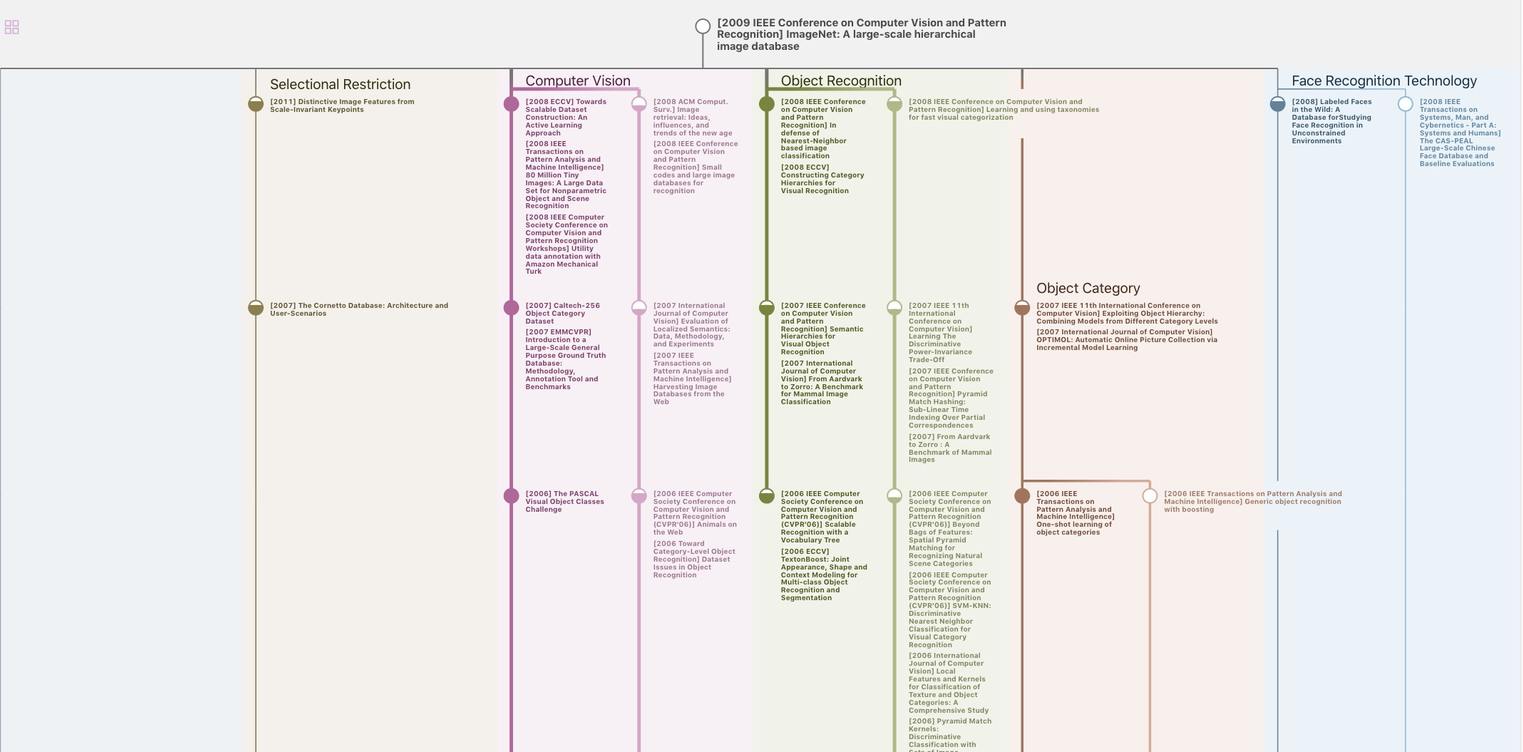
生成溯源树,研究论文发展脉络
Chat Paper
正在生成论文摘要