Contrastive Learning for Prediction of Alzheimer's Disease Using Brain 18F-FDG PET
IEEE Journal of Biomedical and Health Informatics(2022)
摘要
Brain 18F-FDG PET images are commonly-known materials for effectively predicting Alzheimer's disease (AD). However, the data volume of PET is usually insufficient, which is unfavorable to train an accurate AD prediction networks. Furthermore, the PET image is noisy with low signal-to-noise ratio, and simultaneously the feature (metabolic abnormality) used for predicting AD in PET image is not always obvious. Therefore, a contrastive-based learning method is proposed to address the challenges of PET image inherently possessed. Firstly, the slices of 3D PET image are amplified by cropping the image of anchors (i.e., an augmented version of the same image) to generate extended training data. Meanwhile, contrastive loss is adopted to enlarge inter-class feature distances and reduce intra-class feature differences using subject fuzzy labels as supervised information. Secondly, we construct a double convolutional hybrid attention module to enhance the network to learn different perceptual domains where two convolutional layers with different convolutional kernels (
$7\times 7$
and
$5\times 5$
) are constructed. Moreover, we recommend a diagnosis mechanism by analyzing the consistency of predicted result for PET slices alone with clinical neuropsychological assessment to achieve a better AD diagnosis. The experimental results show that the proposed method outperforms the state-of-the-arts for brain 18F-FDG PET images, and hence demonstrate the advantage of the method in effectively predicting AD.
更多查看译文
关键词
Alzheimer's disease (AD),deep learning,brain 18F-FDG PET,contrastive loss,multiattention mechanism,multi-correlation analysis
AI 理解论文
溯源树
样例
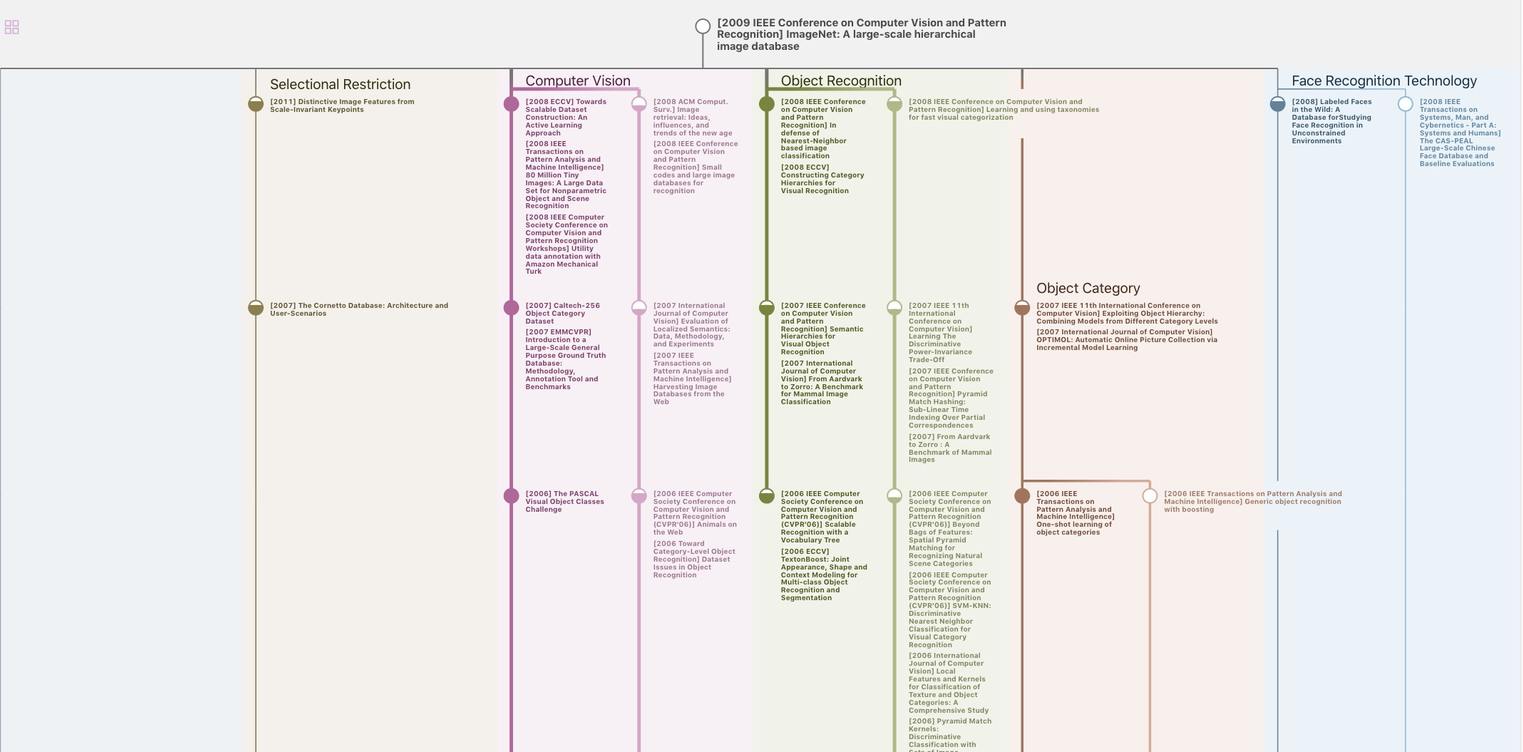
生成溯源树,研究论文发展脉络
Chat Paper
正在生成论文摘要