Block-diagonal test for high-dimensional covariance matrices
TEST(2022)
摘要
The structure testing of a high-dimensional covariance matrix plays an important role in financial stock analyses, genetic series analyses, and many other fields. Testing that the covariance matrix is block-diagonal under the high-dimensional setting is the main focus of this paper. Several test procedures that rely on normality assumptions, two-diagonal block assumptions, or sub-block dimensionality assumptions have been proposed to tackle this problem. To relax these assumptions, we develop a test framework based on U-statistics, and the asymptotic distributions of the U-statistics are established under the null and local alternative hypotheses. Moreover, a test approach is developed for alternatives with different sparsity levels. Finally, both a simulation study and real data analysis demonstrate the performance of our proposed methods.
更多查看译文
关键词
Block-diagonal structure,High-dimensional covariance matrix,U-statistic
AI 理解论文
溯源树
样例
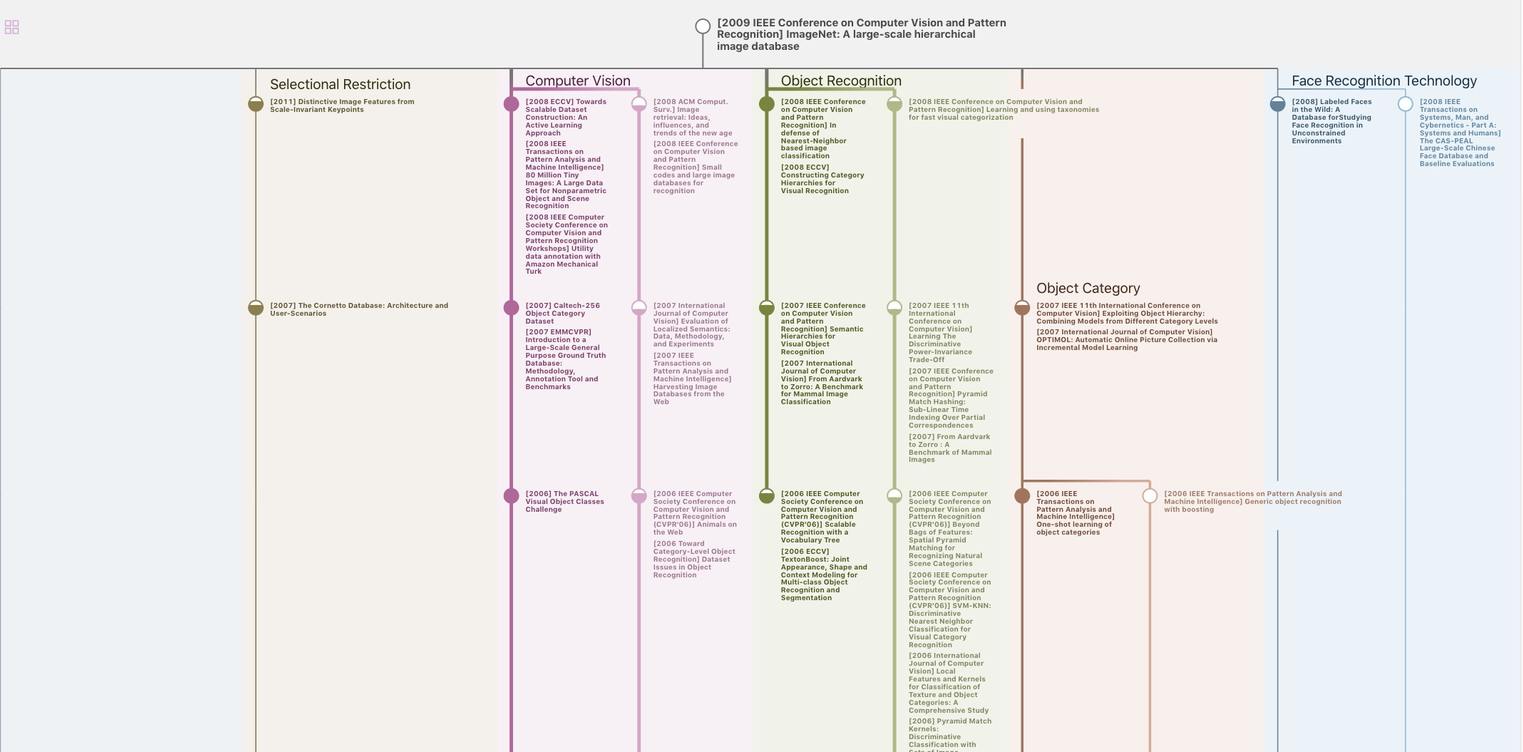
生成溯源树,研究论文发展脉络
Chat Paper
正在生成论文摘要