391 Application of a machine learning-based decision support tool in injury surveillance system
Abstracts(2022)
摘要
Background
Emergency department (ED)-based injury surveillance systems across many countries face resourcing challenges related to the validation and coding of data, which largely rely on manual coding. This presentation describes the evaluation of a machine learning-based Decision Support Tool (DST) to assist injury surveillance departments in the validation, coding and use of their data.Methods
Manually-coded Queensland Injury Surveillance Unit (QISU) data has been used to develop, train and iteratively refine a machine learning-based classifier to enable semi-automated coding of injury narrative data. This paper describes a trial implementation of the machine learning-based DST in the QISU workflow using a major pediatric hospital’s ED data comparing outcomes in coding time and accuracy pre and post-implementation.Results
In total, 3174 injury records in February and March 2020 were analyzed. Statistical analysis shows a 10% reduction in manual coding time after introducing the DST. Concordance study comparing the kappa statistics from both DST-assisted and unassisted data shows increases in accuracy across three data fields; injury intent (85.4% unassisted vs. 94.5% assisted), external cause (88.8% unassisted vs. 91.8% assisted) and injury factor (89.3% unassisted vs. 92.9% assisted). The classifier was also used to produce a timely report monitoring injury patterns during the COVID-19 pandemic. Hence, it has the potential for near real-time surveillance of emerging hazards to inform public health responses.Conclusion
The integration of the DST into the QISU workflow shows benefits to the unit as it facilitates timely reporting and acts as a DST in the manual coding process.查看译文
关键词
injury surveillance system,decision support tool,learning-based
AI 理解论文
溯源树
样例
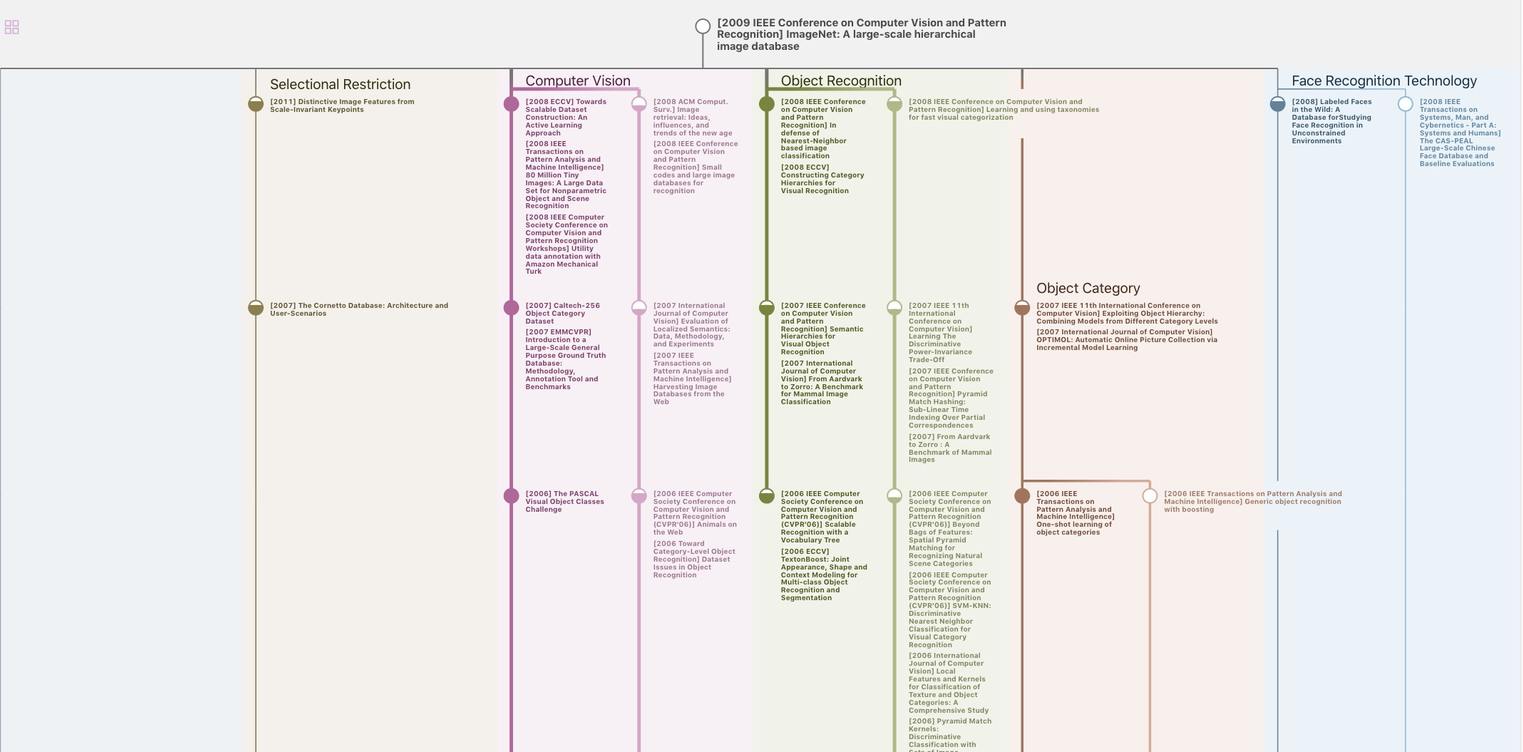
生成溯源树,研究论文发展脉络
Chat Paper
正在生成论文摘要