Hybrid Variable Monitoring Mixture Model for Anomaly Detection in Industrial Processes
IEEE TRANSACTIONS ON CYBERNETICS(2024)
摘要
Effective process monitoring is both a prerequisite and a guarantee for high system reliability. In modern industrial processes, binary variables may appear together with continuous variables, making process monitoring more intractable. Recently, a model named hybrid variable monitoring (HVM) has been proposed to conduct anomaly detection with both continuous and binary variables. Although the performance of HVM has been significantly improved after using the information of binary variables, it assumes that every continuous variable obeys a single Gaussian distribution and each binary variable obeys a single Bernoulli distribution. It is difficult for practical processes to satisfy such strict assumptions. To overcome this problem, this study proposes an improved algorithm called HVM mixture model (HVMMM). The HVMMM contains multiple components with the assumption of an HVM for every component. Compared with the HVM, the HVMMM is suitable for more general situations and has a more accurate characterization of the data features. Subsequently, the expectation-maximization (EM) algorithm is adopted for parameter learning for multiple components. The mathematical expressions of the parameters are derived in detail. In addition, the improvement on the monitoring performance caused by multiple components is analyzed. Finally, a numerical example and a practical case are used to demonstrate the effectiveness and efficiency of HVMMM. After multiple components are considered, the fault detection rate increases by 5.49% in the numerical example and the false alarm rate reduces by 1.6% in the practical case.
更多查看译文
关键词
Principal component analysis,Process monitoring,Mixture models,Gaussian distribution,Automation,Anomaly detection,Standards,Fault detection,hybrid variable monitoring mixture model (HVMMM),hybrid variable,process monitoring
AI 理解论文
溯源树
样例
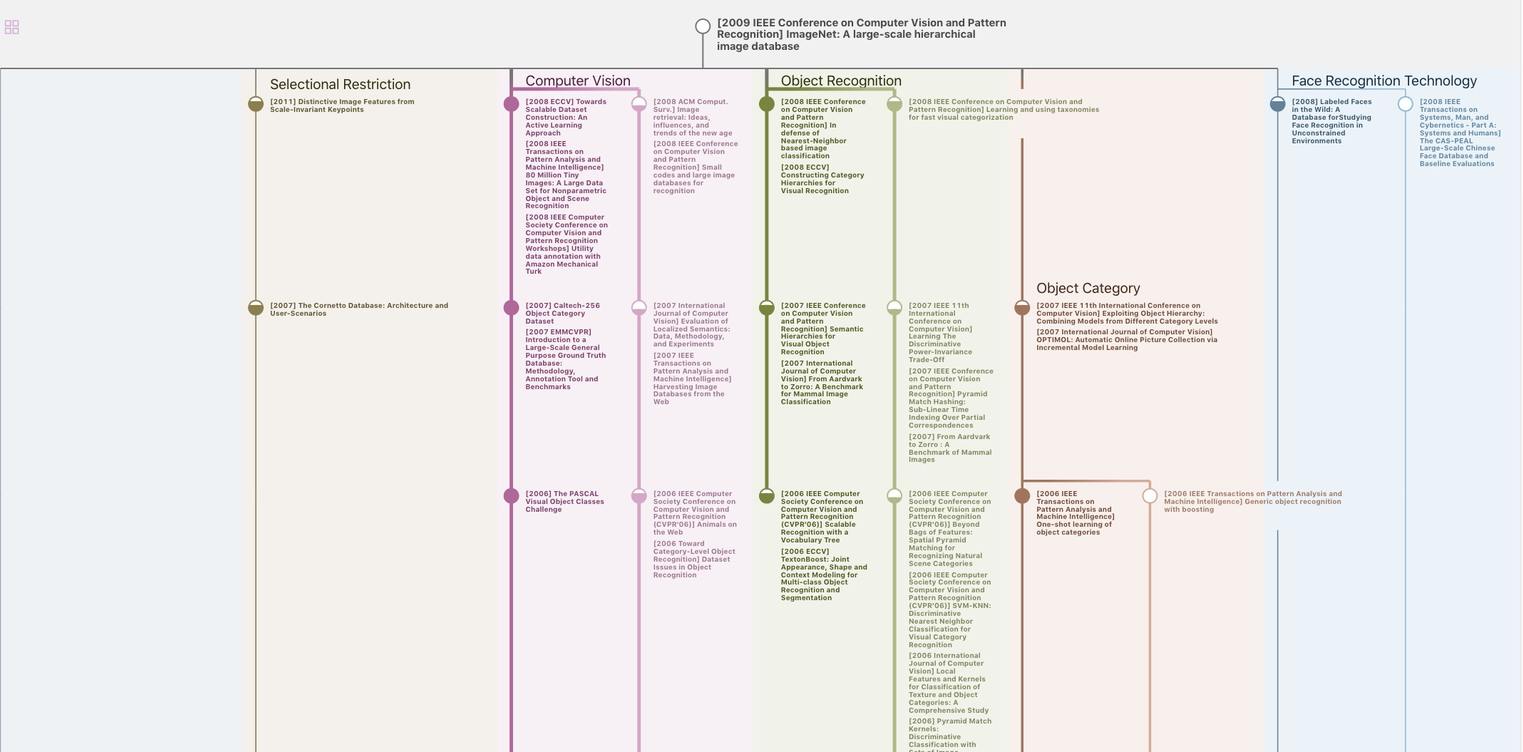
生成溯源树,研究论文发展脉络
Chat Paper
正在生成论文摘要