Machine learning methods applied for the prediction of biological activities of triple reuptake inhibitors.
Journal of biomolecular structure & dynamics(2022)
摘要
Major depressive disorder (MDD) is characterized by a series of disabling symptoms like anhedonia, depressed mood, lack of motivation for daily tasks and self-extermination thoughts. The monoamine deficiency hypothesis states that depression is mainly caused by a deficiency of monoamine at the synaptic cleft. Thus, major efforts have been made to develop drugs that inhibit serotonin (SERT), norepinephrine (NET) and dopamine (DAT) transporters and increase the availability of these monoamines. Current gold standard treatment of MDD uses drugs that target one or more monoamine transporters. Triple reuptake inhibitors (TRIs) can target SERT, NET, and DAT simultaneously, and are believed to have the potential to be early onset antidepressants. Quantitative structure-activity relationship models were developed using machine learning algorithms in order to predict biological activities of a series of triple reuptake inhibitor compounds that showed inhibitory activity against multiple targets. The results, using mostly interpretable descriptors, showed that the internal and external predictive ability of the models are adequate, particularly of the DAT and NET by Random Forest and Support Vector Machine models. The current work shows that models developed from relatively simple, chemically interpretable descriptors can predict the activity of TRIs with similar structure in the applicability domain using ML methods.Communicated by Ramaswamy H. Sarma.
更多查看译文
关键词
QSAR,Random Forest,Support Vector Machine,Triple reuptake inhibitors,computer aided-drug design,machine-learning
AI 理解论文
溯源树
样例
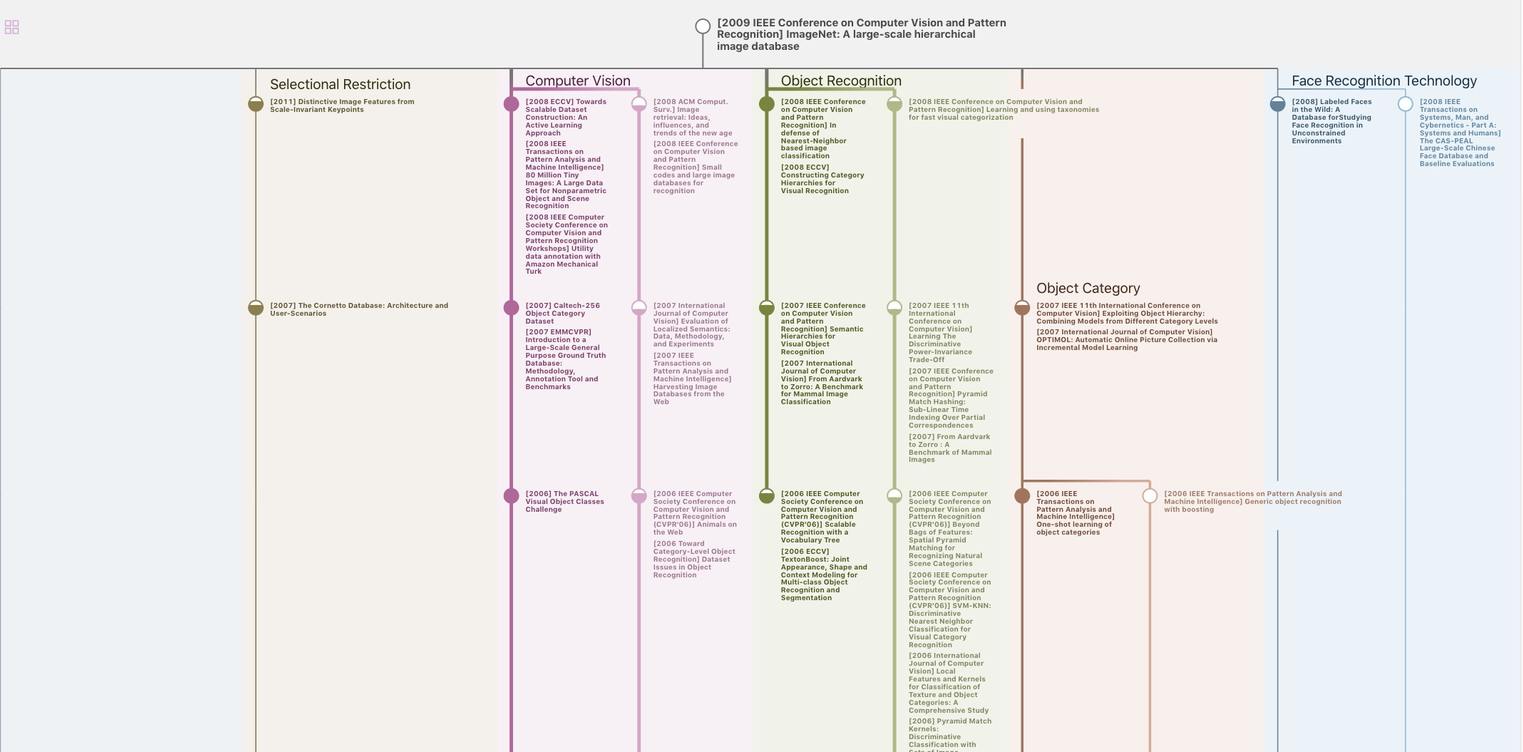
生成溯源树,研究论文发展脉络
Chat Paper
正在生成论文摘要