PeptideForest: Semisupervised machine learning integrating multiple search engines for peptide identification
biorxiv(2022)
摘要
The first step in bottom-up proteomics is the assignment of measured fragmentation mass spectra to peptide sequences, also known as peptide spectrum matches. In recent years novel algorithms have pushed the assignment to new heights, unfortunately, different algorithms come with different strengths and weaknesses and choosing the appropriate algorithm poses a challenge for the user. Here we introduce PeptideForest, a semi-supervised machine learning approach that integrates the assignments of multiple algorithms to train a random forest classifier to elevate that issue. Additionally, PeptideForest increases the number of peptide-to-spectrum matches that exhibit a q-value lower than 1% by 25.2 ± 1.6% compared to MS-GF+ data on samples containing mixed HEK and E. coli proteomes. However, an increase in quantity does not necessarily reflect an increase in quality and this is why we devised a novel approach to determine the quality of the assigned spectra through TMT quantification of samples with known ground truths. Thereby, we could show that the increase in PSMs below 1% q-value does not come with a decrease in quantification quality and as such PeptideForest offers a possibility to gain deeper insights into bottom-up proteomics. PeptideForest has been integrated into our pipeline framework Ursgal and can therefore be combined with a wide array of algorithms.
### Competing Interest Statement
The authors have declared no competing interest.
更多查看译文
关键词
peptideforest,multiple search engines,machine learning
AI 理解论文
溯源树
样例
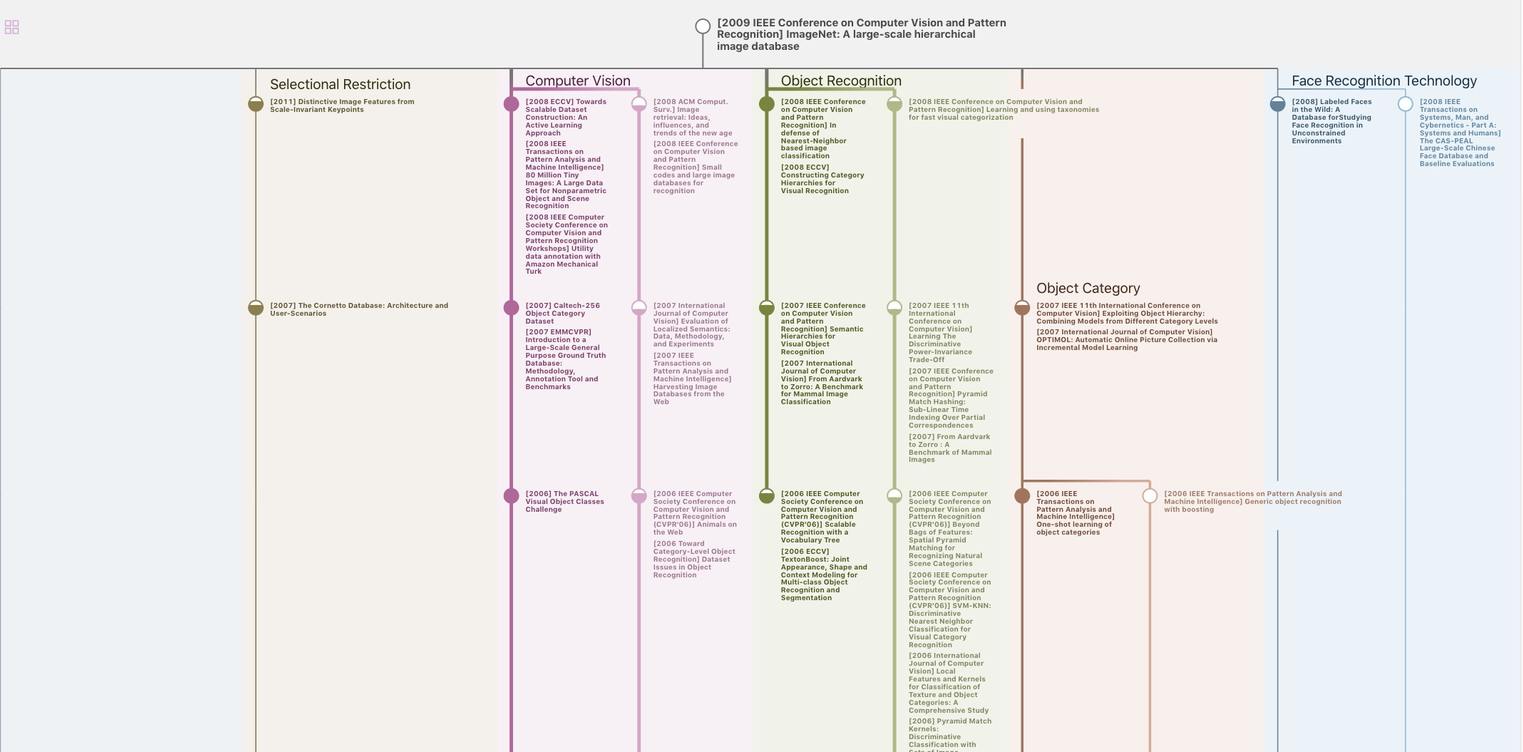
生成溯源树,研究论文发展脉络
Chat Paper
正在生成论文摘要