Over-the-Air Federated Learning with Enhanced Privacy
ICC 2023 - IEEE INTERNATIONAL CONFERENCE ON COMMUNICATIONS(2023)
摘要
Federated learning (FL) has emerged as a promising learning paradigm in which only local model parameters (gradients) are shared. Private user data never leaves the local devices thus preserving data privacy. However, recent research has shown that even when local data is never shared by a user, exchanging model parameters without protection can also leak private information. Moreover, in wireless systems, the frequent transmission of model parameters can cause tremendous bandwidth consumption and network congestion when the model is large. To address this problem, we propose a new FL framework with efficient over-the-air parameter aggregation and strong privacy protection of both user data and models. We achieve this by introducing pairwise cancellable random artificial noises (PCR-ANs) on end devices. As compared to existing over-the-air computation (AirComp) based FL schemes, our design provides stronger privacy protection. We analytically show the secrecy capacity and the convergence rate of the proposed wireless FL aggregation algorithm.
更多查看译文
关键词
Over-the-air computation (AirComp),wireless multiple-access channel,federated learning
AI 理解论文
溯源树
样例
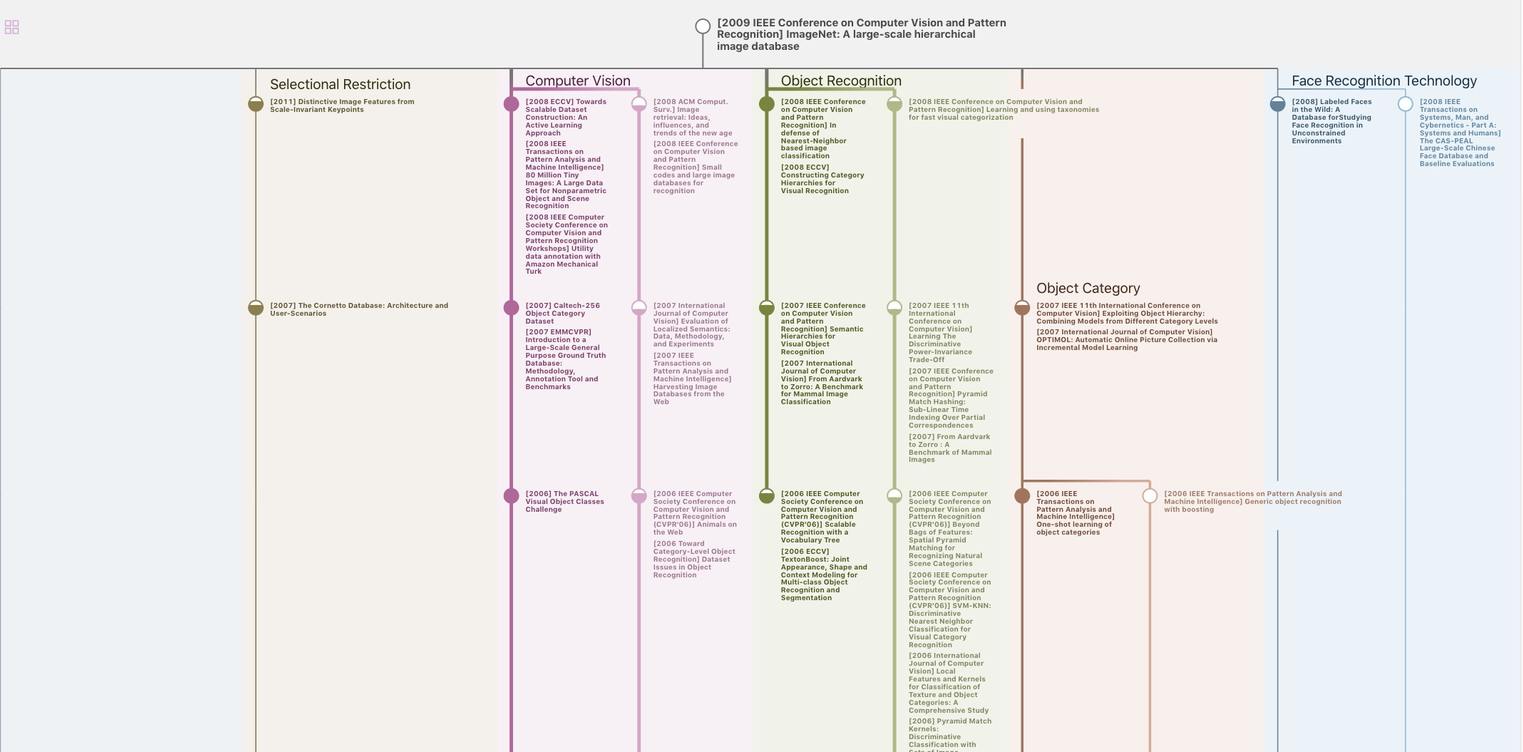
生成溯源树,研究论文发展脉络
Chat Paper
正在生成论文摘要