Feature Acquisition using Monte Carlo Tree Search
arXiv (Cornell University)(2022)
摘要
Feature acquisition algorithms address the problem of acquiring informative features while balancing the costs of acquisition to improve the learning performances of ML models. Previous approaches have focused on calculating the expected utility values of features to determine the acquisition sequences. Other approaches formulated the problem as a Markov Decision Process (MDP) and applied reinforcement learning based algorithms. In comparison to previous approaches, we focus on 1) formulating the feature acquisition problem as a MDP and applying Monte Carlo Tree Search, 2) calculating the intermediary rewards for each acquisition step based on model improvements and acquisition costs and 3) simultaneously optimizing model improvement and acquisition costs with multi-objective Monte Carlo Tree Search. With Proximal Policy Optimization and Deep Q-Network algorithms as benchmark, we show the effectiveness of our proposed approach with experimental study.
更多查看译文
关键词
Feature Acquisition,Monte Carlo Tree Search,Multi-objective Optimization
AI 理解论文
溯源树
样例
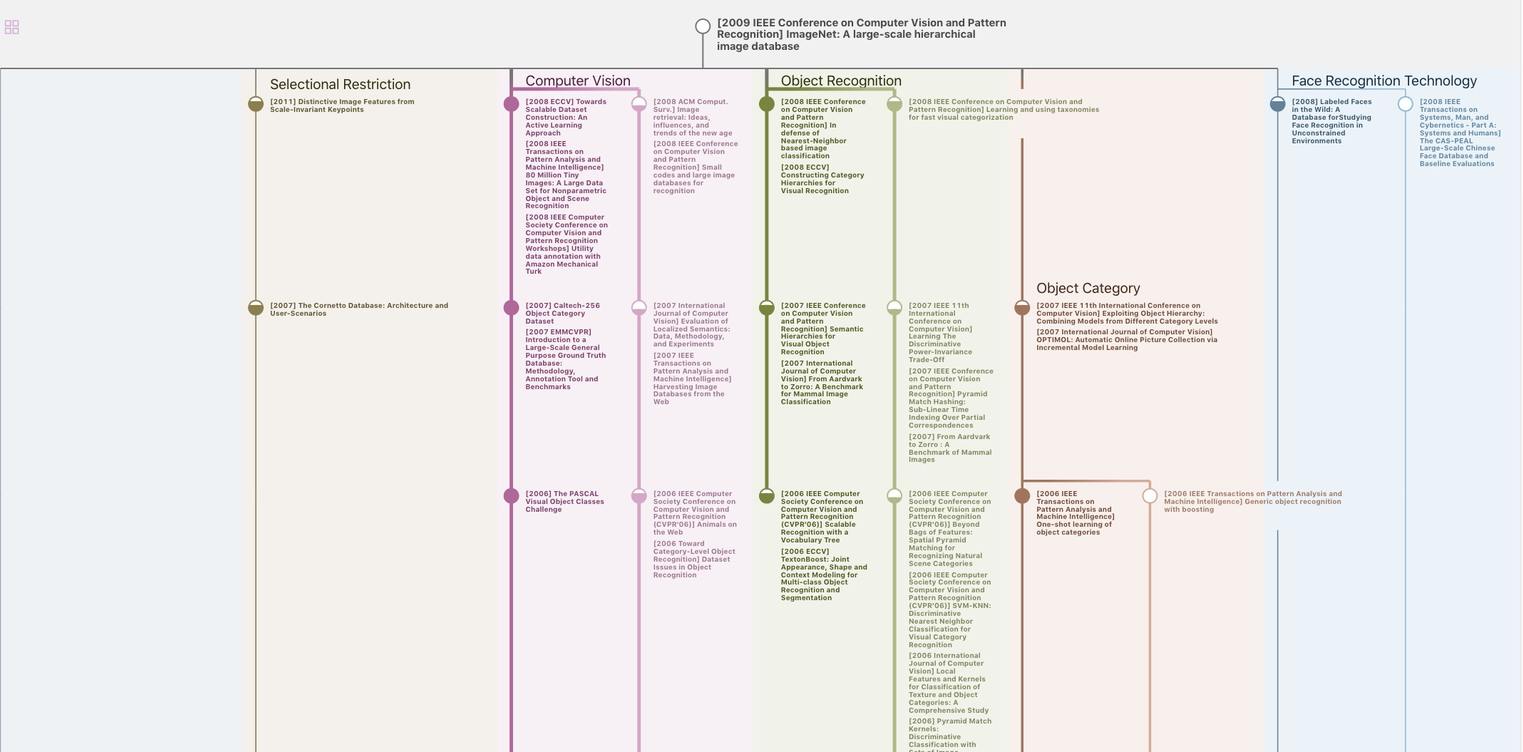
生成溯源树,研究论文发展脉络
Chat Paper
正在生成论文摘要