Contention alleviation in WiFi networks by using light-weight machine learning model
Computer Networks(2022)
摘要
The random access MAC (e.g., CSMA/CA) is known to be vulnerable to heavy collisions when the transmission contention level is high. Heavy collisions have typically been mitigated by adjusting the length of the waiting interval imposed before individual transmission attempts, called ’backoff time’. For example, there exist a large body of studies to enhance the efficiency of the WiFi backoff mechanism. In this paper, we propose a novel scheme that inserts a configurable non-random duration, called offset, before the beginning of the conventional CSMA/CA backoff. Since the existing backoff mechanism is untouched, the proposed scheme is compatible with all legacy and future WiFi standards. The size of offset is dynamically determined at run time by using a lightweight machine learning model. While machine learning models have been applied to the problem of contention alleviation in WiFi networks, the proposed scheme is unique in its flexibility and low overhead for model training and inferencing. Our scheme employs a simple lightweight DNN (Deep Neural Network) model which does not require a large amount of training data. The complexity of our machine learning model does not increase even if the number of the devices in the WiFi networks increases. Furthermore, the proposed scheme is executed in a distributed fashion, so that (near) real-time adaptation to dynamic change of traffic condition is possible at each end device that is not equipped with high computing resource (i.e., on-device DNN model execution).
更多查看译文
关键词
IEEE 802.11,Light-weight deep learning,Contention alleviation,Reinforcement learning
AI 理解论文
溯源树
样例
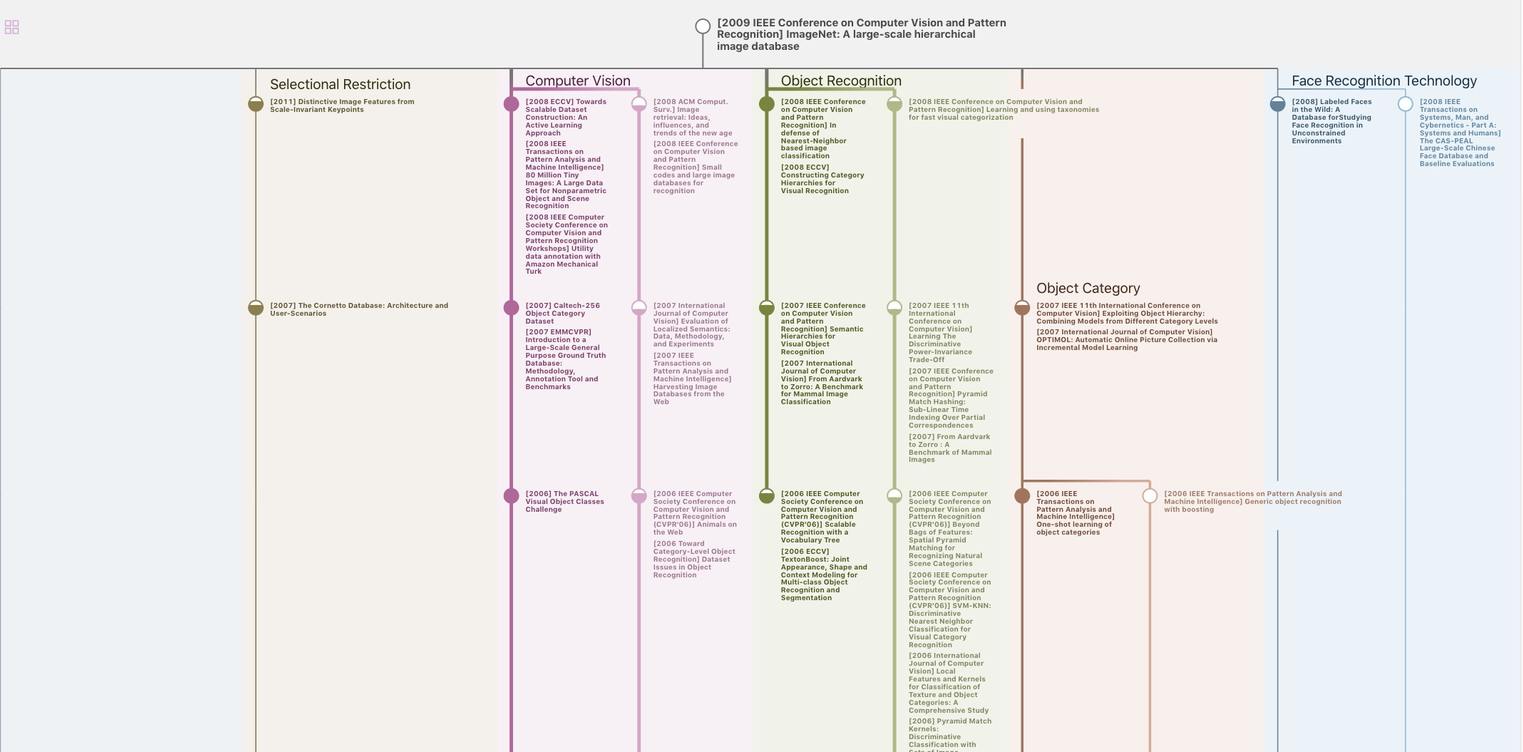
生成溯源树,研究论文发展脉络
Chat Paper
正在生成论文摘要