Model averaging for semiparametric varying coefficient quantile regression models
ANNALS OF THE INSTITUTE OF STATISTICAL MATHEMATICS(2022)
摘要
In this study, we propose a model averaging approach to estimating the conditional quantiles based on a set of semiparametric varying coefficient models. Different from existing literature on the subject, we consider a particular form for all candidates, where there is only one varying coefficient in each sub-model, and all the candidates under investigation may be misspecified. We propose a weight choice criterion based on a leave-more-out cross-validation objective function. Moreover, the resulting averaging estimator is more robust against model misspecification due to the weighted coefficients that adjust the relative importance of the varying and constant coefficients for the same predictors. We prove out statistical properties for each sub-model and asymptotic optimality of the weight selection method. Simulation studies show that the proposed procedure has satisfactory prediction accuracy. An analysis of a skin cutaneous melanoma data further supports the merits of the proposed approach.
更多查看译文
关键词
Model averaging,Quantile regression,Local linear fitting,Semiparametric model,Varying coefficient
AI 理解论文
溯源树
样例
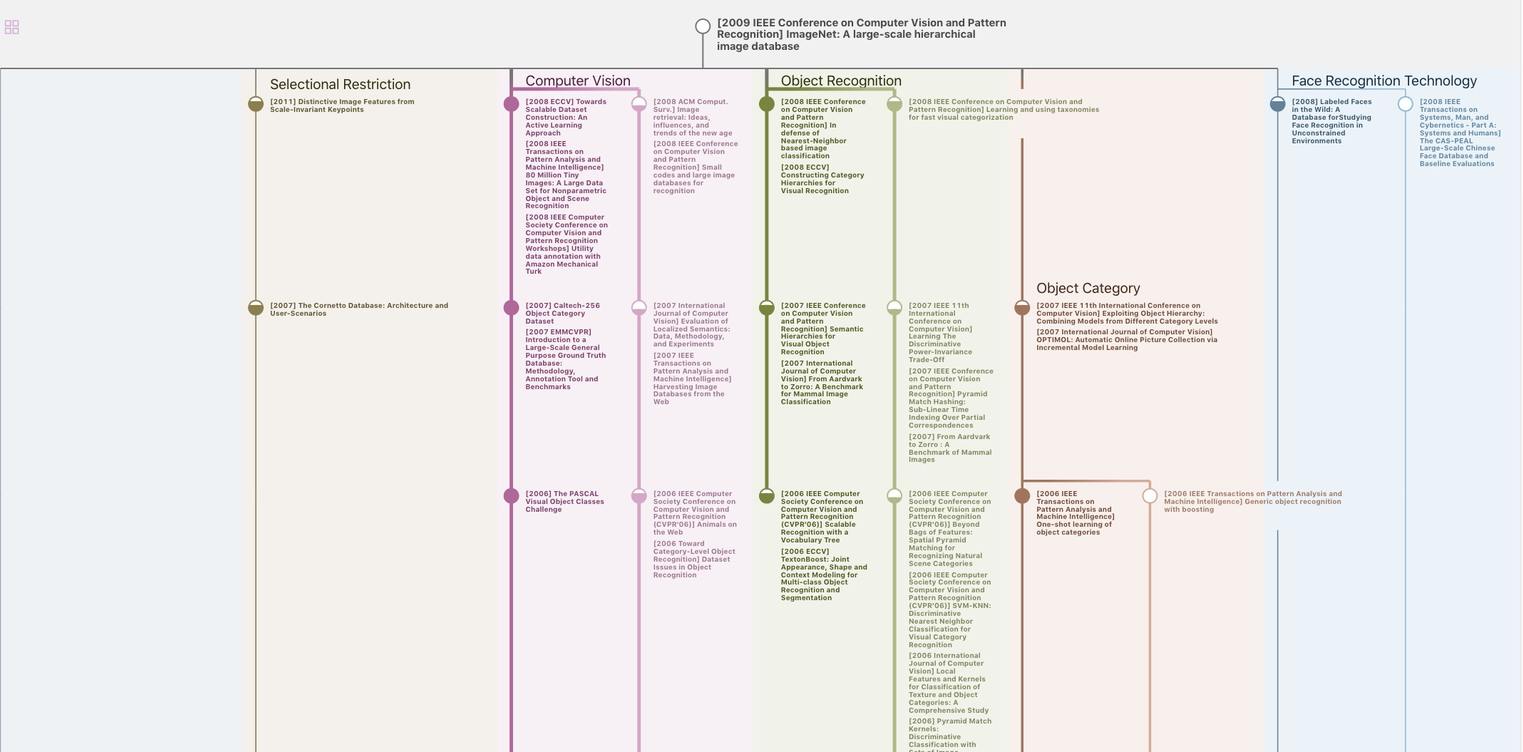
生成溯源树,研究论文发展脉络
Chat Paper
正在生成论文摘要