LasUIE: Unifying Information Extraction with Latent Adaptive Structure-aware Generative Language Model
NeurIPS 2022(2022)
摘要
Universally modeling all typical information extraction tasks (UIE) with one generative language model (GLM) has revealed great potential by the latest study, where various IE predictions are unified into a linearized hierarchical expression under a GLM. Syntactic structure information, a type of effective feature which has been extensively utilized in IE community, should also be beneficial to UIE. In this work, we propose a novel structure-aware GLM, fully unleashing the power of syntactic knowledge for UIE. A heterogeneous structure inductor is explored to unsupervisedly induce rich heterogeneous structural representations by post-training an existing GLM. In particular, a structural broadcaster is devised to compact various latent trees into explicit high-order forests, helping to guide a better generation during decoding. We finally introduce a task-oriented structure fine-tuning mechanism, further adjusting the learned structures to most coincide with the end-task's need. Over 12 IE benchmarks across 7 tasks our system shows significant improvements over the baseline UIE system. Further in-depth analyses show that our GLM learns rich task-adaptive structural bias that greatly resolves the UIE crux, the long-range dependence issue and boundary identifying.
更多查看译文
关键词
Information extraction,Syntactic structure,Language model,Natural language processing
AI 理解论文
溯源树
样例
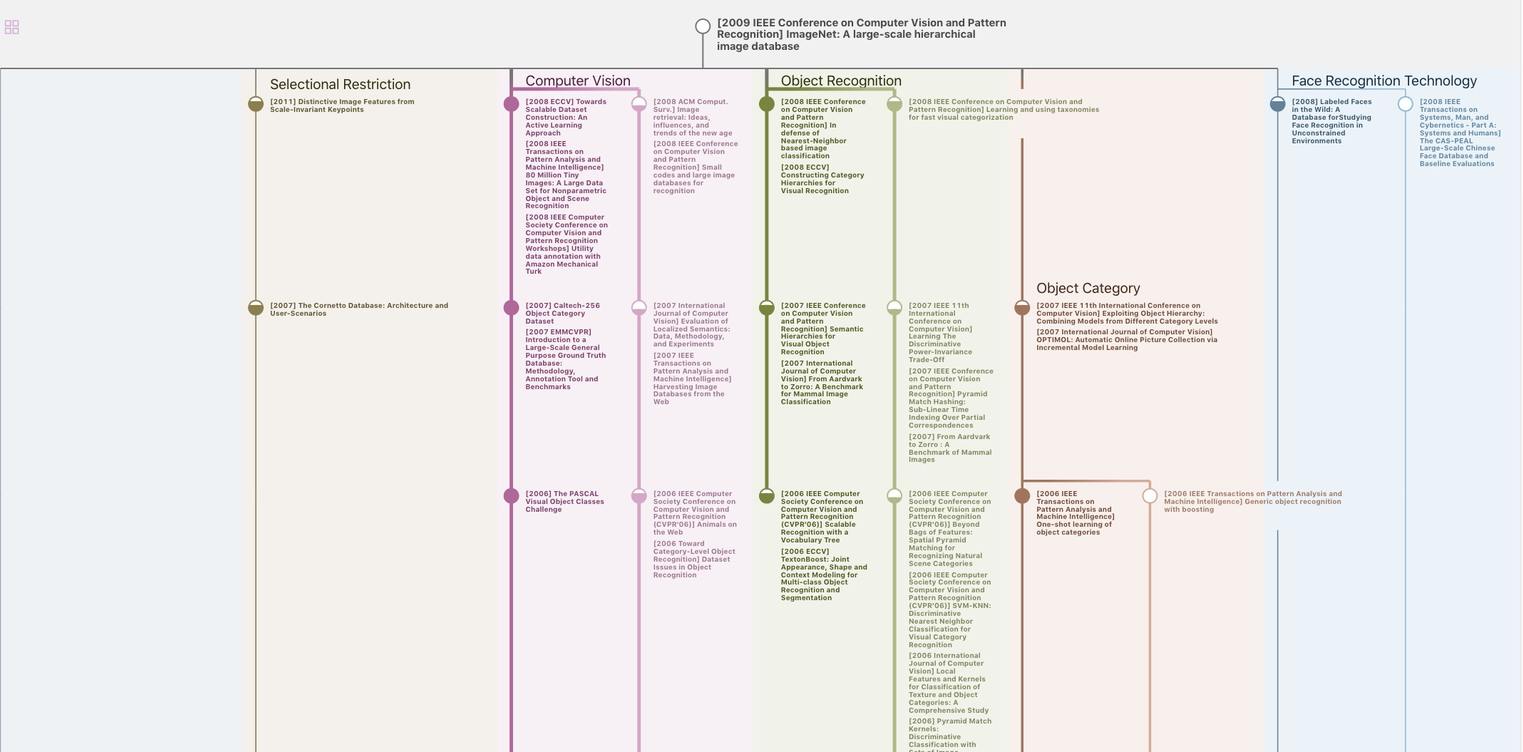
生成溯源树,研究论文发展脉络
Chat Paper
正在生成论文摘要