Learning Manifold Dimensions with Conditional Variational Autoencoders
NeurIPS 2022(2022)
摘要
Although the variational autoencoder (VAE) and its conditional extension (CVAE) are capable of state-of-the-art results across multiple domains, their precise behavior is still not fully understood, particularly in the context of data (like images) that lie on or near a low-dimensional manifold. For example, while prior work has suggested that the globally optimal VAE solution can learn the correct manifold dimension, a necessary (but not sufficient) condition for producing samples from the true data distribution, this has never been rigorously proven. Moreover, it remains unclear how such considerations would change when various types of conditioning variables are introduced, or when the data support is extended to a union of manifolds (e.g., as is likely the case for MNIST digits and related). In this work, we address these points by first proving that VAE global minima are indeed capable of recovering the correct manifold dimension. We then extend this result to more general CVAEs, demonstrating practical scenarios whereby the conditioning variables allow the model to adaptively learn manifolds of varying dimension across samples. Our analyses, which have practical implications for various CVAE design choices, are also supported by numerical results on both synthetic and real-world datasets.
更多查看译文
关键词
Conditional Variational Autoencoder,Deep Generative Model
AI 理解论文
溯源树
样例
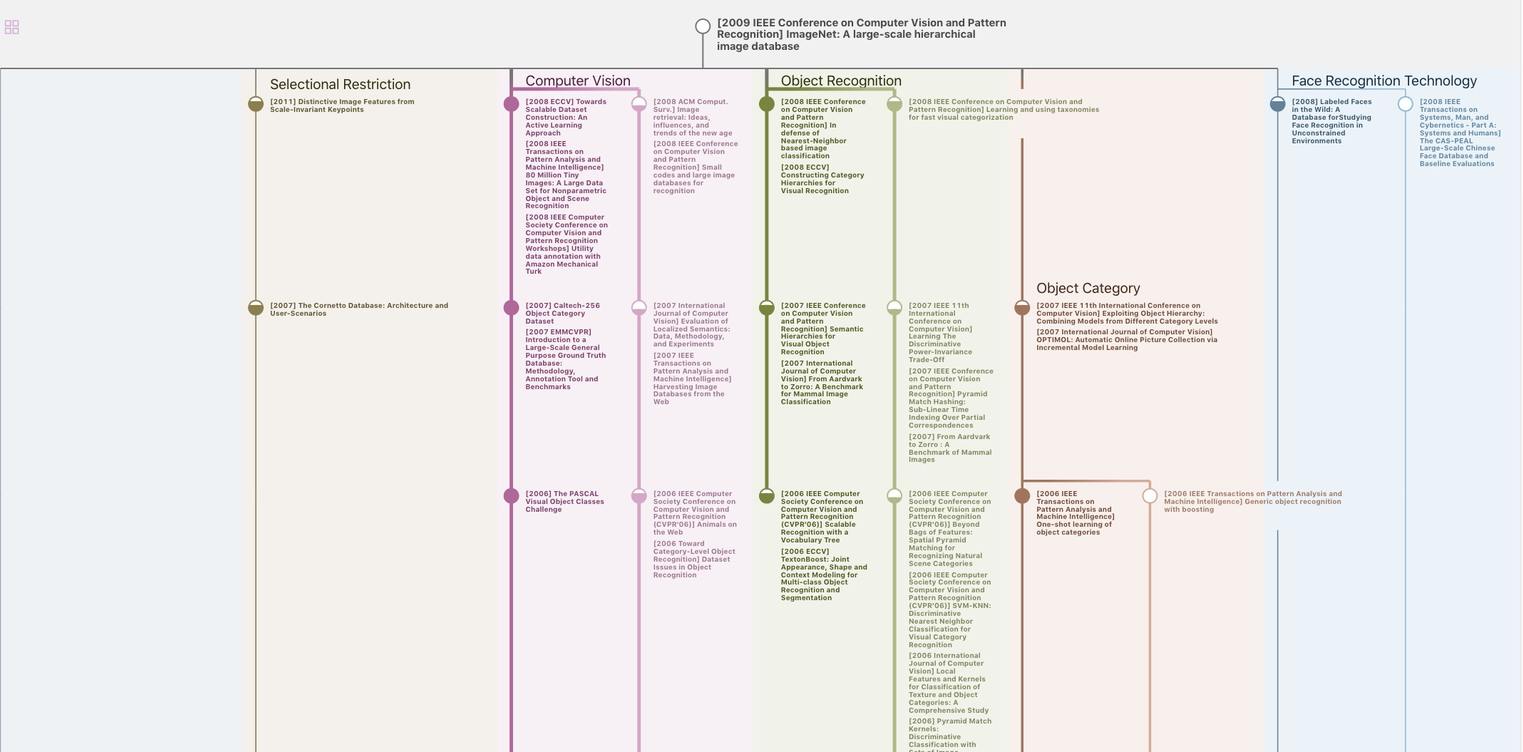
生成溯源树,研究论文发展脉络
Chat Paper
正在生成论文摘要