Alleviating ``Posterior Collapse'' in Deep Topic Models via Policy Gradient
NeurIPS 2022(2022)
摘要
Deep topic models have been proven as a promising way to extract hierarchical latent representations from documents represented as high-dimensional bag-of-words vectors.
However, the representation capability of existing deep topic models is still limited by the phenomenon of "posterior collapse", which has been widely criticized in deep generative models, resulting in the higher-level latent representations exhibiting similar or meaningless patterns.
To this end, in this paper, we first develop a novel deep-coupling generative process for existing deep topic models, which incorporates skip connections into the generation of documents, enforcing strong links between the document and its multi-layer latent representations.
After that, utilizing data augmentation techniques, we reformulate the deep-coupling generative process as a Markov decision process and develop a corresponding Policy Gradient (PG) based training algorithm, which can further alleviate the information reduction at higher layers.
Extensive experiments demonstrate that our developed methods can effectively alleviate "posterior collapse" in deep topic models, contributing to providing higher-quality latent document representations.
更多查看译文
关键词
Deep Topic Models,Posterior Collapse,Reinforcement Learning
AI 理解论文
溯源树
样例
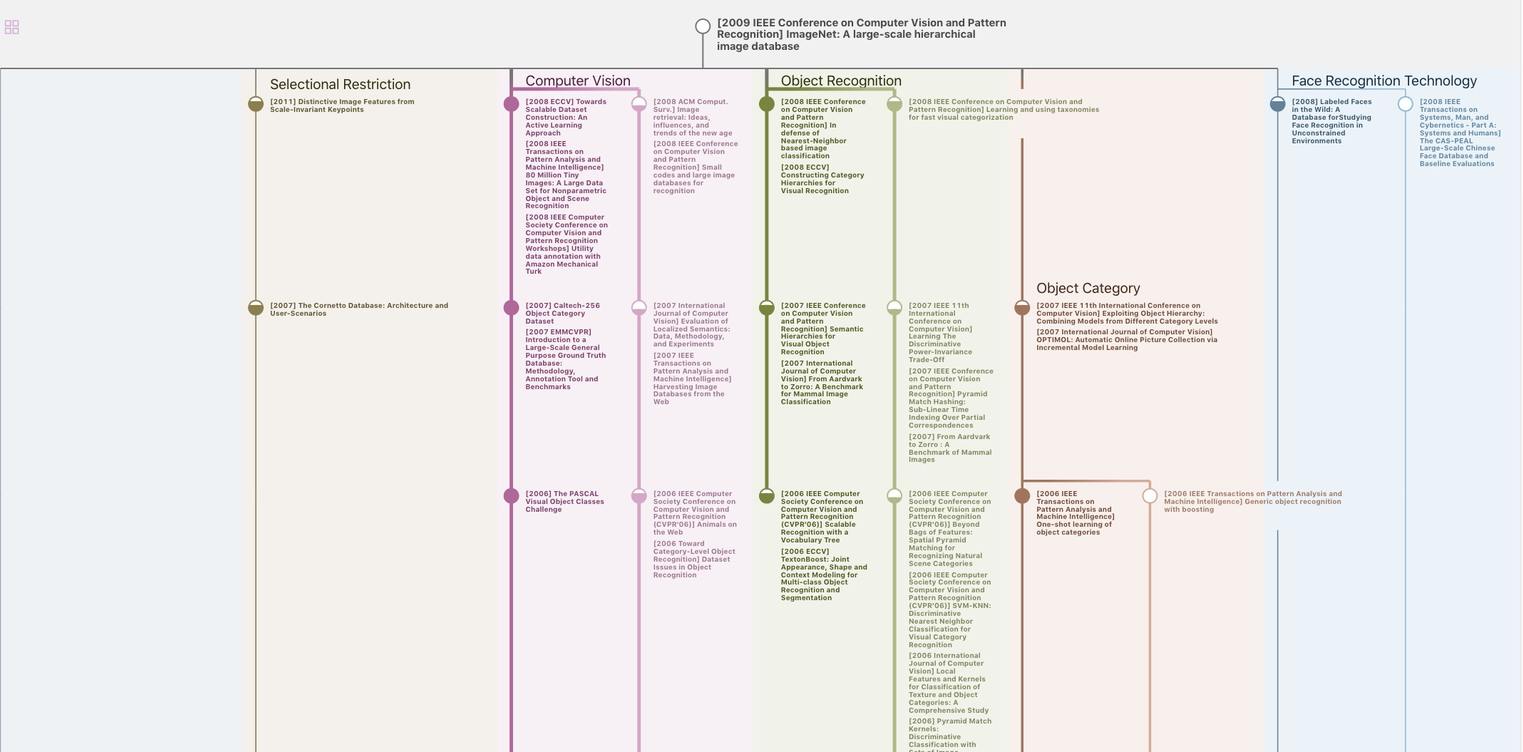
生成溯源树,研究论文发展脉络
Chat Paper
正在生成论文摘要