PlasticityNet: Learning to Simulate Metal, Sand, and Snow for Optimization Time Integration
NeurIPS 2022(2022)
摘要
In this paper, we propose a neural network-based approach for learning to represent the behavior of plastic solid materials ranging from rubber and metal to sand and snow. Unlike elastic forces such as spring forces, these plastic forces do not result from the positional gradient of any potential energy, imposing great challenges on the stability and flexibility of their simulation. Our method effectively resolves this issue by learning a generalizable plastic energy whose derivative closely matches the analytical behavior of plastic forces. Our method, for the first time, enables the simulation of a wide range of arbitrary elasticity-plasticity combinations using time step-independent, unconditionally stable optimization-based time integrators. We demonstrate the efficacy of our method by learning and producing challenging 2D and 3D effects of metal, sand, and snow with complex dynamics.
更多查看译文
关键词
neural network,plasticity,optimization-based time integrator
AI 理解论文
溯源树
样例
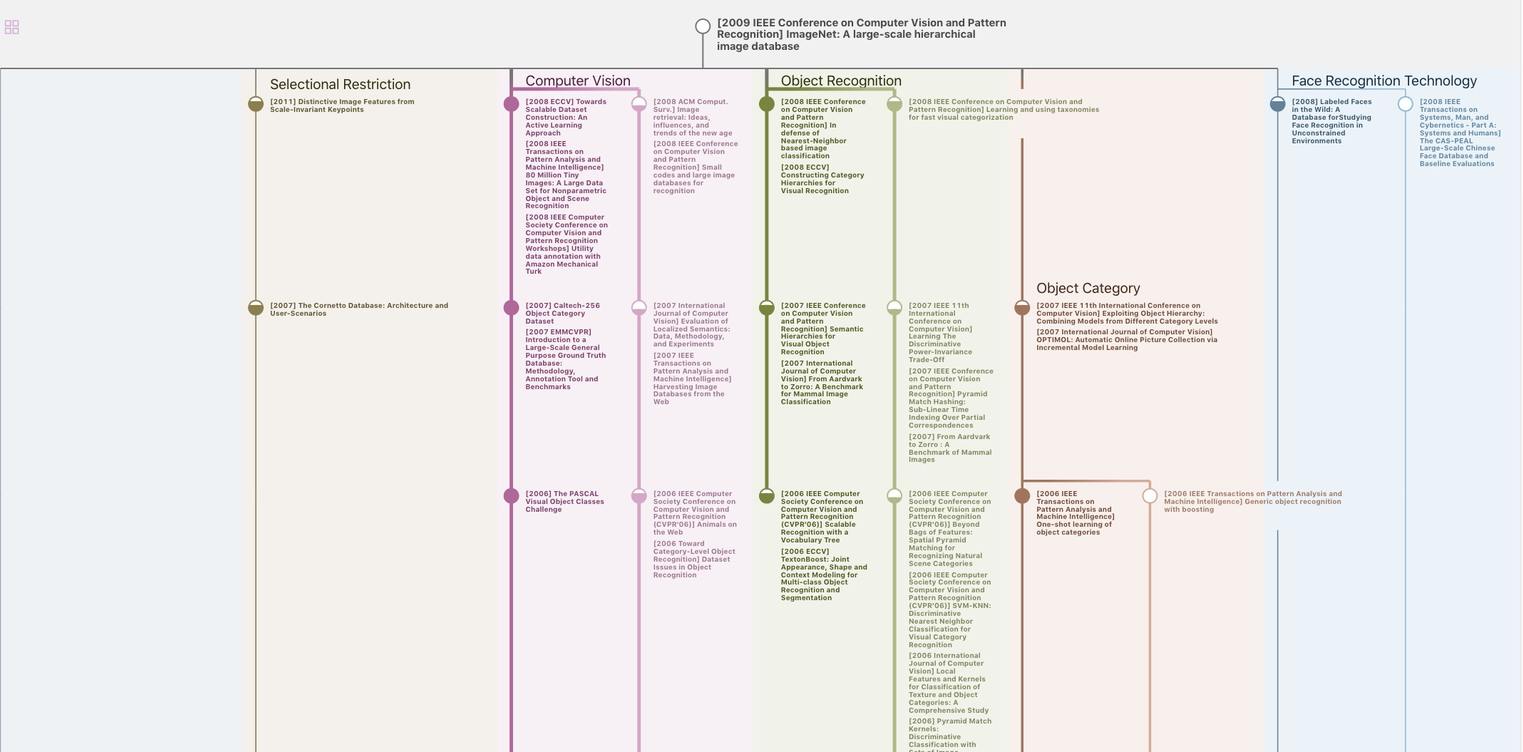
生成溯源树,研究论文发展脉络
Chat Paper
正在生成论文摘要