Balanced Split: A new train-test data splitting strategy for imbalanced datasets
arxiv(2022)
摘要
Classification data sets with skewed class proportions are called imbalanced. Class imbalance is a problem since most machine learning classification algorithms are built with an assumption of equal representation of all classes in the training dataset. Therefore to counter the class imbalance problem, many algorithm-level and data-level approaches have been developed. These mainly include ensemble learning and data augmentation techniques. This paper shows a new way to counter the class imbalance problem through a new data-splitting strategy called balanced split. Data splitting can play an important role in correctly classifying imbalanced datasets. We show that the commonly used data-splitting strategies have some disadvantages, and our proposed balanced split has solved those problems.
更多查看译文
关键词
split,splitting,datasets,train-test
AI 理解论文
溯源树
样例
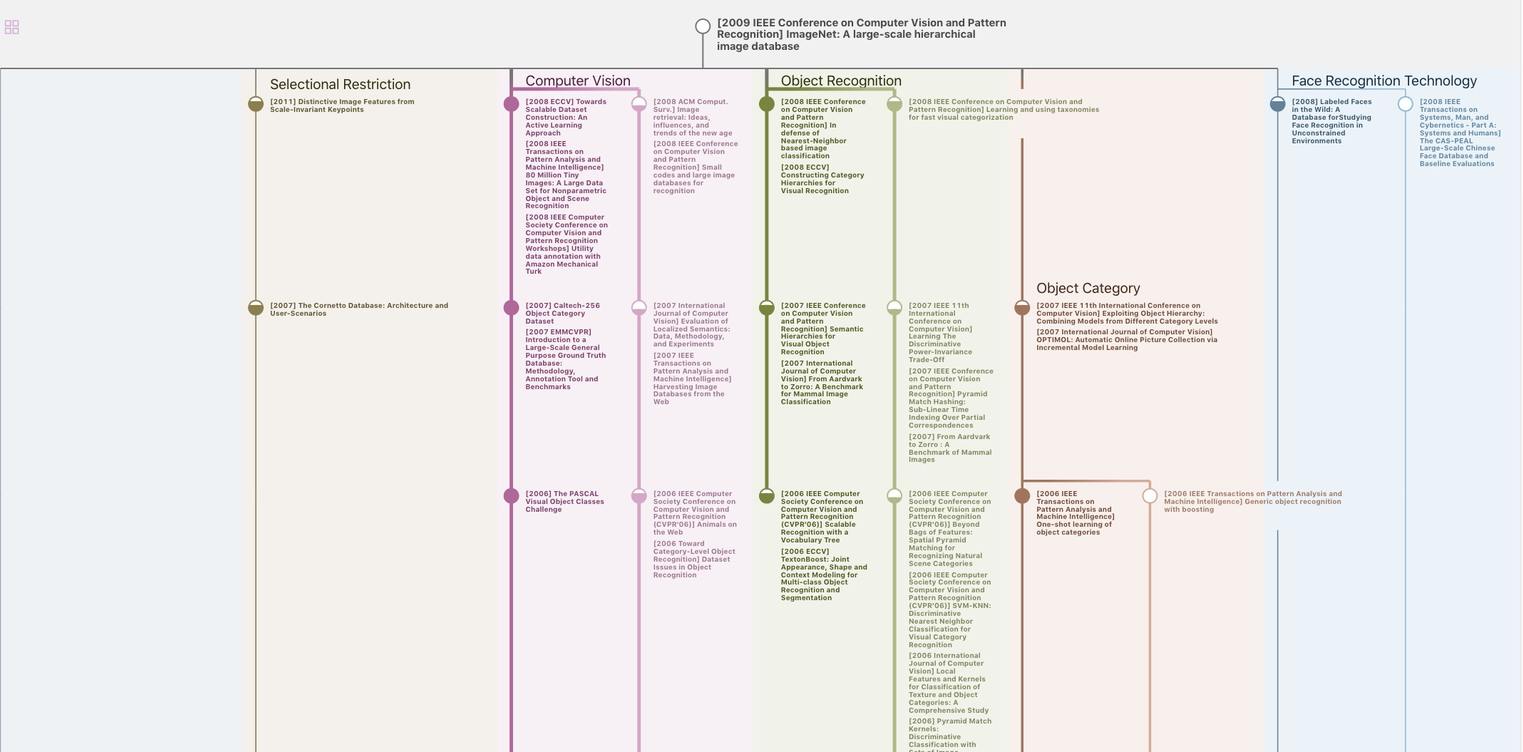
生成溯源树,研究论文发展脉络
Chat Paper
正在生成论文摘要