MAViC: Multimodal Active Learning for Video Captioning
arXiv (Cornell University)(2022)
摘要
A large number of annotated video-caption pairs are required for training video captioning models, resulting in high annotation costs. Active learning can be instrumental in reducing these annotation requirements. However, active learning for video captioning is challenging because multiple semantically similar captions are valid for a video, resulting in high entropy outputs even for less-informative samples. Moreover, video captioning algorithms are multimodal in nature with a visual encoder and language decoder. Further, the sequential and combinatorial nature of the output makes the problem even more challenging. In this paper, we introduce MAViC which leverages our proposed Multimodal Semantics Aware Sequential Entropy (M-SASE) based acquisition function to address the challenges of active learning approaches for video captioning. Our approach integrates semantic similarity and uncertainty of both visual and language dimensions in the acquisition function. Our detailed experiments empirically demonstrate the efficacy of M-SASE for active learning for video captioning and improve on the baselines by a large margin.
更多查看译文
关键词
multimodal active learning,video captioning
AI 理解论文
溯源树
样例
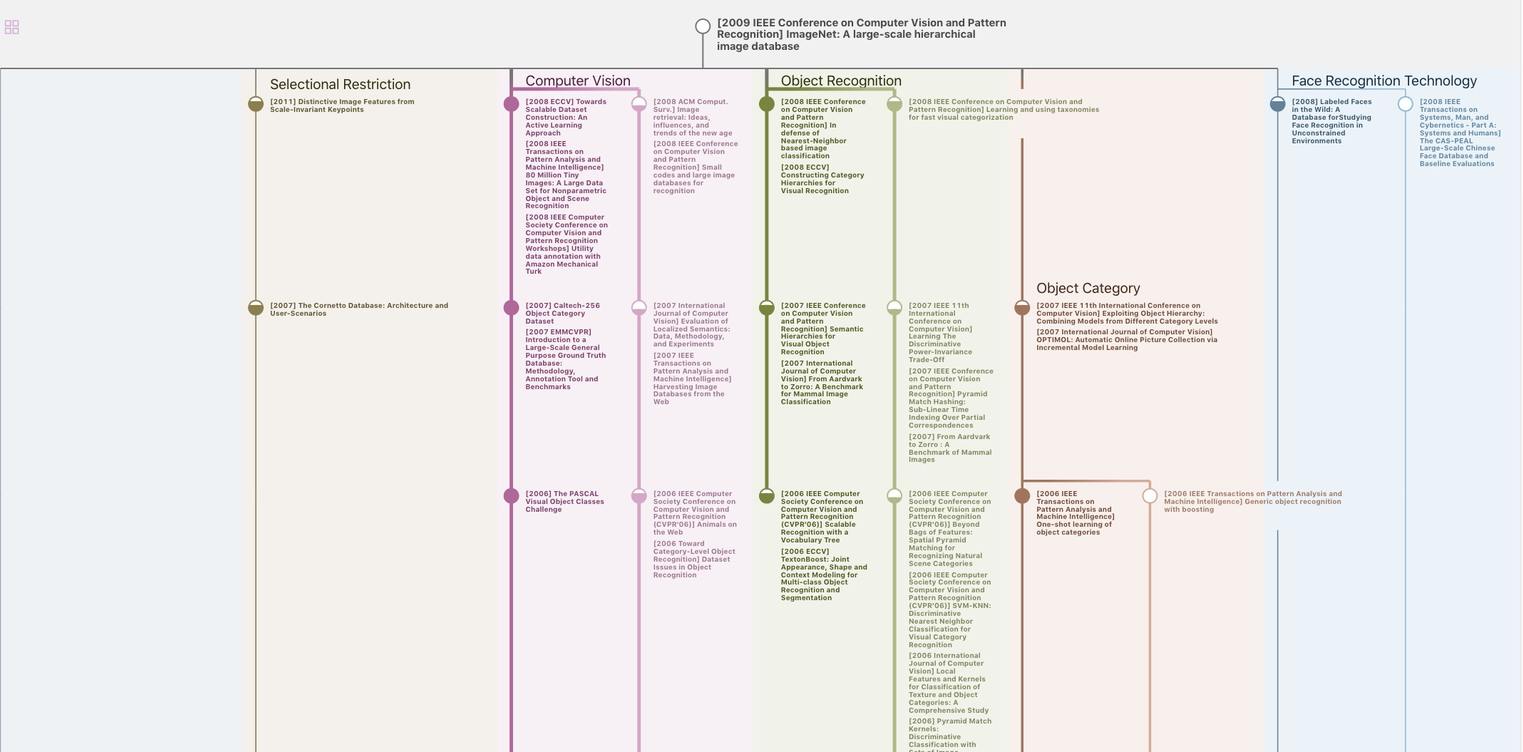
生成溯源树,研究论文发展脉络
Chat Paper
正在生成论文摘要