TruFor: Leveraging All-Round Clues for Trustworthy Image Forgery Detection and Localization
CVPR 2023(2023)
摘要
In this paper we present TruFor, a forensic framework that can be applied to a large variety of image manipulation methods, from classic cheapfakes to more recent manipulations based on deep learning. We rely on the extraction of both high-level and low-level traces through a transformer-based fusion architecture that combines the RGB image and a learned noise-sensitive fingerprint. The latter learns to embed the artifacts related to the camera internal and external processing by training only on real data in a self-supervised manner. Forgeries are detected as deviations from the expected regular pattern that characterizes each pristine image. Looking for anomalies makes the approach able to robustly detect a variety of local manipulations, ensuring generalization. In addition to a pixel-level localization map and a whole-image integrity score, our approach outputs a reliability map that highlights areas where localization predictions may be error-prone. This is particularly important in forensic applications in order to reduce false alarms and allow for a large scale analysis. Extensive experiments on several datasets show that our method is able to reliably detect and localize both cheapfakes and deepfakes manipulations outperforming state-of-the-art works. Code is publicly available at https://grip-unina.github.io/TruFor/.
更多查看译文
关键词
trustworthy image forgery
AI 理解论文
溯源树
样例
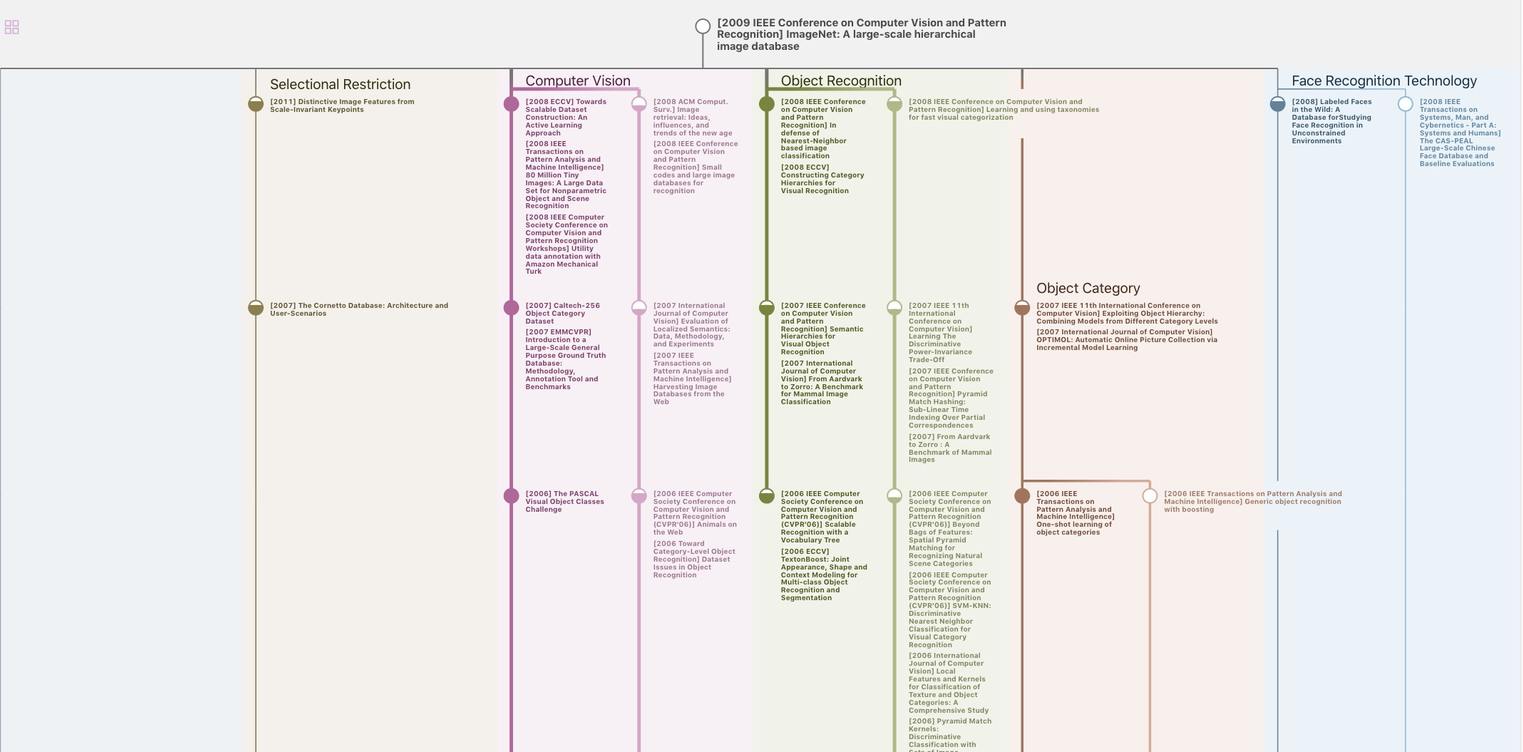
生成溯源树,研究论文发展脉络
Chat Paper
正在生成论文摘要