SPT: Semi-Parametric Prompt Tuning for Multitask Prompted Learning
arxiv(2022)
摘要
Pre-trained large language models can efficiently interpolate human-written prompts in a natural way. Multitask prompted learning can help generalization through a diverse set of tasks at once, thus enhancing the potential for more effective downstream fine-tuning. To perform efficient multitask-inference in the same batch, parameter-efficient fine-tuning methods such as prompt tuning have been proposed. However, the existing prompt tuning methods may lack generalization. We propose SPT, a semi-parametric prompt tuning method for multitask prompted learning. The novel component of SPT is a memory bank from where memory prompts are retrieved based on discrete prompts. Extensive experiments, such as (i) fine-tuning a full language model with SPT on 31 different tasks from 8 different domains and evaluating zero-shot generalization on 9 heldout datasets under 5 NLP task categories and (ii) pretraining SPT on the GLUE datasets and evaluating fine-tuning on the SuperGLUE datasets, demonstrate effectiveness of SPT.
更多查看译文
关键词
learning,semi-parametric
AI 理解论文
溯源树
样例
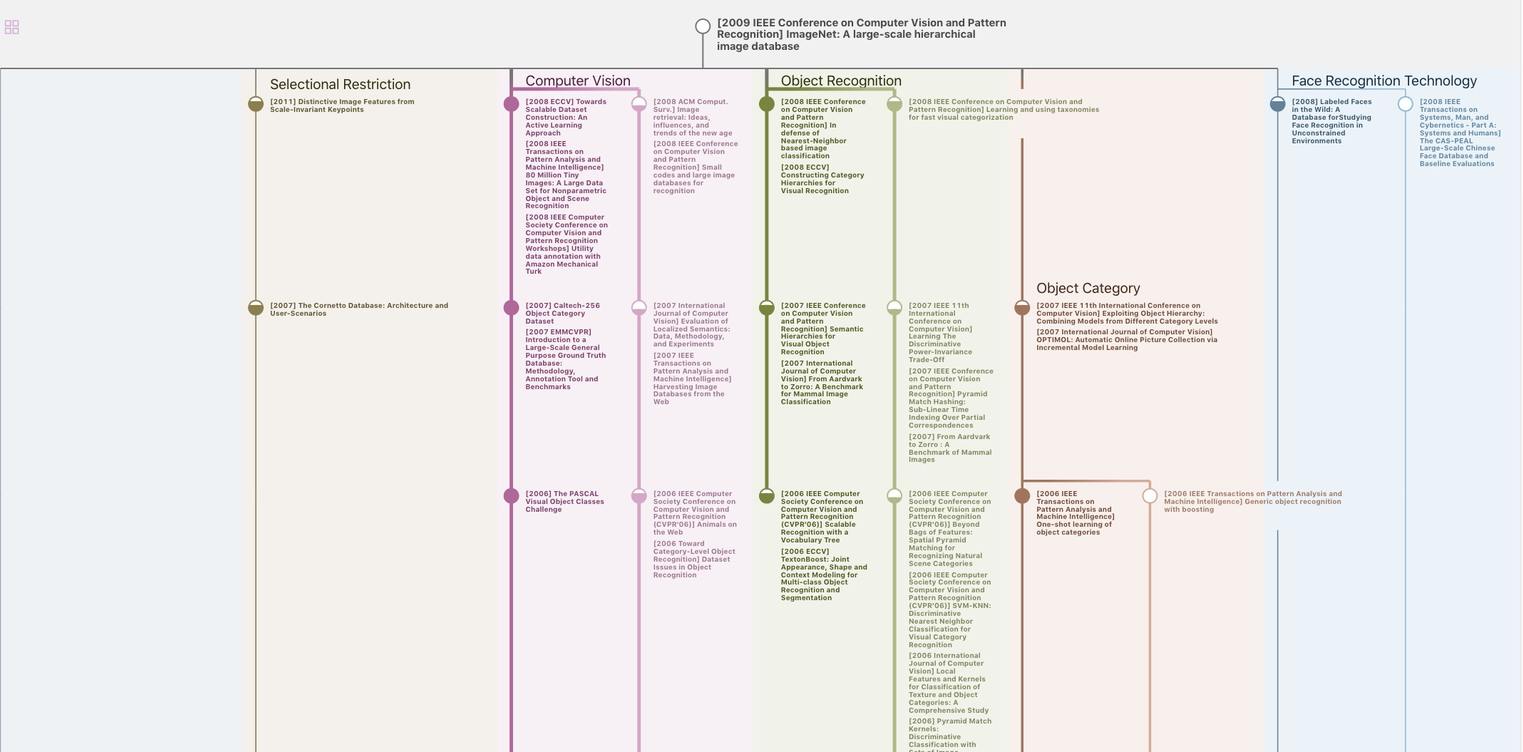
生成溯源树,研究论文发展脉络
Chat Paper
正在生成论文摘要