Local Differential Privacy Image Generation Using Flow-Based Deep Generative Models
APPLIED SCIENCES-BASEL(2023)
摘要
Diagnostic radiologists need artificial intelligence (AI) for medical imaging, but access to medical images required for training in AI has become increasingly restrictive. To release and use medical images, we need an algorithm that can simultaneously protect privacy and preserve pathologies in medical images. To address this, we introduce DP-GLOW, a hybrid that combines the local differential privacy (LDP) algorithm with GLOW, one of the flow-based deep generative models. By applying a GLOW model, we disentangle the pixelwise correlation of images, which makes it difficult to protect privacy with straightforward LDP algorithms for images. Specifically, we map images to the latent vector of the GLOW model, where each element follows an independent normal distribution. We then apply the Laplace mechanism to this latent vector to achieve epsilon-LDP, which is one of the LDP algorithms. Moreover, we applied DP-GLOW to chest X-ray images to generate LDP images while preserving pathologies. The epsilon-LDP-processed chest X-ray images obtained with DP-GLOW indicate that we have obtained a powerful tool for releasing and using medical images for training AI.
更多查看译文
关键词
differential privacy,deep generative models,medical images,privacy protection,database,image obfuscation
AI 理解论文
溯源树
样例
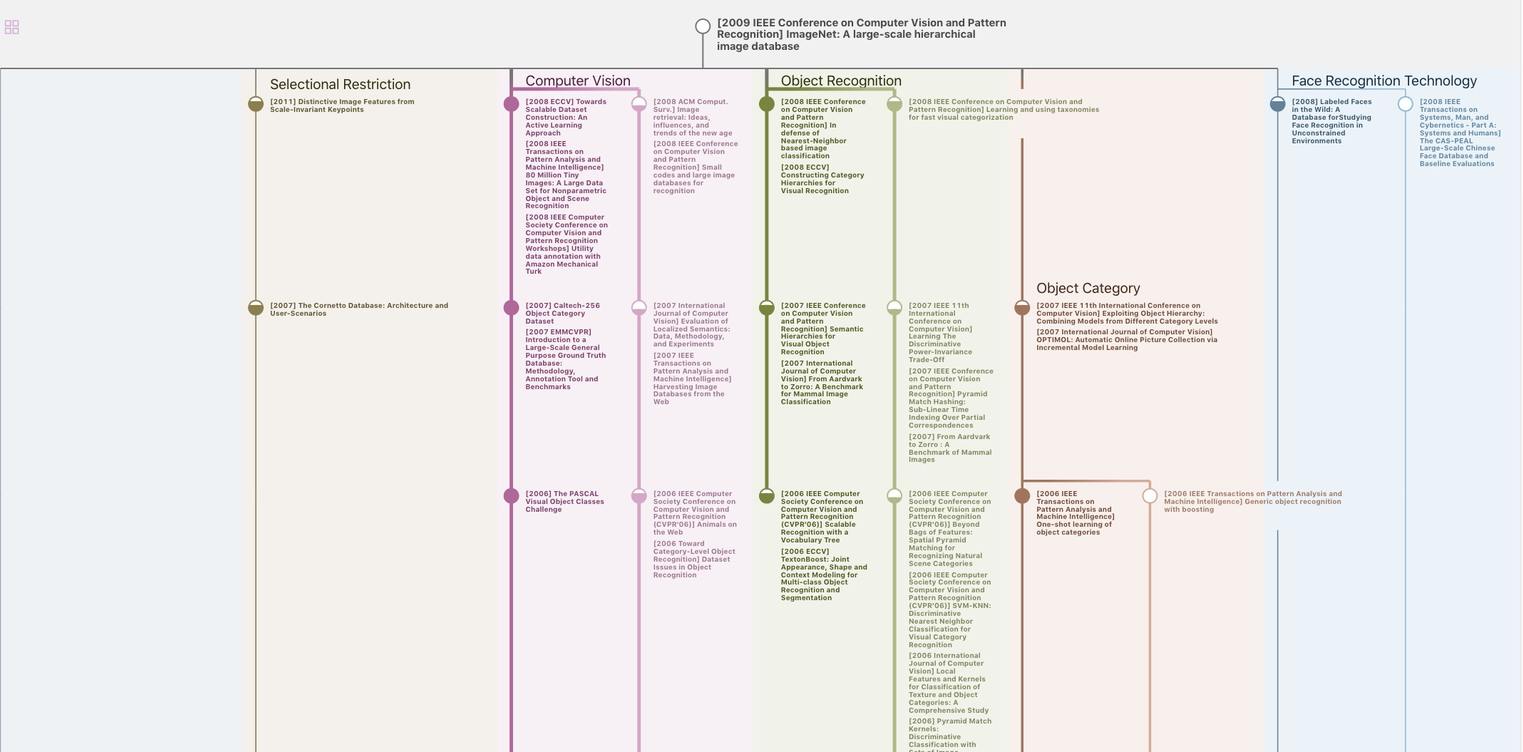
生成溯源树,研究论文发展脉络
Chat Paper
正在生成论文摘要