Contraction-based model predictive control for stochastic nonlinear discrete-time systems with time-varying delays via multi-dimensional Taylor network
Journal of the Franklin Institute(2023)
摘要
For stochastic nonlinear systems with time-varying delays, the existing robust control approaches are unnecessarily conservative in most practical scenarios. Within this context, a mathematically rigorous and computationally tractable tube-based model predictive control scheme is proposed in the framework of contraction theory. A contraction metric is systematically constructed via convex optimization by forming a differential LyapunovKrasovskii function on tangent space. It guarantees the perturbed actual solution trajectories to be contained within a robust positive invariant tube centered along the reference trajectories and results in an explicit exponential bound on the deviation. The application scenarios of the control contraction metric controller are extended from constant delay systems into time-varying delay systems thereby. Compared with the existing robust mechanism for time-delay systems based on min-max optimization formulation with a linear feedback controller, the proposed scheme greatly reduces the design conservativeness and yields a larger region of attraction. A sparse multi-dimensional Taylor network (MTN) is designed to parameterize the family of the geodesic. Compared to conventional NNs and MTN surrogates, sparse MTN features a more concise topology that enhances its computational efficiency conspicuously. Results of the numerical simulations verify the effectiveness of the proposed method.
更多查看译文
关键词
predictive control,delays,stochastic,contraction-based,discrete-time,time-varying,multi-dimensional
AI 理解论文
溯源树
样例
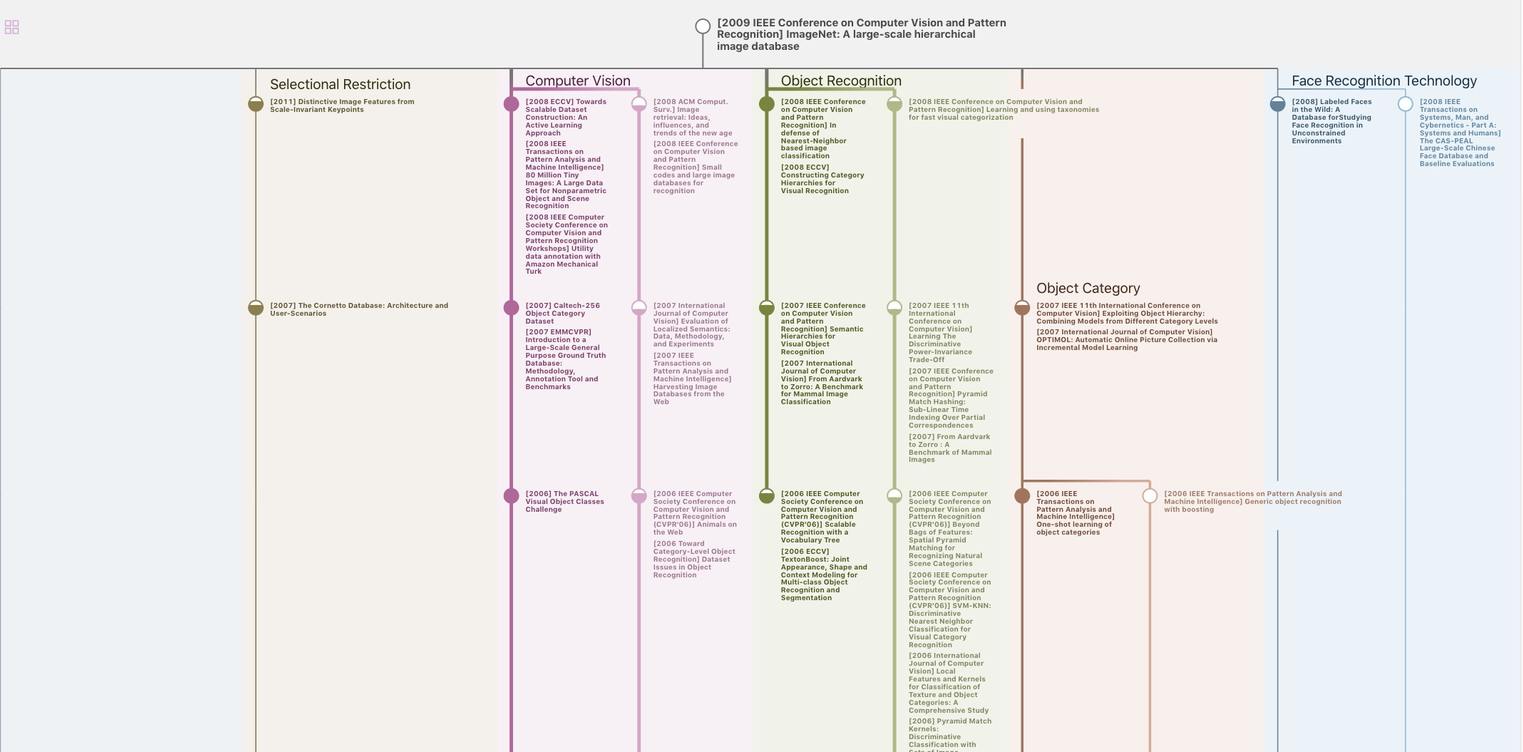
生成溯源树,研究论文发展脉络
Chat Paper
正在生成论文摘要