Data independent warmup scheme for non-IID federated learning
Information Sciences(2023)
摘要
Machine Learning’s influence increases daily, and its application prevails in different, critical, and life-changing fields. However, collecting the needed data is challenging due to the increasing concerns about clients’ privacy. In this context, federated learning addresses privacy issues by adopting an on-device model training strategy while communicating only the model parameters rather than raw data. Such an approach allows for preserving users’ information and shielding them from harm following malicious or suspicious parties’ dissemination of their private information. Nevertheless, the distributed nature of federated learning makes it vulnerable to the weight divergence problem caused by the Non-IID (Non-Independent And Identically Distributed) clients. As a result, we can observe a substantial accuracy reduction and convergence time increase. In this paper, we propose a scheme for federated learning addressing the Non-IID clients while also handling the dynamic evolution of the learning context without sacrificing the clients’ privacy. Our scheme takes advantage of the client’s weights to select a compatible subset with the minimum weight divergence to aggregate the initial global model. Our experiments show that the proposed method can boost the federated learning performance while reducing the convergence time.
更多查看译文
关键词
Federated learning,Non-IID,Genetic algorithm,Machine learning,Distributed learning
AI 理解论文
溯源树
样例
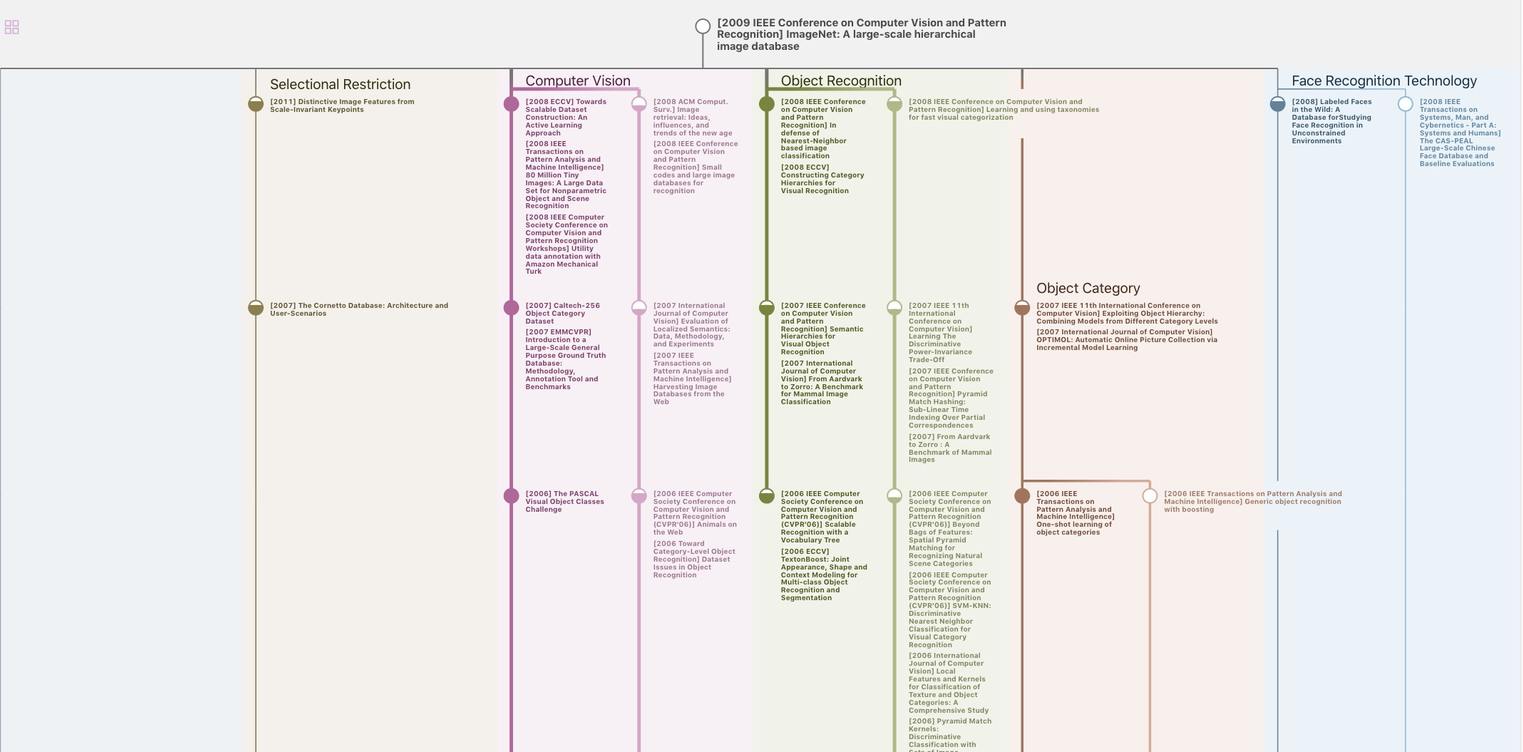
生成溯源树,研究论文发展脉络
Chat Paper
正在生成论文摘要