Difference embedding for recommender systems
Data Mining and Knowledge Discovery(2022)
摘要
This paper proposes a novel and straightforward pointwise training strategy, namely difference embedding (DifE), for recommender systems that capture the personalized information retained in pairwise preference differences while simply using effective and efficient pointwise training. Specifically, a function was designed to capture and emphasize pairwise preference differences. Then, a novel projection was used to construct a new space in which pairwise information is preserved, and the newly proposed pointwise loss function is sufficient to learn a better embedding. To verify the superiority and generality of the proposed strategy, we integrate the proposed loss function with four state-of-the-art recommenders and obtain four corresponding optimized models namely MF-DifE, NeuMF-DifE, GCN-DifE, and SGL-DifE. Comprehensive and comparative experiment results on three public datasets show that these optimized models achieve significant improvement compared to their corresponding baselines and outperform various recent recommendation methods, which indicates the excellence and generality of the proposed loss function.
更多查看译文
关键词
Machine learning,Recommender systems,Graph convolutional networks,Embedding
AI 理解论文
溯源树
样例
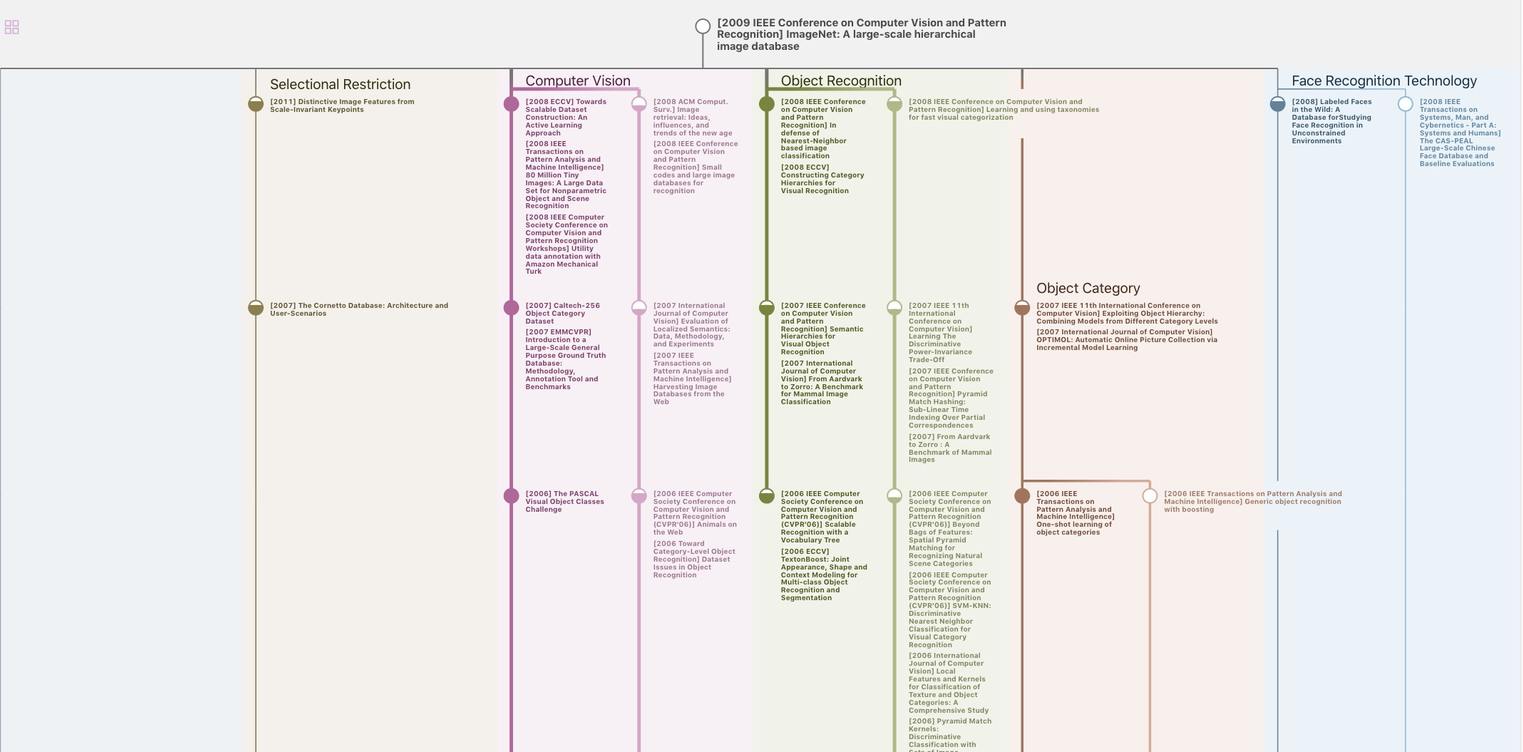
生成溯源树,研究论文发展脉络
Chat Paper
正在生成论文摘要