Normalized Contrastive Learning for Text-Video Retrieval
emnlp 2022(2022)
摘要
Cross-modal contrastive learning has led the recent advances in multimodal retrieval with its simplicity and effectiveness. In this work, however, we reveal that cross-modal contrastive learning suffers from incorrect normalization of the sum retrieval probabilities of each text or video instance. Specifically, we show that many test instances are either over- or under-represented during retrieval, significantly hurting the retrieval performance. To address this problem, we propose Normalized Contrastive Learning (NCL) which utilizes the Sinkhorn-Knopp algorithm to compute the instance-wise biases that properly normalize the sum retrieval probabilities of each instance so that every text and video instance is fairly represented during cross-modal retrieval. Empirical study shows that NCL brings consistent and significant gains in text-video retrieval on different model architectures, with new state-of-the-art multimodal retrieval metrics on the ActivityNet, MSVD, and MSR-VTT datasets without any architecture engineering.
更多查看译文
关键词
contrastive learning,text-video
AI 理解论文
溯源树
样例
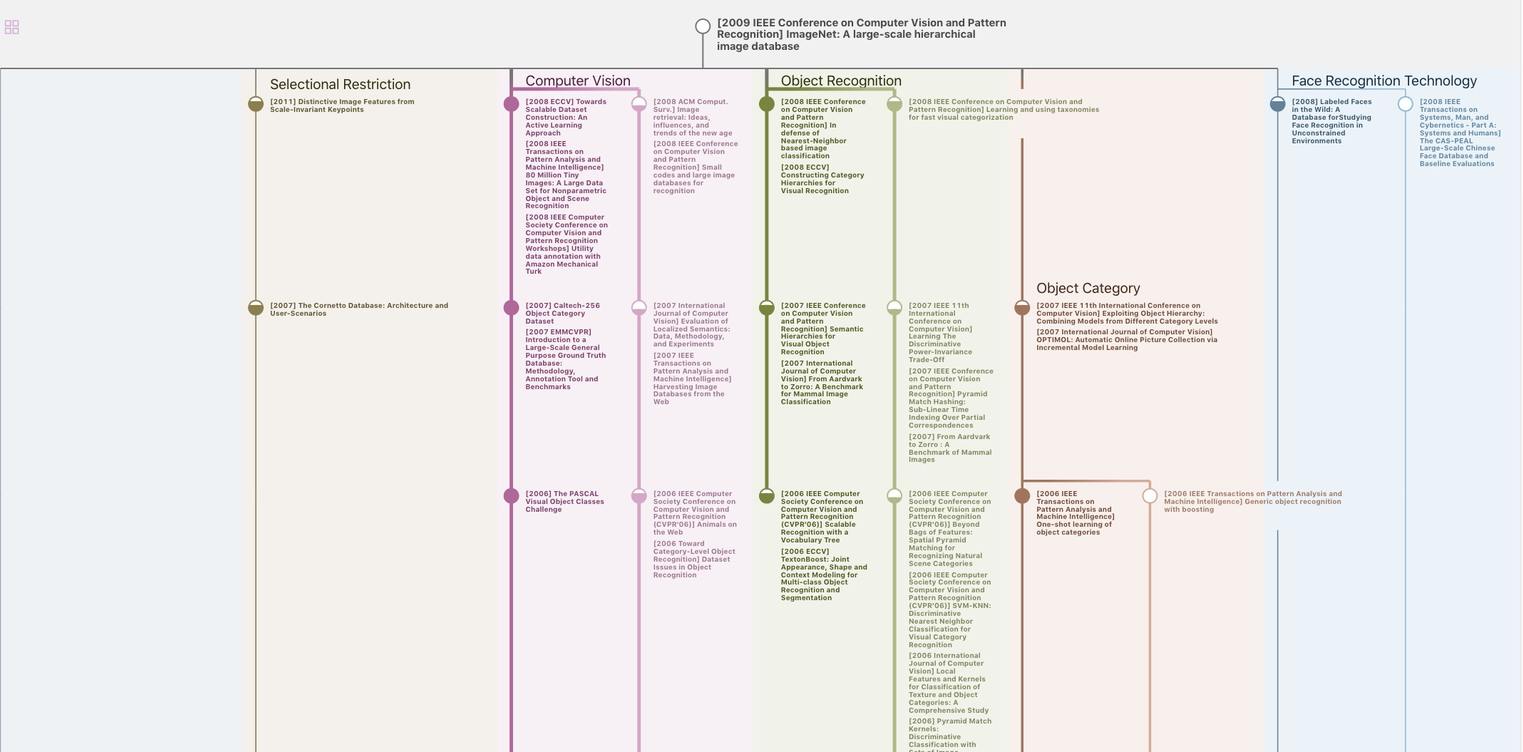
生成溯源树,研究论文发展脉络
Chat Paper
正在生成论文摘要