Vision Transformer With Contrastive Learning for Remote Sensing Image Scene Classification
IEEE Journal of Selected Topics in Applied Earth Observations and Remote Sensing(2022)
摘要
Remote sensing images (RSIs) are characterized by complex spatial layouts and ground object structures. ViT can be a good choice for scene classification owing to the ability to capture long-range interactive information between patches of input images. However, due to the lack of some inductive biases inherent to CNNs, such as locality and translation equivariance, ViT cannot generalize well when trained on insufficient amounts of data. Compared with training ViT from scratch, transferring a large-scale pretrained one is more cost-efficient with better performance even when the target data are small scale. In addition, the cross-entropy (CE) loss is frequently utilized in scene classification yet has low robustness to noise labels and poor generalization performances for different scenes. In this article, a ViT-based model in combination with supervised contrastive learning (CL) is proposed, named ViT-CL. For CL, supervised contrastive (SupCon) loss, which is developed by extending the self-supervised contrastive approach to the fully supervised setting, can explore the label information of RSIs in embedding space and improve the robustness to common image corruption. In ViT-CL, a joint loss function that combines CE loss and SupCon loss is developed to prompt the model to learn more discriminative features. Also, a two-stage optimization framework is introduced to enhance the controllability of the optimization process of the ViT-CL model. Extensive experiments on the AID, NWPU-RESISC45, and UCM datasets verified the superior performance of ViT-CL, with the highest accuracies of 97.42%, 94.54%, and 99.76% among all competing methods, respectively.
更多查看译文
关键词
Joint loss function,remote sensing,scene classification,supervised contrastive (SupCon) loss,vision transformer
AI 理解论文
溯源树
样例
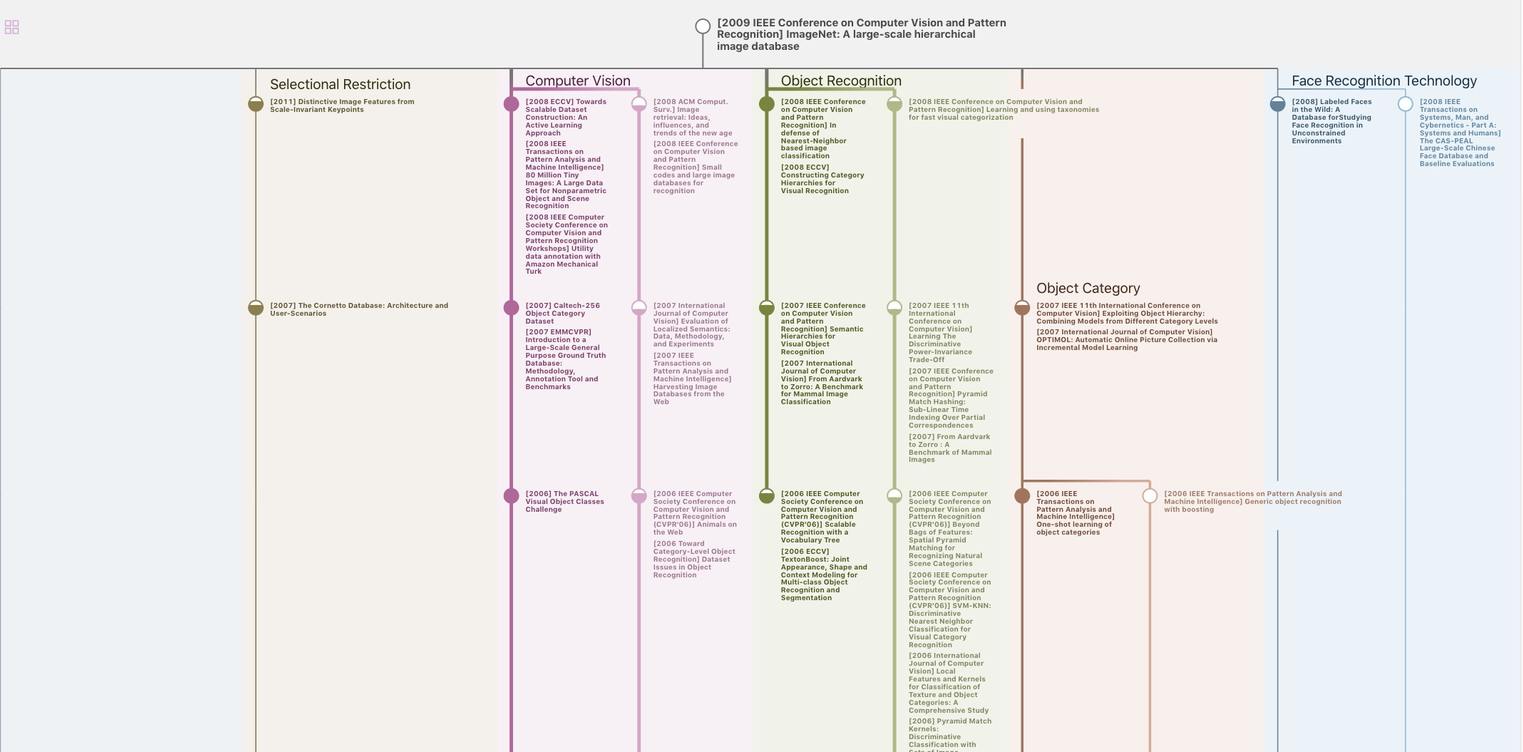
生成溯源树,研究论文发展脉络
Chat Paper
正在生成论文摘要