Wheat leaf traits monitoring based on machine learning algorithms and high-resolution satellite imagery
Ecological Informatics(2023)
摘要
The leaf, which is a crucial indicator for evaluating crop status, plays an important role in plants' functions. Determining and monitoring leaf parameters can facilitate the detection and estimation of crop yield, which is essential for food security. Crop monitoring by remote sensing technology is critical to support crop production, especially over large scales. In this study, we developed a methodology to estimate leaf parameters based entirely on vegetation indices (VIs) from remotely sensed imagery in wheat under different management practices. Therefore, the current study aimed to examine the utility of VIs calculated from the sentinel-2 data in estimating the Leaf area index (LAI) and leaf parameters at wheat farms using machine learning algorithms. Leaf parameters included leaf dry weight (LDW), specific leaf area (SLA) and leaf specific weight (SLW), and machine learning algorithms were SVM (support vector machine), ANN (artificial neural network) and DNN (deep neural network). Leaf parameters were measured at several developmental stages of wheat in two contrasting environments in the southern Iran. The results demonstrated that the DNN algorithm could efficiently predict leaf parameters in the southern Iran with an overall precision of >72%, which assessed the potential of employing DNN to achieve the temporal and spatial distribution data of wheat based on the Sentinel-2 imagery. The validation of the DNN model generally showed high accuracy (R = 0.80, RMSE = 1.19, and MAE = 0.98) between observed and estimated LAI values when this model was used. NDVI was also highly sensitive to wheat LDW and SLA parameters, with a good correlation between field measurements and those predicted by the DNN model from sentinel-2 imagery, with the R values of 0.66 and 0.85, respectively. Further, NDVI and PVI (Perpendicular Vegetation Index) were linearly correlated with SLW across both temporal and spatial scales (R = 0.79). Among VIs considered from sentinel-2 imagery to predict wheat leaf parameters, NDVI was more sensitive than other VIs. This research, thus, indicated that using sentinel-2 data within a DNN model could provide a comparatively precise and robust prediction of leaf parameters and yield valuable insights into crop management with high temporal and spatial accuracy.
更多查看译文
关键词
Leaf parameters,Vegetation indices,Sentinel-2,Support vector machine,Artificial neural network,Deep neural network
AI 理解论文
溯源树
样例
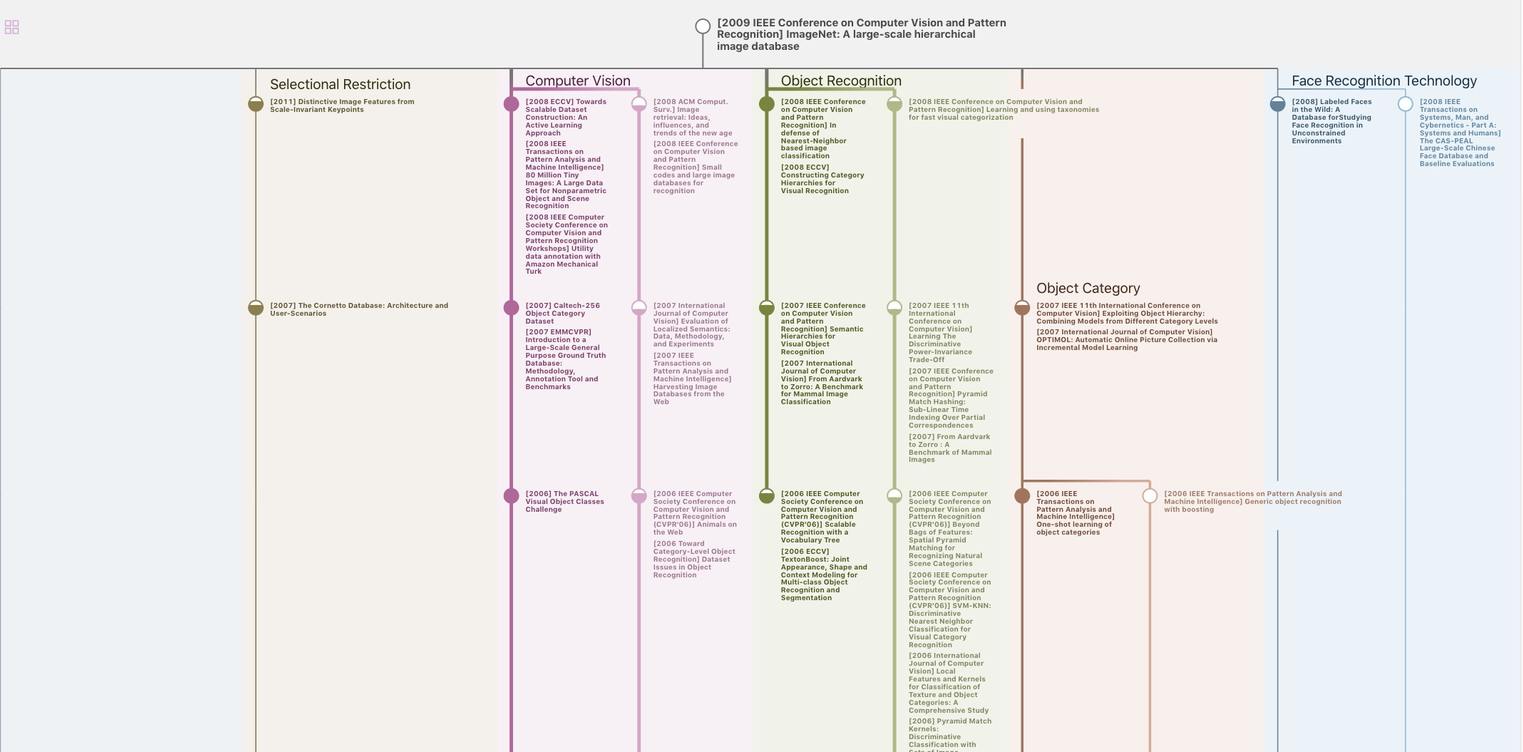
生成溯源树,研究论文发展脉络
Chat Paper
正在生成论文摘要