Dynamic Molecular Graph-based Implementation for Biophysical Properties Prediction
arxiv(2022)
摘要
Neural Networks (GNNs) have revolutionized the molecular discovery to understand patterns and identify unknown features that can aid in predicting biophysical properties and protein-ligand interactions. However, current models typically rely on 2-dimensional molecular representations as input, and while utilization of 2\3- dimensional structural data has gained deserved traction in recent years as many of these models are still limited to static graph representations. We propose a novel approach based on the transformer model utilizing GNNs for characterizing dynamic features of protein-ligand interactions. Our message passing transformer pre-trains on a set of molecular dynamic data based off of physics-based simulations to learn coordinate construction and make binding probability and affinity predictions as a downstream task. Through extensive testing we compare our results with the existing models, our MDA-PLI model was able to outperform the molecular interaction prediction models with an RMSE of 1.2958. The geometric encodings enabled by our transformer architecture and the addition of time series data add a new dimensionality to this form of research.
更多查看译文
关键词
biophysical properties
AI 理解论文
溯源树
样例
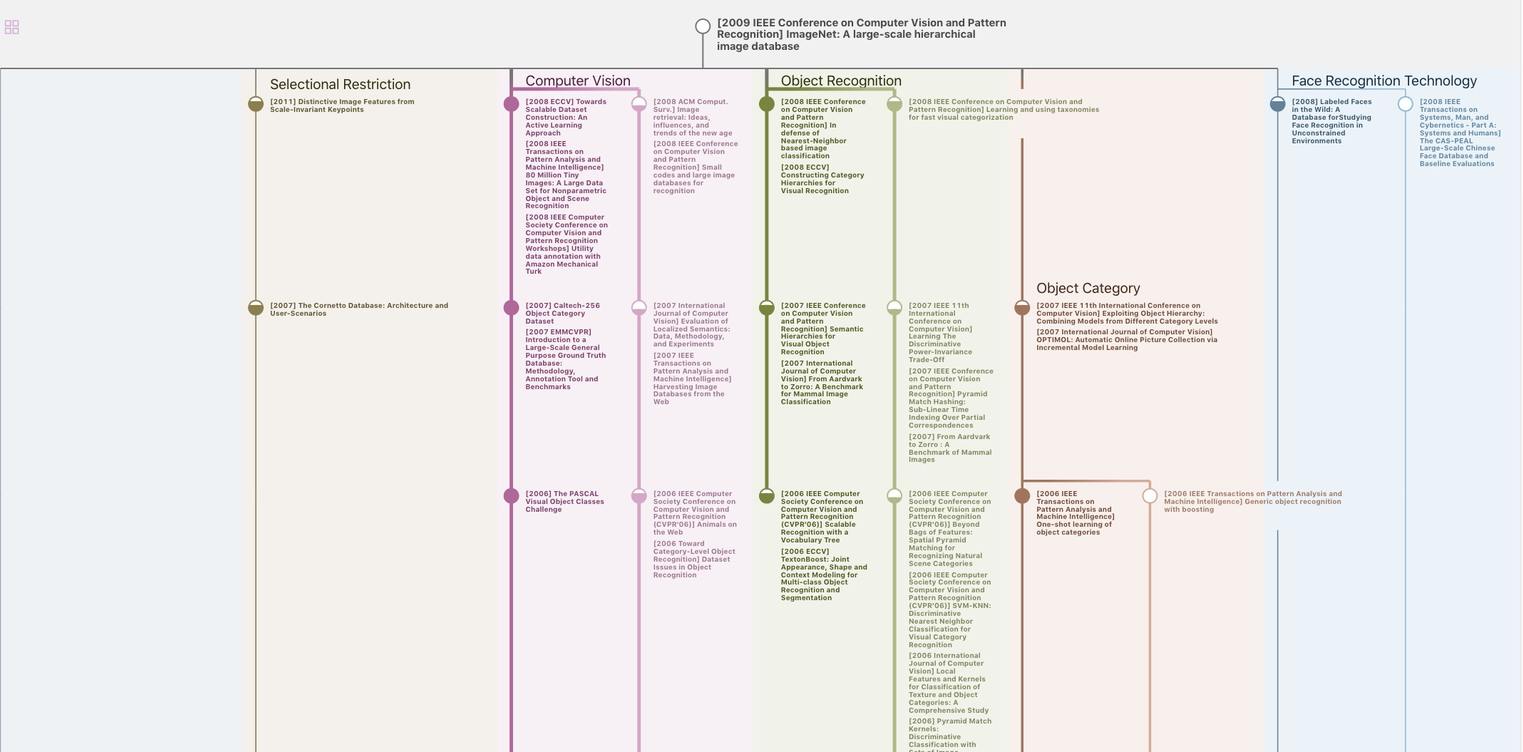
生成溯源树,研究论文发展脉络
Chat Paper
正在生成论文摘要