Time-varying Online Transfer Learning for Intelligent Bearing Fault Diagnosis with Incomplete Unlabeled Target Data
IEEE Transactions on Industrial Informatics(2022)
摘要
Intelligent transfer domain adaptation fault diagnosis approaches have received more and more attention. An important prerequisite for the effectiveness of the existing transfer diagnosis methods is to have complete target machine data of all failure types for domain adaptation. However, in practical applications, it is often difficult and time-consuming to obtain all kinds of target fault data. In addition, the diagnosis model cannot be updated to adapt to the actual operating conditions. To overcome these limitations, we propose a time-varying online transfer learning (TVOTL) model for intelligent fault diagnosis of rolling bearings, which can not only construct the transfer diagnosis model with incomplete unlabeled target data offline but also update dynamically during online application. In the offline stage, the initial feature transformation matrix is first obtained using limited inter-domain data and the raw data are mapped to the corresponding feature space to get new representations. Then, in the online stage, data distribution discrepancies between domains are further reduced with online collected target instances and the transfer diagnosis model is updated. In the case study, the proposed TVOTL model is tested on ten transfer diagnosis tasks to illustrate the outstanding performance compared with other online fault diagnosis models.
更多查看译文
关键词
Fault diagnosis,online transfer learning,rolling bearing,time-varying model
AI 理解论文
溯源树
样例
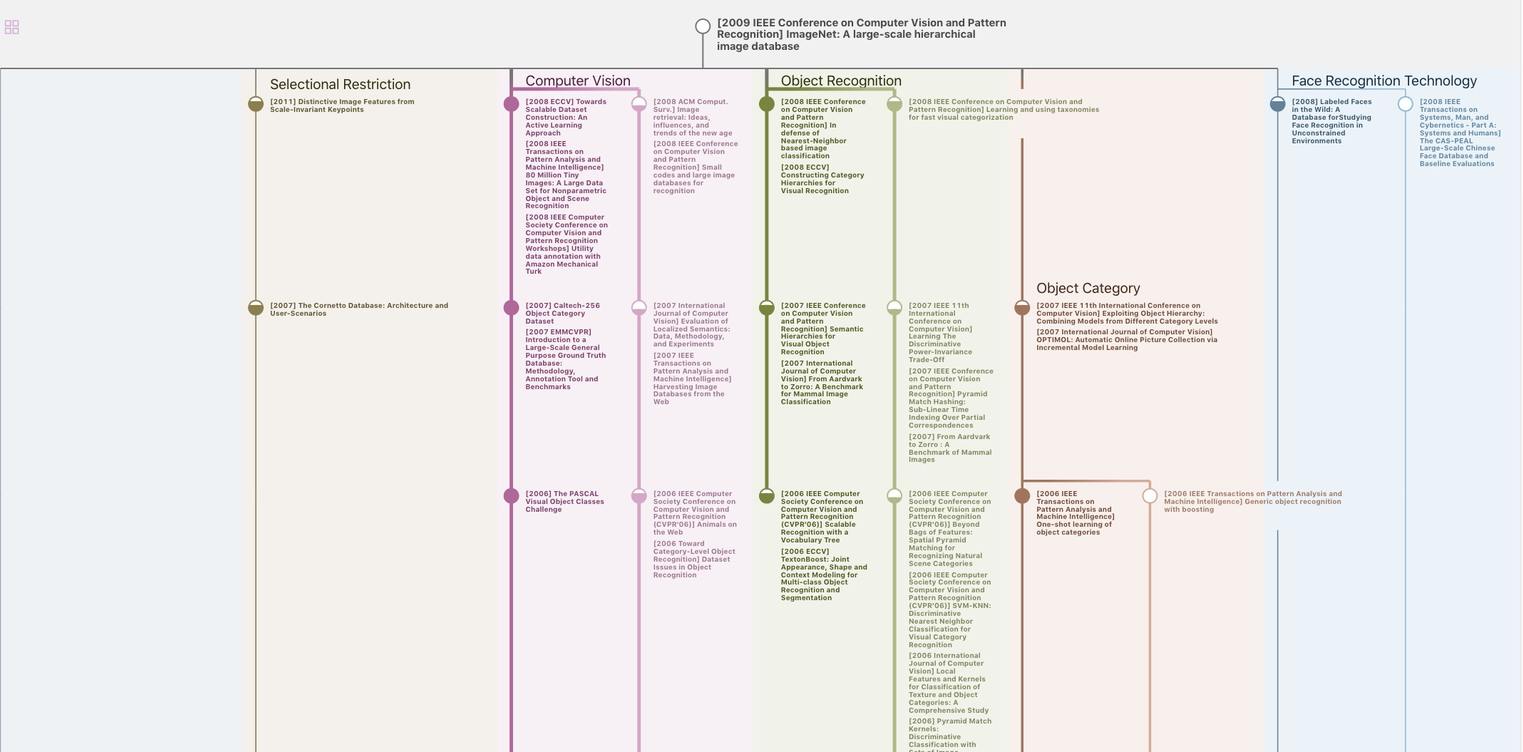
生成溯源树,研究论文发展脉络
Chat Paper
正在生成论文摘要