A constraint score guided meta-heuristic searching to attribute reduction
Journal of Intelligent & Fuzzy Systems(2023)
摘要
Essentially, the problem solving of attribute reduction can be regarded as a process of reduct searching which will be terminated if a pre-defined restriction is achieved. Presently, among a variety of searching strategies, meta-heuristic searching has been widely accepted. Nevertheless, it should be emphasized that the iterative procedures in most meta-heuristic algorithms rely heavily on the random generation of initial population, such a type of generation is naturally associated with the limitations of inferior stability and performance. Therefore, a constraint score guidance is proposed before carrying out meta-heuristic searching and then a novel framework to seek out reduct is developed. Firstly, for each attribute and each label in data, the index called local constraint score is calculated. Secondly, the qualified attributes are identified by those constraint scores, which consist of the foundation of initial population. Finally, the meta-heuristic searching can be further employed to achieve the required restriction in attribute reduction. Note that most existing meta-heuristic searchings and popular measures (evaluate the significance of attributes) can be embedded into our framework. Comprehensive experiments over 20 public datasets clearly validated the effectiveness of our framework: it is beneficial to reduct with superior stabilities, and the derived reduct may further contribute to the improvement of classification performance.
更多查看译文
关键词
Attribute reduction,constraint score,meta-heuristic searching,rough set
AI 理解论文
溯源树
样例
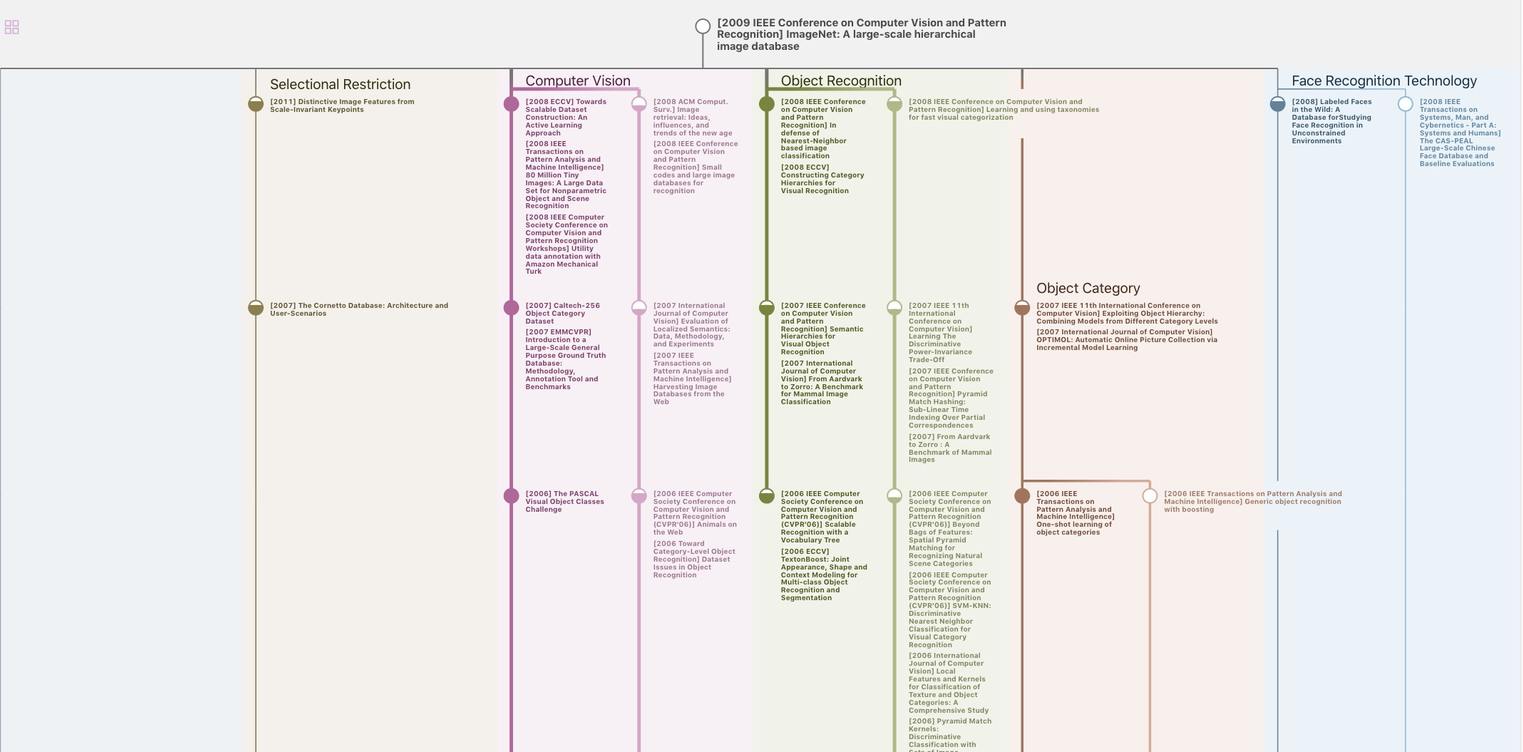
生成溯源树,研究论文发展脉络
Chat Paper
正在生成论文摘要