Capturing Morphometric Patterns of Alzheimer's Disease Progression with a TV‐L1 Ordinal Machine Learning Model
Alzheimer's & Dementia(2022)
摘要
Background The most common neurodegenerative disease, Alzheimer’s disease (AD) constitutes about two thirds of cases of dementia overall [1]. Mild cognitive impairment (MCI) is considered to be a transitional stage between aging and AD [2]. This general AD staging information and ample MCI data motivate a further investigation on the subcortical shape analysis incorporating MCI data in AD study. We therefore designed a novel logistic regression model to explore more morphometric patterns associated with the disease progression of AD. Method In this study, we used T1‐weighted MRI data of 225 controls, 398 MCI patients and 195 AD patients from the ADNI‐1 dataset. We designed an ordinal logistic regression model [3] regularized by total variation (TV) and L1 penalization term [4]. Unlike the binary or conventional multi‐class classifiers, our TV‐L1 ordinal classifier is capable of sparse‐spatially utilizing ordinal morphometric features to learn potential ordinal patterns and predict normal control, MCI and AD all at once in a single model. We extracted vertex‐wise thickness features in 7 subcortical regions [5] and trained our model with all 14 regions of interest (ROI) across the whole brain. To evaluate the performance and find the optimal hyper‐parameters in our classification task, we computed the precision, recall score and f‐score with a 4‐fold cross‐validation (CV) grid search. For comparison we also trained a TV‐L1 logistic regression model binary classifiers for controls and AD. Result Table 1 shows the confusion matrix, precision, recall score and f‐score. Based on the 4‐fold CV, the average precision and recall score for control, MCI and AD are 0.457 and 0.443 respectively. The weights map of the trained TV‐L1 logistic regression model is shown in Figure 1 , and that of the TV‐L1 ordinal classifier in Figure 2 . Conclusion By designing an TV‐L1 ordinal logistic regression machine learning model, we performed a shape feature‐based classification for controls, MCI and AD with f1 score substantially above chance across all three AD stages. The trained weights map reveals subtle morphometric patterns associated with the disease progression from a cognitively normal state to MCI and eventually AD stage. This could potentially provide more insights for clinical studies of AD.
更多查看译文
关键词
alzheimer disease,morphometric patterns,disease progression,machine learning
AI 理解论文
溯源树
样例
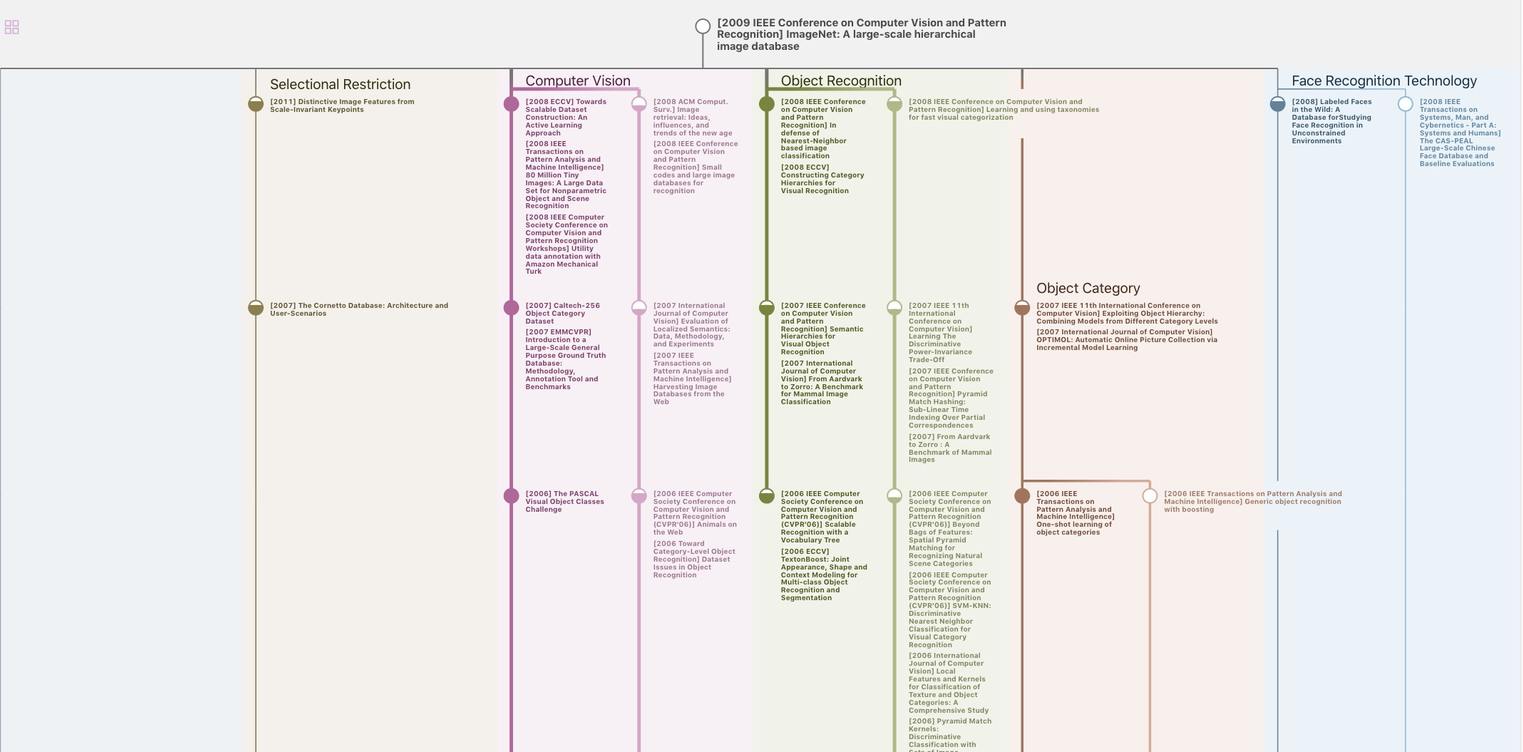
生成溯源树,研究论文发展脉络
Chat Paper
正在生成论文摘要