Sea surface height super-resolution using high-resolution sea surface temperature with a subpixel convolutional residual network
Environmental Data Science(2022)
摘要
Abstract The oceans have a very important role in climate regulation due to their massive heat storage capacity. Thus, for the past decades, oceans have been observed by satellites to better understand their dynamics. Satellites retrieve several data with various spatial resolutions. For instance, sea surface height (SSH) is a low-resolution data field where sea surface temperature (SST) can be retrieved in a much higher one. These two physical parameters are linked by a physical link that can be learned by a super-resolution machine-learning algorithm. In this work, we present a subpixel convolutional deep learning model that takes advantage of the higher resolution SST field to guide the downscaling of the SSH one. The data fields that we use are simulated by a physic-based ocean model at a higher sampling rate than the satellites provide. We compared our approach with a convolutional neural network model. Our architecture generalized well with validation performances of 3.94 cm root mean squared error (RMSE) and training performances of 2.65 cm RMSE.
更多查看译文
AI 理解论文
溯源树
样例
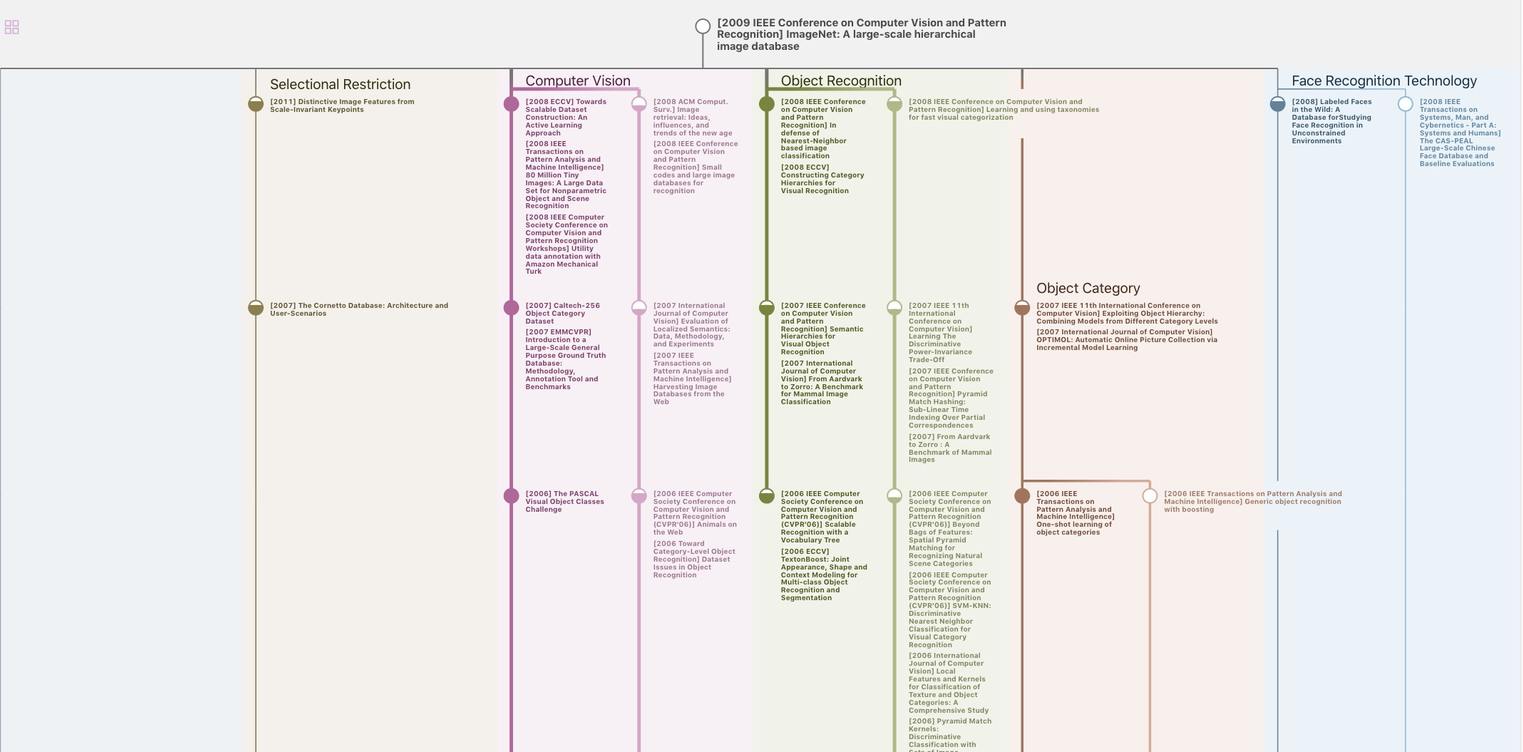
生成溯源树,研究论文发展脉络
Chat Paper
正在生成论文摘要