Total organic carbon estimation in seagrass beds in tauranga harbour, new zealand using multi-sensors imagery and grey wolf optimization
Geocarto International(2022)
摘要
Estimation of carbon stock in seagrass meadows is in challenges of paucity of assessment and low accuracy of the estimates. In this study, we used a fusion of the synthetic aperture radar (SAR) Sentinel-1 (S-1), the multi-spectral Sentinel-2 (S-2), and coupled this with advanced machine learning (ML) models and meta-heuristic optimization to improve the estimation of total organic carbon (TOC) stock in the Zostera muelleri meadows in Tauranga Harbour, New Zealand. Five scenarios containing combinations of data, ML models (Random Forest, Extreme Gradient Boost, Rotation Forest, CatBoost) and optimization were developed and evaluated for TOC retrieval. Results indicate a fusion of S1, S2 images, a novel ML model CatBoost and the grey wolf optimization algorithm (the CB-GWO model) yielded the best prediction of seagrass TOC (R (2), RMSE were 0.738 and 10.64 Mg C ha(-1)). Our results provide novel ideas of deriving a low-cost, scalable and reliable estimates of seagrass TOC globally.
更多查看译文
关键词
Seagrass,total organic carbon,Sentinel image,CatBoost,metaheuristic optimization
AI 理解论文
溯源树
样例
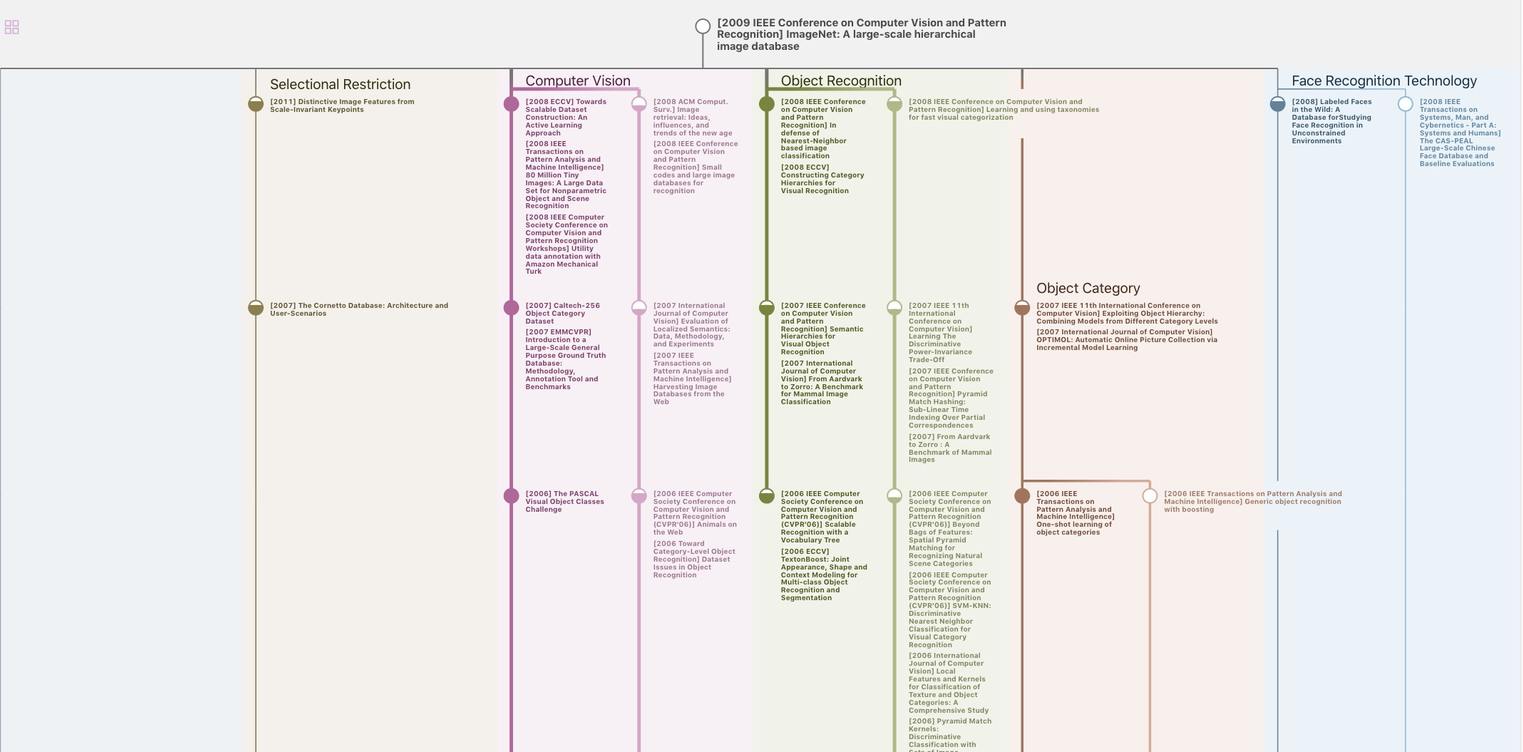
生成溯源树,研究论文发展脉络
Chat Paper
正在生成论文摘要