Combining Remote and In-situ Sensing for Autonomous Underwater Vehicle Localization and Navigation
OCEANS 2022, Hampton Roads(2022)
摘要
Scientists continue to study the red tide and fish-kill events happening in Florida. Machine learning applications using remote sensing data on coastal waters to monitor water quality parameters and detect harmful algal blooms are also being studied. Unmanned Surface Vehicles (USVs) and Autonomous Underwater Vehicles (AUVs) are often deployed on data collection and disaster response missions. To enhance study and mitigation efforts, robots must be able to use available data to navigate these underwater environments. In this study, we compute a satellite-derived underwater environment (SDUE) model by implementing a supervised machine learning model where remote sensing reflectance (R-rs) indices are labeled with in-situ data they correlate with. The models predict bathymetry and water quality parameters given a recent remote sensing image. In our experiment, we use Sentinel-2 (S2) images and in-situ data of the Biscayne Bay to create an SDUE that can be used as a Chlorophyll-a map. The SDUE is then used in an Extended Kalman Filter (EKF) application that solves an underwater vehicle localization and navigation problem.
更多查看译文
关键词
remote sensing, machine learning, water quality, linear regression, estimation, autonomous underwater vehicle, chlorophyll-a, robots
AI 理解论文
溯源树
样例
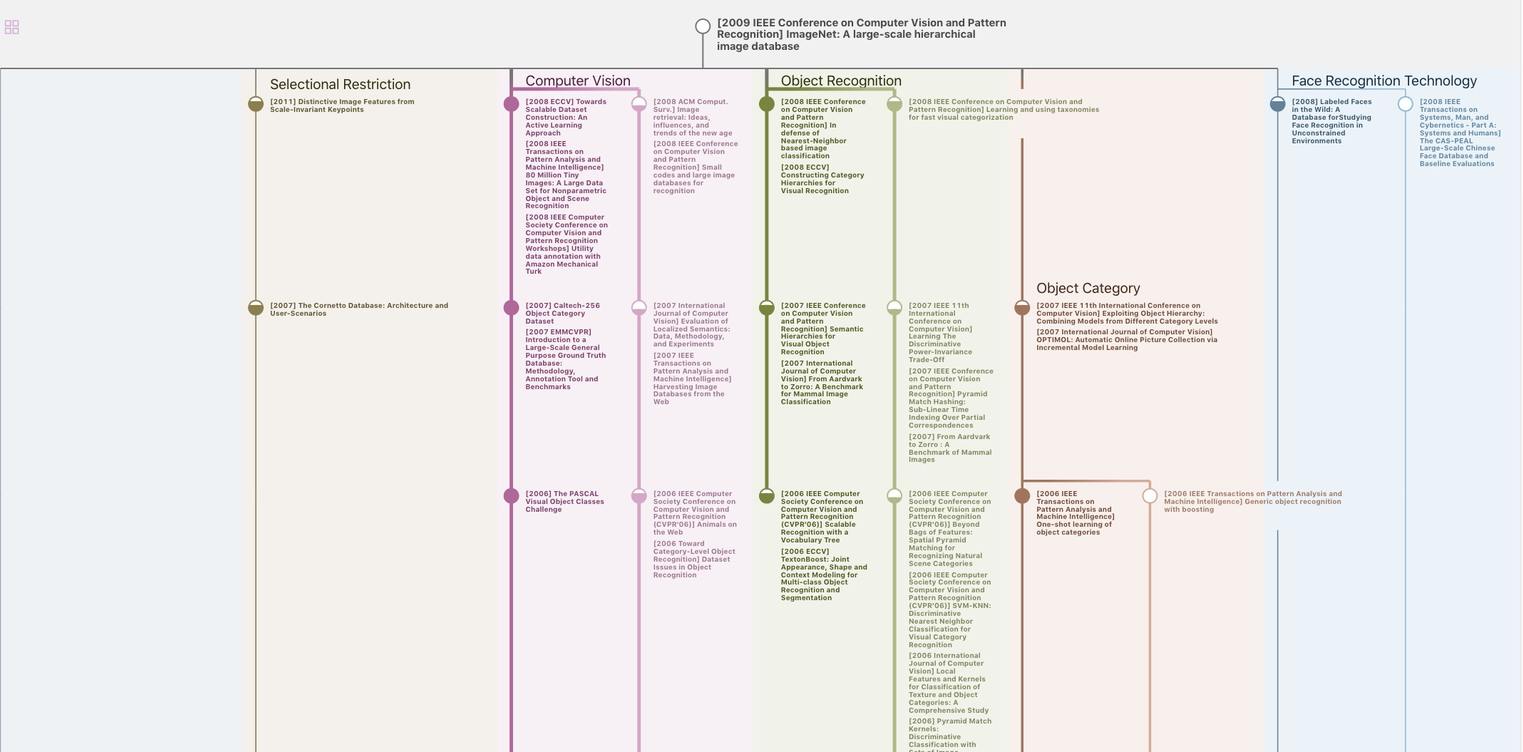
生成溯源树,研究论文发展脉络
Chat Paper
正在生成论文摘要