Continual Learning for Instruction Following from Realtime Feedback.
CoRR(2022)
摘要
We study the problem of continually training an instruction-following agent through feedback provided by users during collaborative interactions. During interaction, human users instruct an agent using natural language, and provide realtime binary feedback as they observe the agent's instruction execution. We cast learning as a contextual bandit problem, converting the user feedback to immediate reward. We evaluate through multiple rounds of human-agent interactions, demonstrating 15.4% absolute improvement in instruction execution over time. We also show our approach is robust to several design variations, and that the feedback signal is roughly equivalent to the learning signal of supervised demonstration data.
更多查看译文
AI 理解论文
溯源树
样例
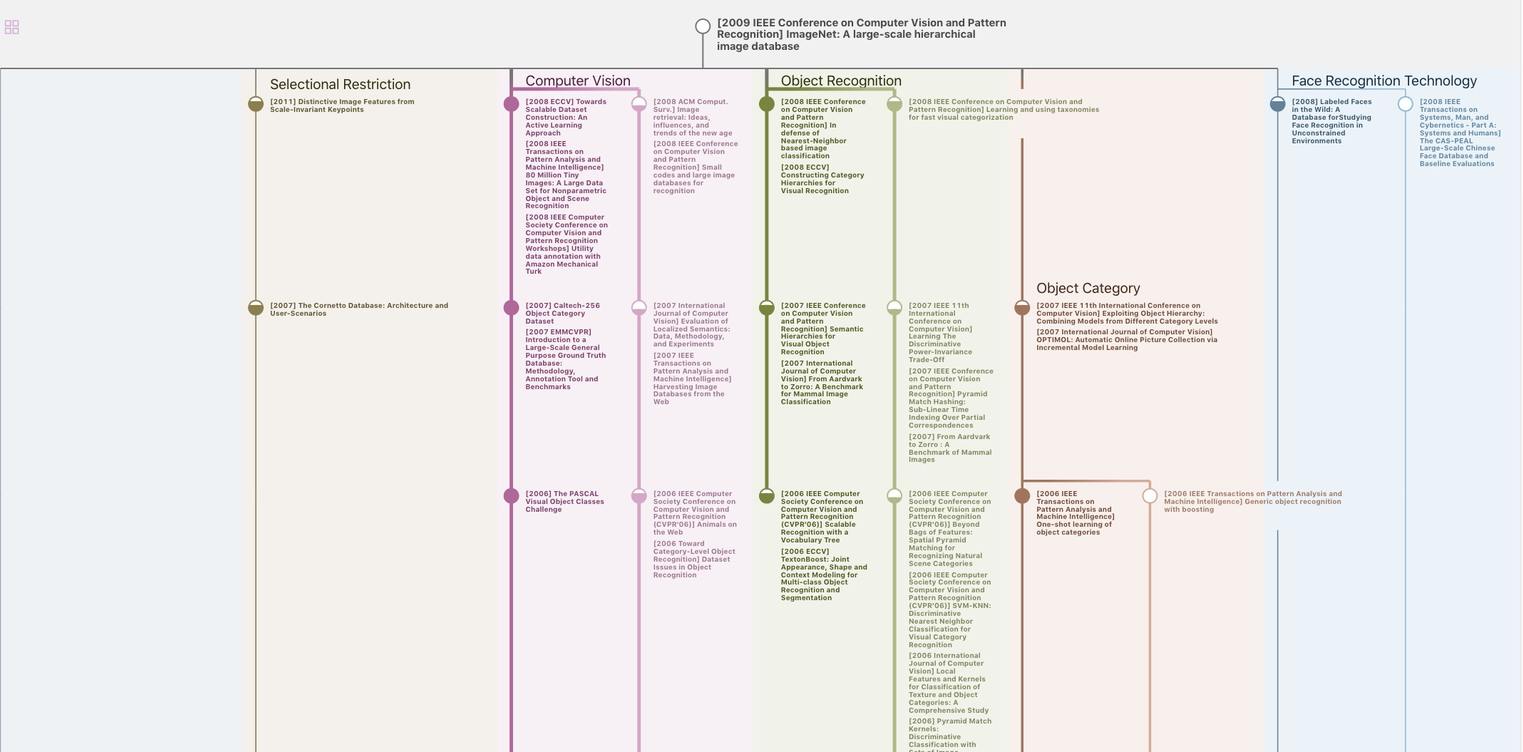
生成溯源树,研究论文发展脉络
Chat Paper
正在生成论文摘要