JFP: Joint Future Prediction with Interactive Multi-Agent Modeling for Autonomous Driving
arxiv(2022)
摘要
We propose JFP, a Joint Future Prediction model that can learn to generate accurate and consistent multi-agent future trajectories. For this task, many different methods have been proposed to capture social interactions in the encoding part of the model, however, considerably less focus has been placed on representing interactions in the decoder and output stages. As a result, the predicted trajectories are not necessarily consistent with each other, and often result in unrealistic trajectory overlaps. In contrast, we propose an end-to-end trainable model that learns directly the interaction between pairs of agents in a structured, graphical model formulation in order to generate consistent future trajectories. It sets new state-of-the-art results on Waymo Open Motion Dataset (WOMD) for the interactive setting. We also investigate a more complex multi-agent setting for both WOMD and a larger internal dataset, where our approach improves significantly on the trajectory overlap metrics while obtaining on-par or better performance on single-agent trajectory metrics.
更多查看译文
关键词
joint future prediction,multi-agent multi-agent
AI 理解论文
溯源树
样例
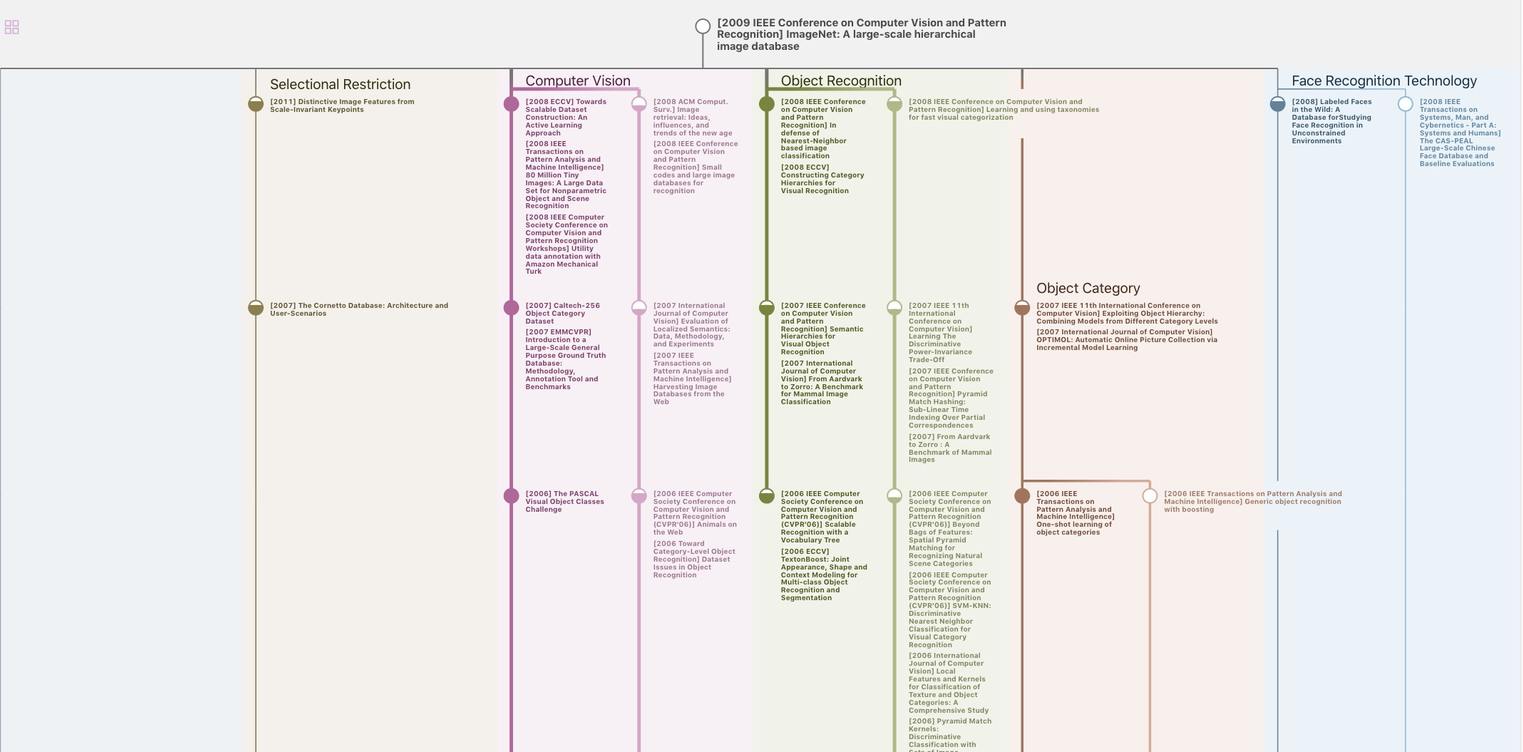
生成溯源树,研究论文发展脉络
Chat Paper
正在生成论文摘要