Nested Gradient Codes for Straggler Mitigation in Distributed Machine Learning
arxiv(2022)
摘要
We consider distributed learning in the presence of slow and unresponsive worker nodes, referred to as stragglers. In order to mitigate the effect of stragglers, gradient coding redundantly assigns partial computations to the worker such that the overall result can be recovered from only the non-straggling workers. Gradient codes are designed to tolerate a fixed number of stragglers. Since the number of stragglers in practice is random and unknown a priori, tolerating a fixed number of stragglers can yield a sub-optimal computation load and can result in higher latency. We propose a gradient coding scheme that can tolerate a flexible number of stragglers by carefully concatenating gradient codes for different straggler tolerance. By proper task scheduling and small additional signaling, our scheme adapts the computation load of the workers to the actual number of stragglers. We analyze the latency of our proposed scheme and show that it has a significantly lower latency than gradient codes.
更多查看译文
关键词
straggler mitigation,distributed,codes,machine
AI 理解论文
溯源树
样例
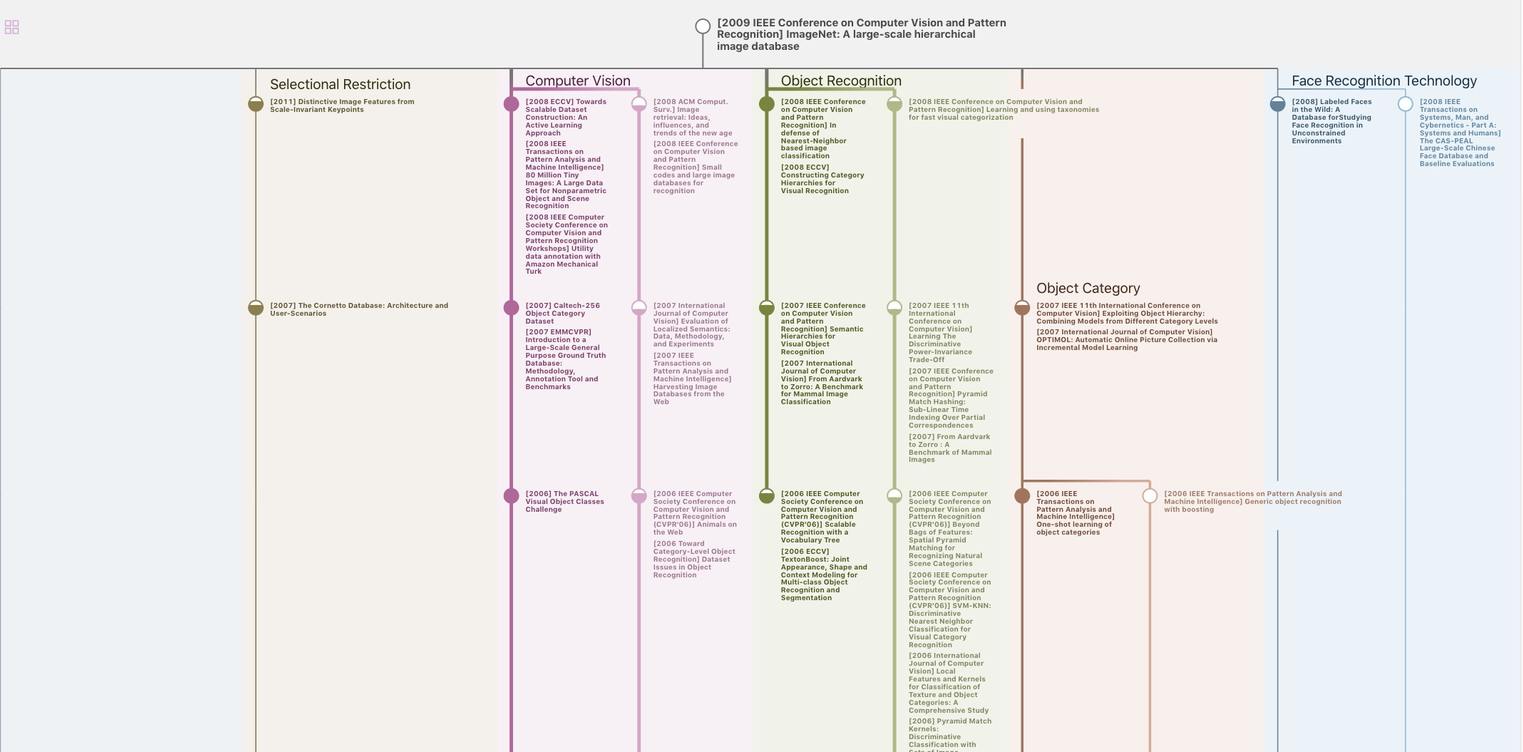
生成溯源树,研究论文发展脉络
Chat Paper
正在生成论文摘要