Federated Learning with Flexible Control
arxiv(2022)
摘要
Federated learning (FL) enables distributed model training from local data collected by users. In distributed systems with constrained resources and potentially high dynamics, e.g., mobile edge networks, the efficiency of FL is an important problem. Existing works have separately considered different configurations to make FL more efficient, such as infrequent transmission of model updates, client subsampling, and compression of update vectors. However, an important open problem is how to jointly apply and tune these control knobs in a single FL algorithm, to achieve the best performance by allowing a high degree of freedom in control decisions. In this paper, we address this problem and propose FlexFL - an FL algorithm with multiple options that can be adjusted flexibly. Our FlexFL algorithm allows both arbitrary rates of local computation at clients and arbitrary amounts of communication between clients and the server, making both the computation and communication resource consumption adjustable. We prove a convergence upper bound of this algorithm. Based on this result, we further propose a stochastic optimization formulation and algorithm to determine the control decisions that (approximately) minimize the convergence bound, while conforming to constraints related to resource consumption. The advantage of our approach is also verified using experiments.
更多查看译文
关键词
Compressed model update,federated learning,partial participation,stochastic optimization
AI 理解论文
溯源树
样例
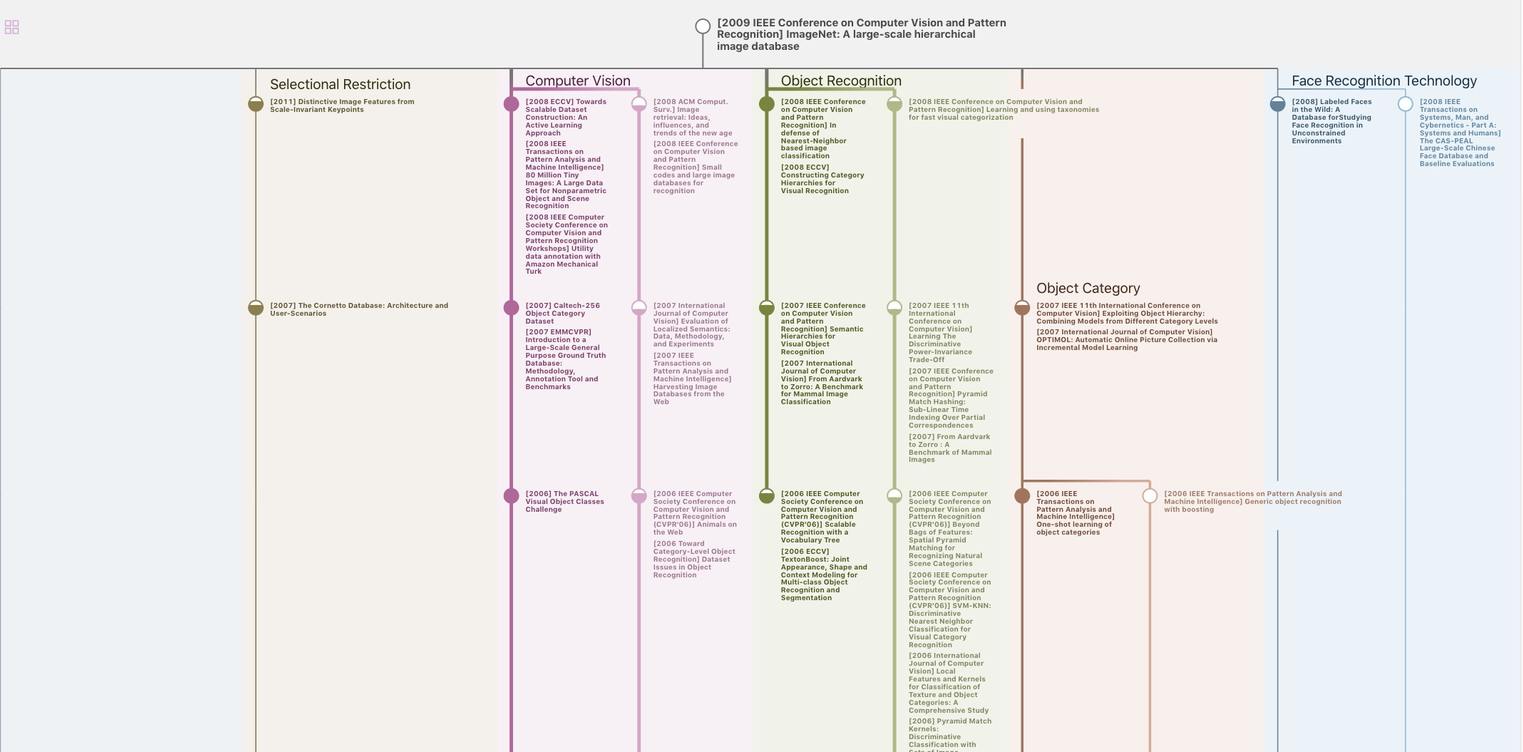
生成溯源树,研究论文发展脉络
Chat Paper
正在生成论文摘要