Effectiveness of Text, Acoustic, and Lattice-based representations in Spoken Language Understanding tasks
arxiv(2022)
摘要
In this paper, we perform an exhaustive evaluation of different
representations to address the intent classification problem in a Spoken
Language Understanding (SLU) setup. We benchmark three types of systems to
perform the SLU intent detection task: 1) text-based, 2) lattice-based, and a
novel 3) multimodal approach. Our work provides a comprehensive analysis of
what could be the achievable performance of different state-of-the-art SLU
systems under different circumstances, e.g., automatically- vs.
manually-generated transcripts. We evaluate the systems on the publicly
available SLURP spoken language resource corpus. Our results indicate that
using richer forms of Automatic Speech Recognition (ASR) outputs, namely
word-consensus-networks, allows the SLU system to improve in comparison to the
1-best setup (5.5
learning from acoustic and text embeddings, obtains performance similar to the
oracle setup, a relative improvement of 17.8
being a recommended alternative to overcome the limitations of working with
automatically generated transcripts.
更多查看译文
关键词
spoken language understanding tasks,representations,text,lattice-based
AI 理解论文
溯源树
样例
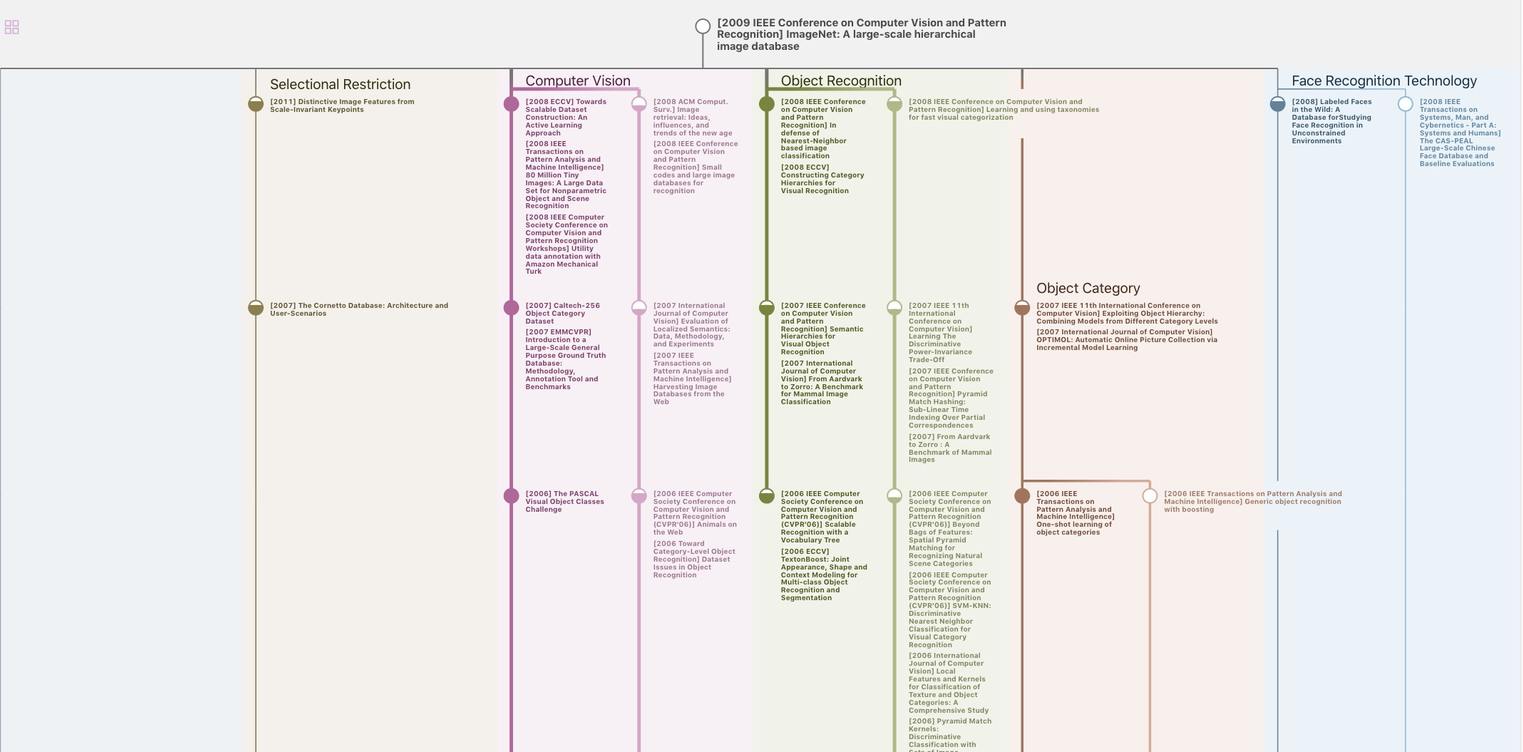
生成溯源树,研究论文发展脉络
Chat Paper
正在生成论文摘要