An Efficient Framework for Monitoring Subgroup Performance of Machine Learning Systems
arxiv(2022)
摘要
Monitoring machine learning systems post deployment is critical to ensure the reliability of the systems. Particularly importance is the problem of monitoring the performance of machine learning systems across all the data subgroups (subpopulations). In practice, this process could be prohibitively expensive as the number of data subgroups grows exponentially with the number of input features, and the process of labelling data to evaluate each subgroup's performance is costly. In this paper, we propose an efficient framework for monitoring subgroup performance of machine learning systems. Specifically, we aim to find the data subgroup with the worst performance using a limited number of labeled data. We mathematically formulate this problem as an optimization problem with an expensive black-box objective function, and then suggest to use Bayesian optimization to solve this problem. Our experimental results on various real-world datasets and machine learning systems show that our proposed framework can retrieve the worst-performing data subgroup effectively and efficiently.
更多查看译文
关键词
monitoring subgroup performance,machine
AI 理解论文
溯源树
样例
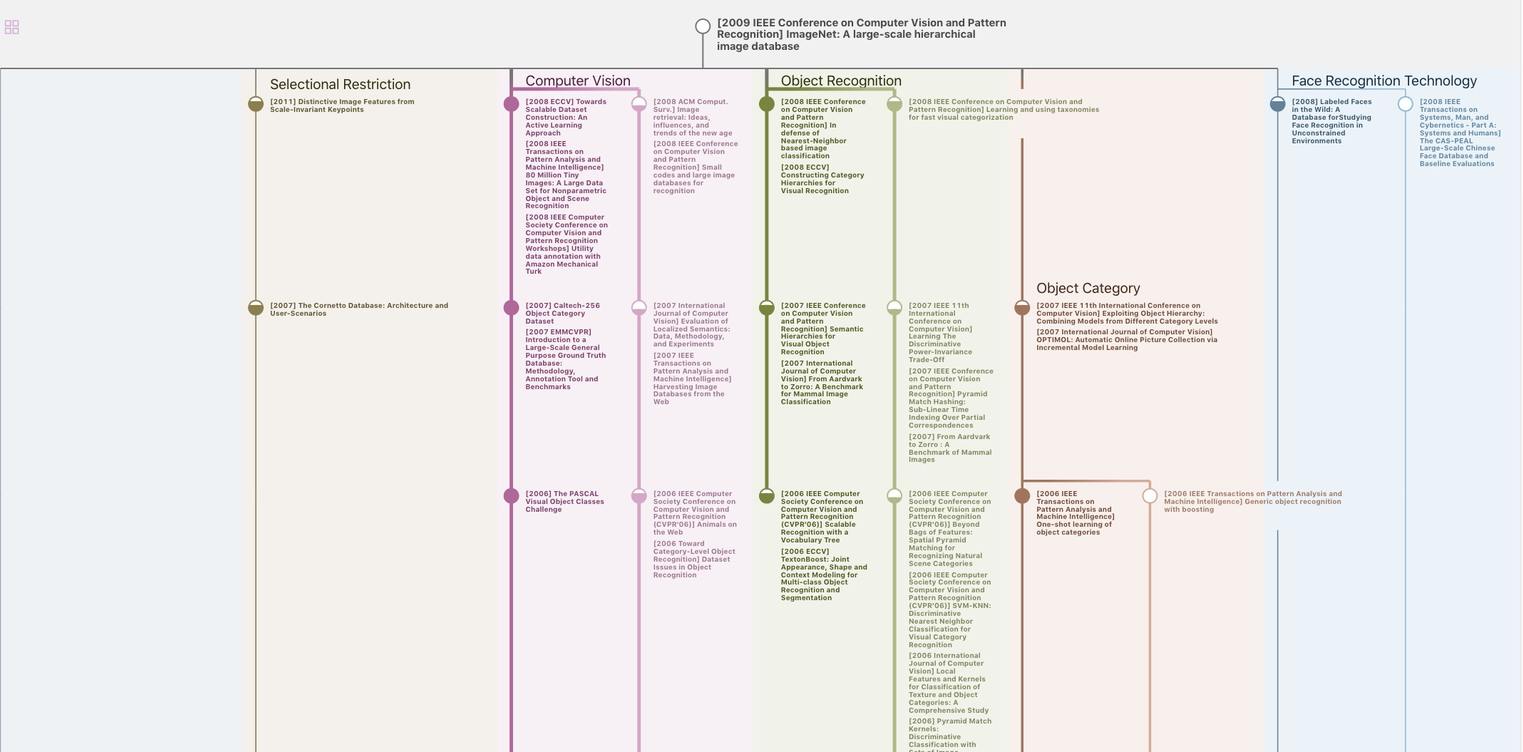
生成溯源树,研究论文发展脉络
Chat Paper
正在生成论文摘要