The KITMUS Test: Evaluating Knowledge Integration from Multiple Sources in Natural Language Understanding Systems
conf_acl(2022)
摘要
Many state-of-the-art natural language understanding (NLU) models are based on pretrained neural language models. These models often make inferences using information from multiple sources. An important class of such inferences are those that require both background knowledge, presumably contained in a model's pretrained parameters, and instance-specific information that is supplied at inference time. However, the integration and reasoning abilities of NLU models in the presence of multiple knowledge sources have been largely understudied. In this work, we propose a test suite of coreference resolution tasks that require reasoning over multiple facts. Our dataset is organized into subtasks that differ in terms of which knowledge sources contain relevant facts. We evaluate state-of-the-art coreference resolution models on our dataset. Our results indicate that several models struggle to reason on-the-fly over knowledge observed both at pretrain time and at inference time. However, with task-specific training, a subset of models demonstrates the ability to integrate certain knowledge types from multiple sources.
更多查看译文
关键词
knowledge integration,kitmus test,multiple sources
AI 理解论文
溯源树
样例
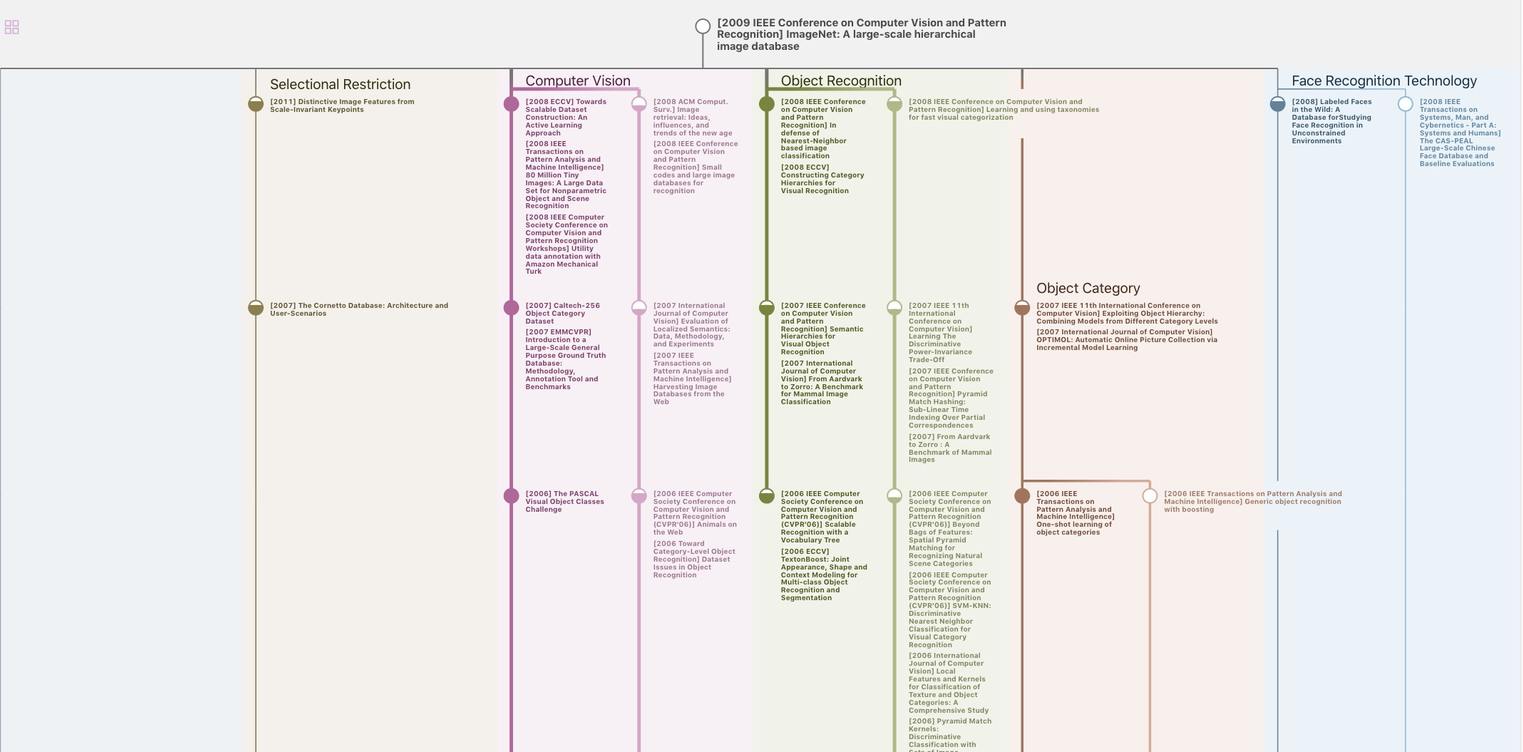
生成溯源树,研究论文发展脉络
Chat Paper
正在生成论文摘要