Learning to repeatedly solve routing problems
NETWORKS(2024)
摘要
In the last years, there has been a great interest in machine-learning-based heuristics for solving NP-hard combinatorial optimization problems. The developed methods have shown potential on many optimization problems. In this paper, we present a learned heuristic for the reoptimization of a problem after a minor change in its data. We focus on the case of the capacited vehicle routing problem with static clients (i.e., same client locations) and changed demands. Given the edges of an original solution, the goal is to predict and fix the ones that have a high chance of remaining in an optimal solution after a change of client demands. This partial prediction of the solution reduces the complexity of the problem and speeds up its resolution, while yielding a good quality solution. The proposed approach resulted in solutions with an optimality gap ranging from 0% to 1.7% on different benchmark instances within a reasonable computing time.
更多查看译文
关键词
heuristics,machine learning,reoptimization,routing
AI 理解论文
溯源树
样例
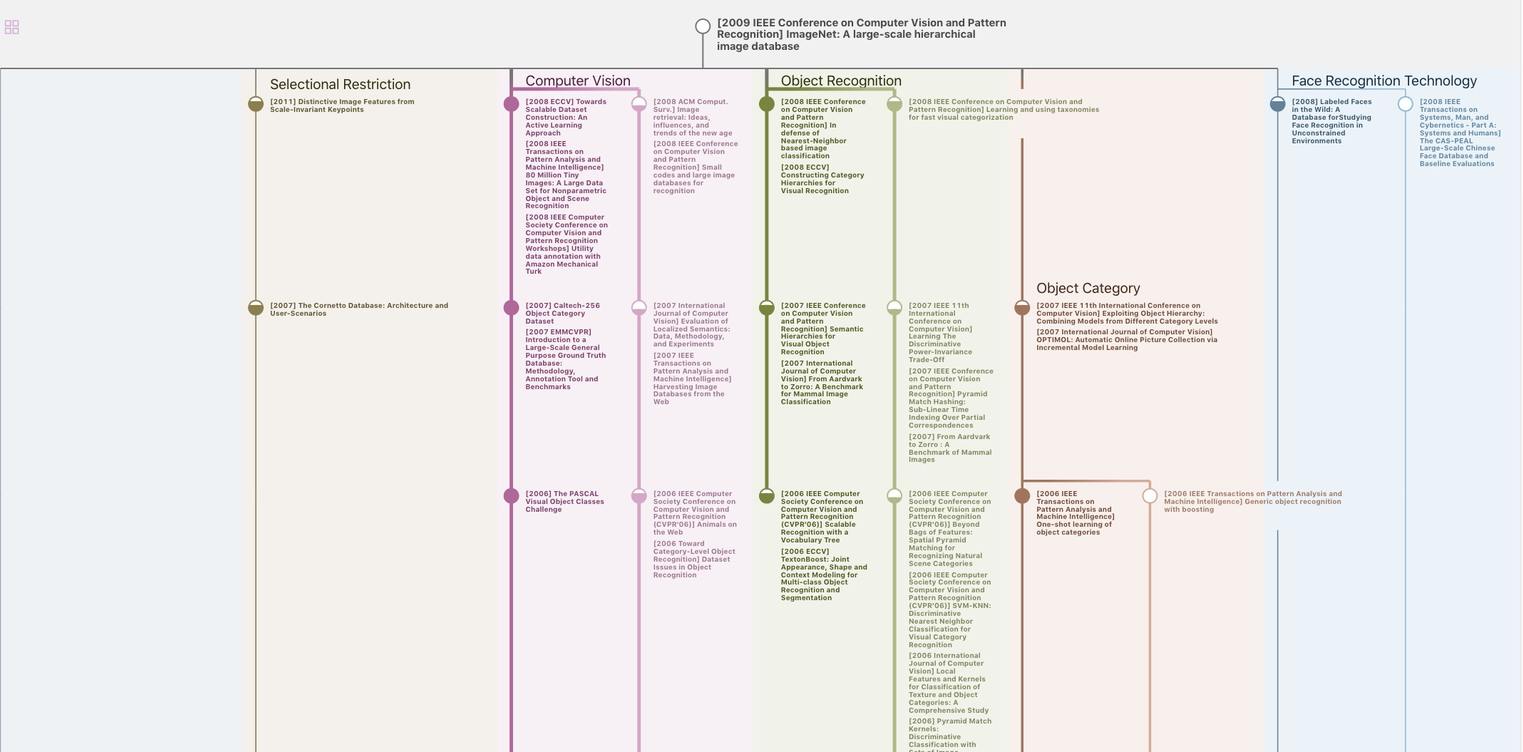
生成溯源树,研究论文发展脉络
Chat Paper
正在生成论文摘要