Power-Performance Characterization of TinyML Systems
2022 IEEE 40th International Conference on Computer Design (ICCD)(2022)
摘要
TinyML systems are enabling machine learning (ML) inference at the edge. However, there exists little quantitative analysis of such systems. This paper presents a systematic performance and power characterization of diverse TinyML applications on micro-controllers (MCUs), spanning neural network models, software libraries, operating systems, and hardware architectures. We focus on the impact of the multiple layers of abstractions that provide higher programmability at the expense of performance and energy efficiency. We propose a model to estimate the costs of different abstraction layers and make recommendations for minimizing those costs. Our findings can help designers with Neural Architecture Search (NAS) and CNN inference optimization on edge devices.
更多查看译文
关键词
TinyML,MCU,general matrix multiplication
AI 理解论文
溯源树
样例
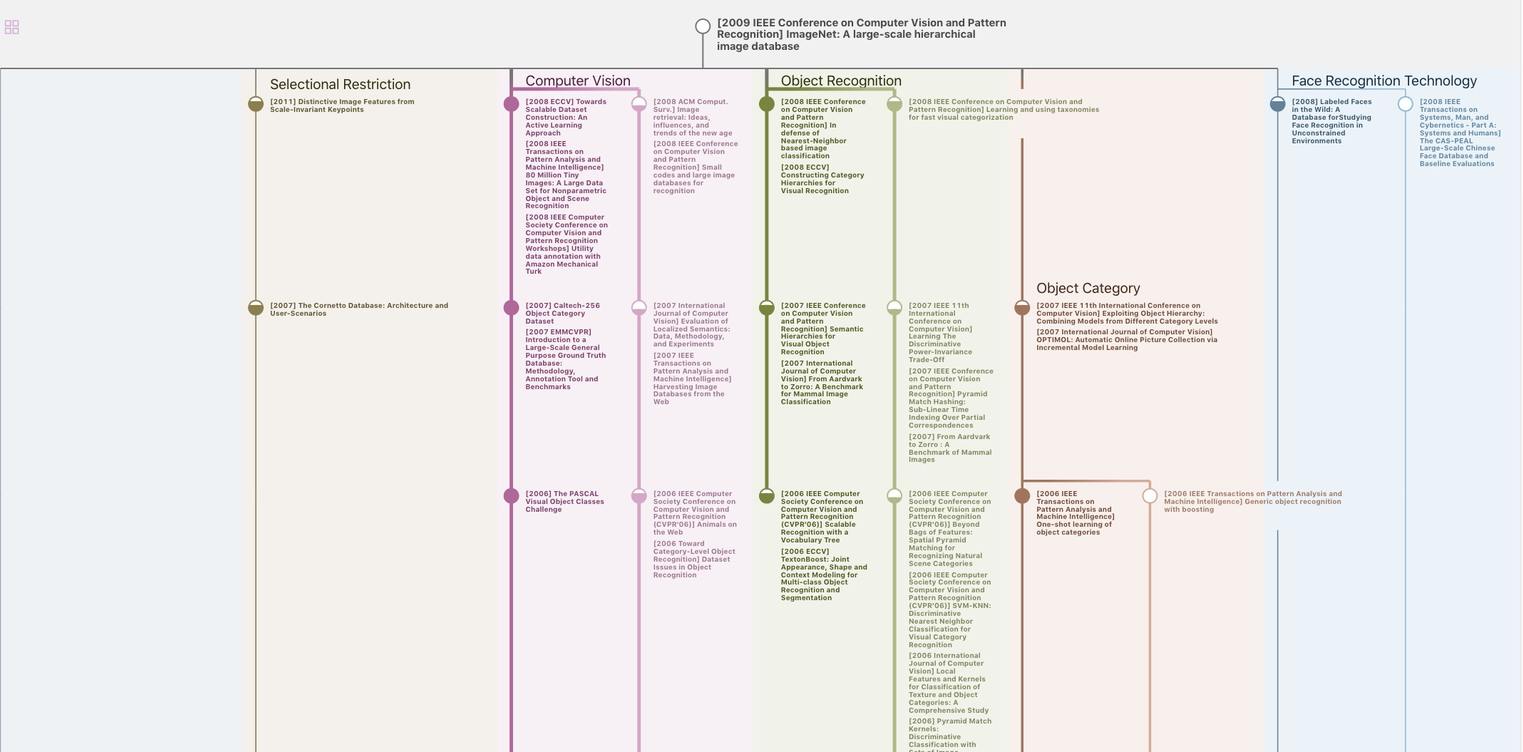
生成溯源树,研究论文发展脉络
Chat Paper
正在生成论文摘要