Improving Lung Cancer Diagnosis with Computed Tomography Radiomics and Serum Histoplasmosis Testing
Cancer Epidemiology, Biomarkers & Prevention(2022)
摘要
Abstract Background: Indeterminate pulmonary nodules (IPNs) are a diagnostic challenge in regions where pulmonary fungal disease and smoking prevalence are high. We aimed to determine the impact of a combined fungal and imaging biomarker approach compared to a validated prediction model (Mayo) to rule out benign disease and diagnose lung cancer. Methods: Adults aged 40-90 years with 6-30 mm IPNs were included from four sites. Serum samples were tested for Histoplasmosis IgG and IgM antibodies by enzyme immunoassay and a computed tomography based risk score was estimated from a validated radiomic model. Multivariable logistic regression models including Mayo score, radiomics score, and IgG and IgM Histoplasmosis antibody levels were estimated. The areas under the receiver-operating characteristics curves (AUC) of the models were compared among themselves and to Mayo. Bias-corrected clinical net reclassification index (cNRI) was estimated to assess clinical reclassification using a combined biomarker model. Results: We included 327 patients; 157 from Histoplasmosis-endemic regions. The combined biomarker model including radiomics, Histoplasmosis serology, and Mayo score demonstrated improved diagnostic accuracy when endemic Histoplasmosis was accounted for (AUC 0.84, 95% CI 0.79-0.88, p<0.0001 compared to 0.73, 95%CI 0.67-0.78 for Mayo). The combined model demonstrated improved reclassification with cNRI of 0.18 among malignant nodules. Conclusions: Fungal and imaging biomarkers may improve diagnostic accuracy and meaningfully reclassify IPNs. The endemic prevalence of Histoplasmosis and cancer impact model performance when using disease related biomarkers. Impact: Integrating a combined biomarker approach into the diagnostic algorithm of IPNs could decrease time to diagnosis.
更多查看译文
AI 理解论文
溯源树
样例
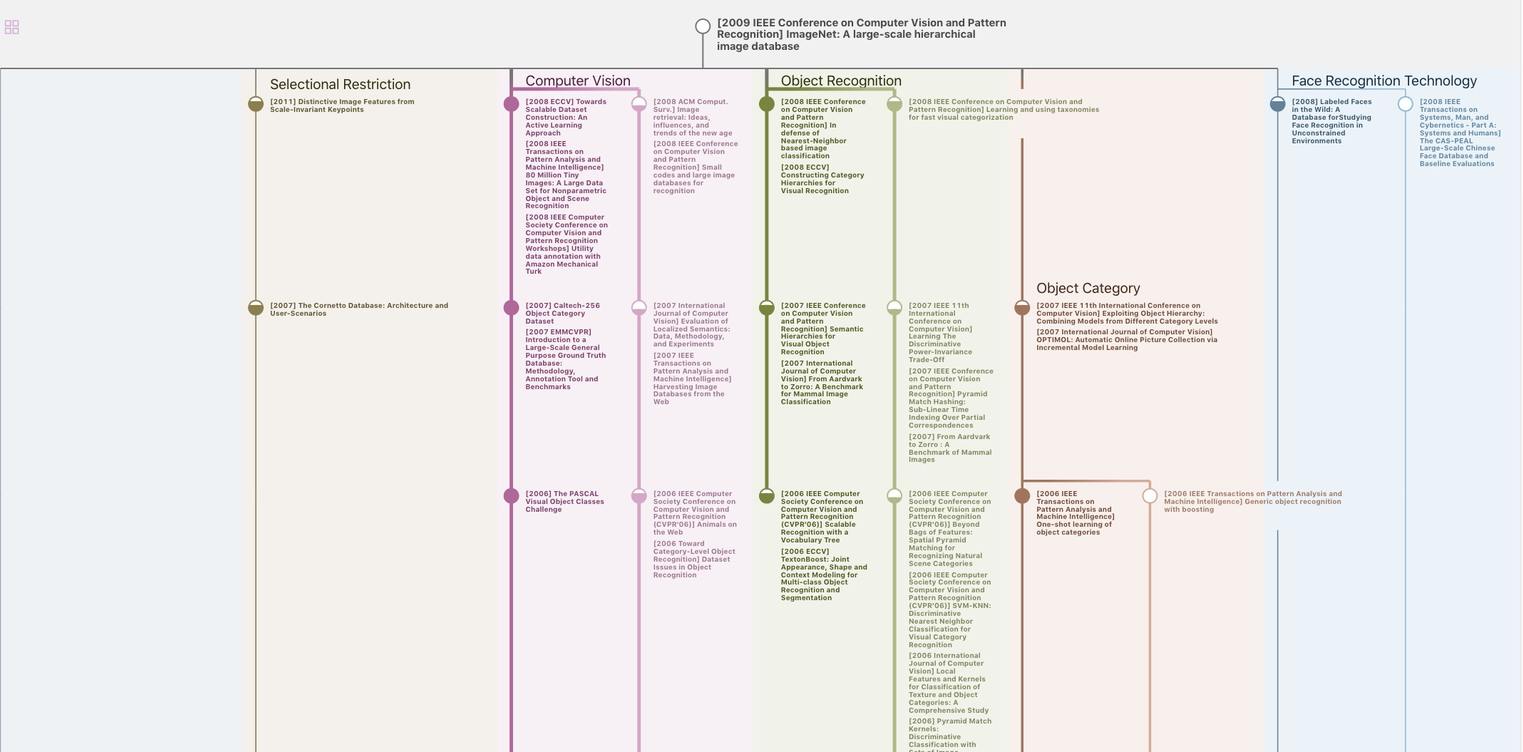
生成溯源树,研究论文发展脉络
Chat Paper
正在生成论文摘要