Machine Learning-Based Classification of Abnormal Liver Tissues Using Relative Permittivity.
Sensors (Basel, Switzerland)(2022)
摘要
The search for non-invasive, fast, and low-cost diagnostic tools has gained significant traction among many researchers worldwide. Dielectric properties calculated from microwave signals offer unique insights into biological tissue. Material properties, such as relative permittivity (εr) and conductivity (σ), can vary significantly between healthy and unhealthy tissue types at a given frequency. Understanding this difference in properties is key for identifying the disease state. The frequency-dependent nature of the dielectric measurements results in large datasets, which can be postprocessed using artificial intelligence (AI) methods. In this work, the dielectric properties of liver tissues in three mouse models of liver disease are characterized using dielectric spectroscopy. The measurements are grouped into four categories based on the diets or disease state of the mice, i.e., healthy mice, mice with non-alcoholic steatohepatitis (NASH) induced by choline-deficient high-fat diet, mice with NASH induced by western diet, and mice with liver fibrosis. Multi-class classification machine learning (ML) models are then explored to differentiate the liver tissue groups based on dielectric measurements. The results show that the support vector machine (SVM) model was able to differentiate the tissue groups with an accuracy up to 90%. This technology pipeline, thus, shows great potential for developing the next generation non-invasive diagnostic tools.
更多查看译文
关键词
dielectric spectroscopy,fibrosis,machine learning,microwave,non-alcoholic steatohepatitis,relative permittivity
AI 理解论文
溯源树
样例
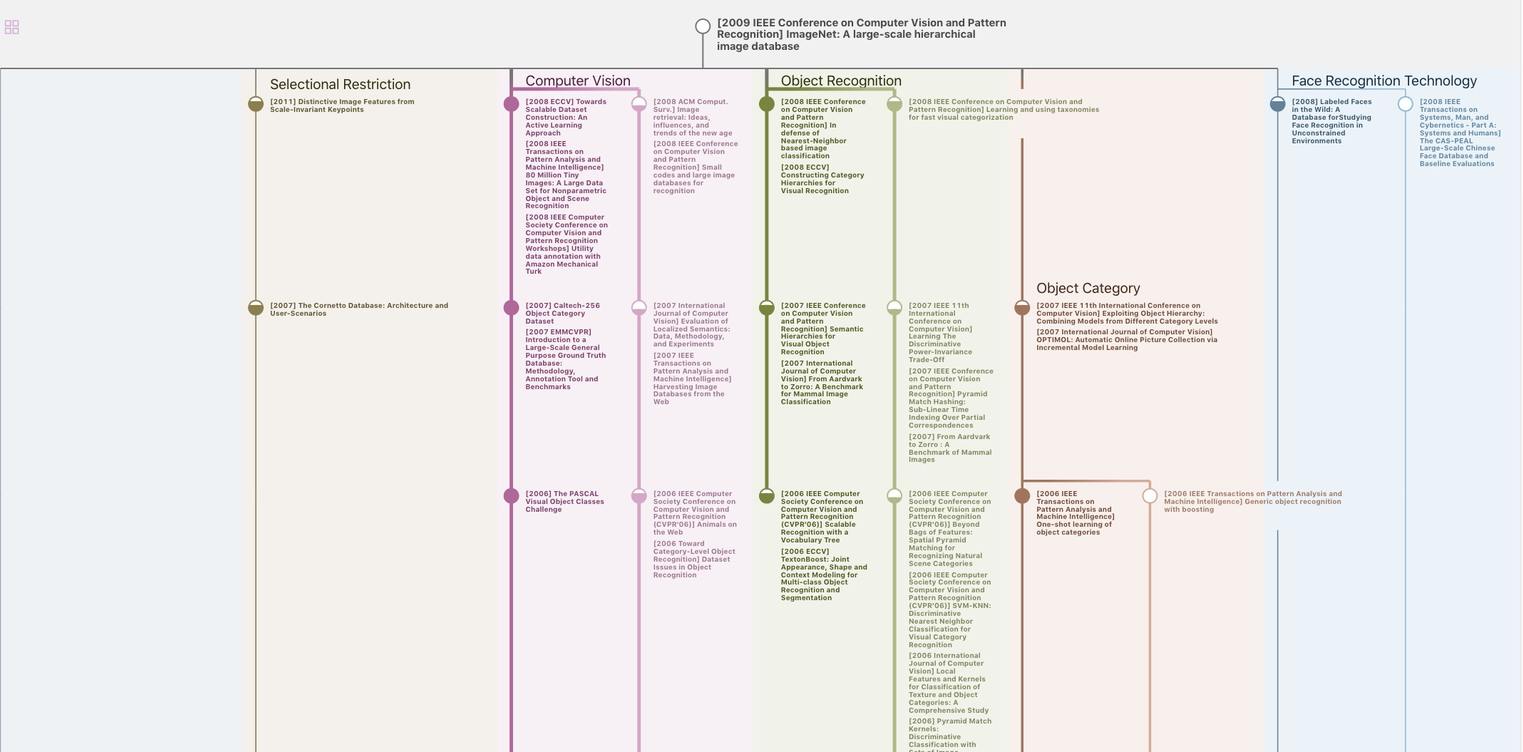
生成溯源树,研究论文发展脉络
Chat Paper
正在生成论文摘要