R-loopAtlas: an integrated R-loop resource from 254 plant species sustained by a deep learning-based tool
Molecular Plant(2022)
摘要
R-loops are chromatin structures consisting of an RNA:DNA hybrid and the other single-stranded DNA, which widely exist among genomes from bacteria to higher eukaryotes and participate in a variety of biological processes (Zhou et al., 2022Zhou J. Zhang W. Sun Q. R-loop: the new genome regulatory element in plants.J. Integr. Plant Biol. 2022; Crossref Scopus (4) Google Scholar). Currently, a variety of approaches to detect genome-wide R-loops have been developed, and ssDRIP-seq (single-strand DNA ligation-based library preparation from DNA:RNA hybrid immunoprecipitation, followed by sequencing) is one of the widely utilized methods (Xu et al., 2022Xu W. Li K. Li Q. Li S. Zhou J. Sun Q. Quantitative, convenient, and efficient genome-wide R-loop profiling by ssDRIP-seq in multiple organisms.Methods Mol. Biol. 2022; 2528: 445-464Crossref PubMed Scopus (3) Google Scholar). However, there are many limitations to genome-wide R-loop mapping based on high-throughput methods. For example, the activity of restriction enzymes for genomic DNA fragmentation; the specificity and sensitivity of antibodies applied in different methods; the technical errors and biological variations; and the depth of sequencing could affect the genome-wide R-loop profiles (Chedin et al., 2021Chédin F. Hartono S.R. Sanz L.A. Vanoosthuyse V. Best practices for the visualization, mapping, and manipulation of R-loops.EMBO J. 2021; 40: e106394Crossref PubMed Scopus (36) Google Scholar). Particularly, when performing genome-wide R-loop detection in non-model organisms, the experimental condition must be re-optimized, which is a time-consuming process. Therefore, the integrated databases of R-loop and computational methods to predict genome-wide R-loops can be used as an effective supplement to the experimental method. Until now, there have been several online R-loop databases, such as R-loopDB (Jenjaroenpun et al., 2017Jenjaroenpun P. Wongsurawat T. Sutheeworapong S. Kuznetsov V.A. R-loopDB: a database for R-loop forming sequences (RLFS) and R-loops.Nucleic Acids Res. 2017; 45: D119-D127Crossref PubMed Scopus (26) Google Scholar), R-loopBase (Lin et al., 2022Lin R. Zhong X. Zhou Y. Geng H. Hu Q. Huang Z. Hu J. Fu X.D. Chen L. Chen J.Y. R-loopBase: a knowledgebase for genome-wide R-loop formation and regulation.Nucleic Acids Res. 2022; 50: D303-D315Crossref PubMed Scopus (9) Google Scholar), and RLBase (Miller et al., 2022Miller H.E. Montemayor D. Li J. Levy S.A. Pawar R. Hartono S. Sharma K. Frost B. Chedin F. Bishop A.J.R. Exploration and analysis of R-loop mapping data with RLBase.Nucleic Acids Res. 2022; : gkac732Google Scholar). The existing prediction methods include the thermodynamic method (Huppert, 2008Huppert J.L. Thermodynamic prediction of RNA-DNA duplex-forming regions in the human genome.Mol. Biosyst. 2008; 4: 686-691Crossref PubMed Scopus (25) Google Scholar; Stolz et al., 2019Stolz R. Sulthana S. Hartono S.R. Malig M. Benham C.J. Chedin F. Interplay between DNA sequence and negative superhelicity drives R-loop structures.Proc. Natl. Acad. Sci. USA. 2019; 116: 6260-6269Crossref PubMed Scopus (64) Google Scholar); the pattern search method QmRLFS-finder (Wongsurawat et al., 2012Wongsurawat T. Jenjaroenpun P. Kwoh C.K. Kuznetsov V. Quantitative model of R-loop forming structures reveals a novel level of RNA-DNA interactome complexity.Nucleic Acids Res. 2012; 40: e16Crossref PubMed Scopus (68) Google Scholar; Jenjaroenpun et al., 2015Jenjaroenpun P. Wongsurawat T. Yenamandra S.P. Kuznetsov V.A. QmRLFS-finder: a model, web server and stand-alone tool for prediction and analysis of R-loop forming sequences.Nucleic Acids Res. 2015; 43: 10081Crossref PubMed Scopus (35) Google Scholar); the formal grammar method rlooperplus (Jonoska et al., 2021Jonoska, N., Obatake, N., Poznanović, S., Price, C., Riehl, M., Vazquez, M. (2021). Modeling RNA:DNA Hybrids with Formal Grammars. In: Segal, R., Shtylla, B., Sindi, S. (eds) Using Mathematics to Understand Biological Complexity. Association for Women in Mathematics Series, vol 22. Springer, Cham.Segal R., Shtylla B., Sindi S. Using Mathematics to Understand Biological Complexity from Cells to Populations. Springer; 2021.Google Scholar); and the hidden Markov model method skewR (Ginno et al., 2012Ginno P.A. Lott P.L. Christensen H.C. Korf I. Chédin F. R-loop formation is a distinctive characteristic of unmethylated human CpG island promoters.Mol. Cell. 2012; 45: 814-825Abstract Full Text Full Text PDF PubMed Scopus (548) Google Scholar). However, a database and prediction tool to support R-loop research in plants is still missing. To cope with the challenge, we developed R-loopAtlas (Figure 1A and Supplemental Figure 1) and deepRloopPre (Figure 1B). R-loopAtlas contains the R-loop data of 254 plant species, among which the R-loop data of Arabidopsis thaliana is obtained by ssDRIP-seq and Karanyi DRIP-seq; the R-loop data of Oryza sativa is obtained by ssDRIP-seq and Fang DRIP-seq; the R-loop data of Zea mays and Glycine max are obtained by ssDRIP-seq; and the R-loop data of 254 plant species are predicted by four deepRloopPre models (the model trained with A. thaliana, with extended A. thaliana, with O. sativa, and with D. rerio, respectively) (Supplemental Tables 1 and 2). In addition, for A. thaliana, we collected the ssDRIP-seq data from 53 samples, which were produced from different developmental stages, under different light and temperature conditions, incthe presence of various biotic and abiotic stresses (Supplemental Table 3). Due to the shortcomings of four existing methods in predicting plant R-loops, we developed a novel deep-learning tool based on neural network and named it deepRloopPre (Supplemental Note). Using the genome sequences of 254 species, we predicted the R-loop data by deepRloopPre trained with four models. We conducted multiple analyses to assess the performance of deepRloopPre. Firstly, we evaluated the R-loop profiles on transposable elements (TEs) and protein-coding genes in O. sativa. The predicted average R-loop level of TEs is consistent with the results of ssDRIP-seq, which are distributed at the baseline (Supplemental Figure 2A). Both the predicted sense R-loops and the observed sense R-loops are concentrated near the transcription start site (TSS) the transcription terminate site, the predicted results of the antisense R-loops are also consistent with those experimental data, and the R-loop signals are both enriched at the TSS (Supplemental Figure 2B). The abundance of antisense R-loops near the TSS has a high correlation between prediction and sequencing data (Supplemental Figure 2C, bottom). However, the correlation between prediction and sequencing results of sense R-loop abundance near the TSS and the transcription terminate site is rather weak (Supplemental Figure 2C, top). Therefore, the predicted profiles could well describe the distribution of R-loops on the TEs and protein-coding genes. Secondly, we used ssDRIP-seq data from rice tissues (flag leaves, calli, spikes, and merge materials) to assess the precision of predicted R-loop locations. Average precision, recall, precision, F1-score, and Jaccard are calculated to describe the accuracy of the model (Supplemental Note; Supplemental Figure 3). We find that deepRloopPre trained with extended A. thaliana has the best performance in both Watson and Crick strands. For example, the predicted R-loops compare with merged data, with an average precision 0.59, precision 0.64, recall 0.59, Jaccard 0.38, and F1-score 0.61 in the Watson strand. Lastly, deepRloopPre was compared with other four prediction tools using ssDRIP-seq data. We employed deepRloopPre trained with A. thaliana, with extended A. thaliana, and with O. sativa, and the parameters for the other four tools are presented in Supplemental Table 6. Through the evaluation, we found that deepRloopPre had a better performance (Supplemental Figure 4). In addition, the results of QmRLFS-finder and thermodynamic law methods have higher precision and lower recall, indicating that some R-loops conform to the QmRLFS-finder pattern and thermodynamic laws. The results of rlooperplus and skewR methods have lower precision, indicating that not all G-rich and G-skew regions could form R-loops. Through the model interpretation, we found that deepRloopPre learned that purine-rich sequences are the key characteristics of R-loops, and G and A enrichment sequences could promote R-loop formation (Supplemental Figure 5; Supplemental Note). All these results demonstrate that deepRloopPre could accurately predict locations and profiles of strand-specific R-loops in the whole genome and that it has a better representation ability for the plant R-loops (an IGV snapshot in Supplemental Figure 2D). Furthermore, we compared the R-loop predicted by the ssDRIP-seq trained model with the R-loop detected by DRIP-seq and confirmed that training the model with only ssDRIP-seq is reliable (Supplemental Note). Together, R-loops predicted by deepRloopPre using four models from 254 species are recorded in R-loopAtlas. Given that our database is comprised of a large amount of R-loop data, a quick engine, which is under the navigation bar “browse,” is provided to browse all these R-loops (Supplemental Figure 6A). R-loopAtlas also provides users a “search” function to query R-loops from both observed data and predicted data by specific gene name or chromosomal regions (Supplemental Figure 6B). In addition, R-loopAtlas also provides sequence similarity search, visualization, and data download. The prediction tool deepRloopPre that was used to generate predict R-loop data for 254 species is also integrated into our database (Supplemental Figure 6C). To better understand the characteristics of R-loops in plants, all R-loop data of 254 plant species predicted by four models are used for further analysis.1)The percentage of R-loops in the genome predicted by different models is not consistent (Supplemental Figure 7, blue circle heatmap). Because different species have distinctive sequences and genomic compositions, there should be some bias when using different models based on various species. Therefore, when predicting R-loops on the target genome, it is recommended to choose species models phylogenetically close to the target (Supplemental Figures 8 and 9).2)R-loop enrichment on TEs was found in 178 species predicted by the model trained with A. thaliana, and among these 178 species, 154 species have TE R-loops that account for more than 50% of all R-loops. R-loop enrichment on TEs was found in 152 species predicted by the model trained with extended A. thaliana, and 131 out of them have TE R-loops that account for more than 50% of all R-loops. Besides, R-loop enrichment on TEs was found in 125 species predicted by the model trained with O. sativa, and 111 out of them have TE R-loops that account for more than 50% of all R-loops. Moreover, R-loop enrichment on TEs was found in 110 species predicted by the model trained with D. rerio, and 84 out of them have TE R-loops that account for more than 50% of all R-loops (Supplemental Figure 7, red tag and brown circle heatmap). These data suggested that R-loops of these species might mainly form on TEs. In the future, R-loopAtlas will be periodically updated, such as increasing the availability of new high-throughput data generated by ssDRIP-seq or other methods and providing more prediction data by deepRloopPre with new genome sequences. In addition, we are planning to develop a new function based on deepRloopPre, which will add a comprehensive model trained by transcriptome and epigenome data. This future tool would predict the R-loop dynamics at different stimuli, developmental stages, and/or tissues, which would be more helpful for investigating R-loop biology in plants. In summary, R-loopAtlas collects and displays comprehensive information of the observed and predicted R-loops in plants. The tools, such as deepRloopPre, JBrowse, Blast, and search function, can make full use of these information. We believe that R-loopAtlas will be a useful, easily accessible, and comprehensive database for R-loop studies in plants. R-loopAtlas is available at http://bioinfor.kib.ac.cn/R-loopAtlas/ to all users without any login or registration restrictions. deepRloopPre code is available at https://github.com/PEHGP/deepRloopPre. This work was supported by the Digitalization, Development, and Application of Biotic Resource Project (202002AA100007 to C.Z.); the National Natural Science Foundation of China (grant nos. 31822028 and 91940306 to Q.S. and 32100428 to J.Z.); and the Ministry of Science and Technology of the People's Republic of China (2016YFA0500800 to Q.S.). We greatly appreciate the useful discussions by all the members from the Zhang lab and the Sun lab. The Zhang Lab is supported by the Yunnan Young & Elite Talents Project (YNWR-QNBJ-2019-268). J.Z. is supported by the postdoctoral fellowship from Tsinghua-Peking Center for Life Sciences.
更多查看译文
AI 理解论文
溯源树
样例
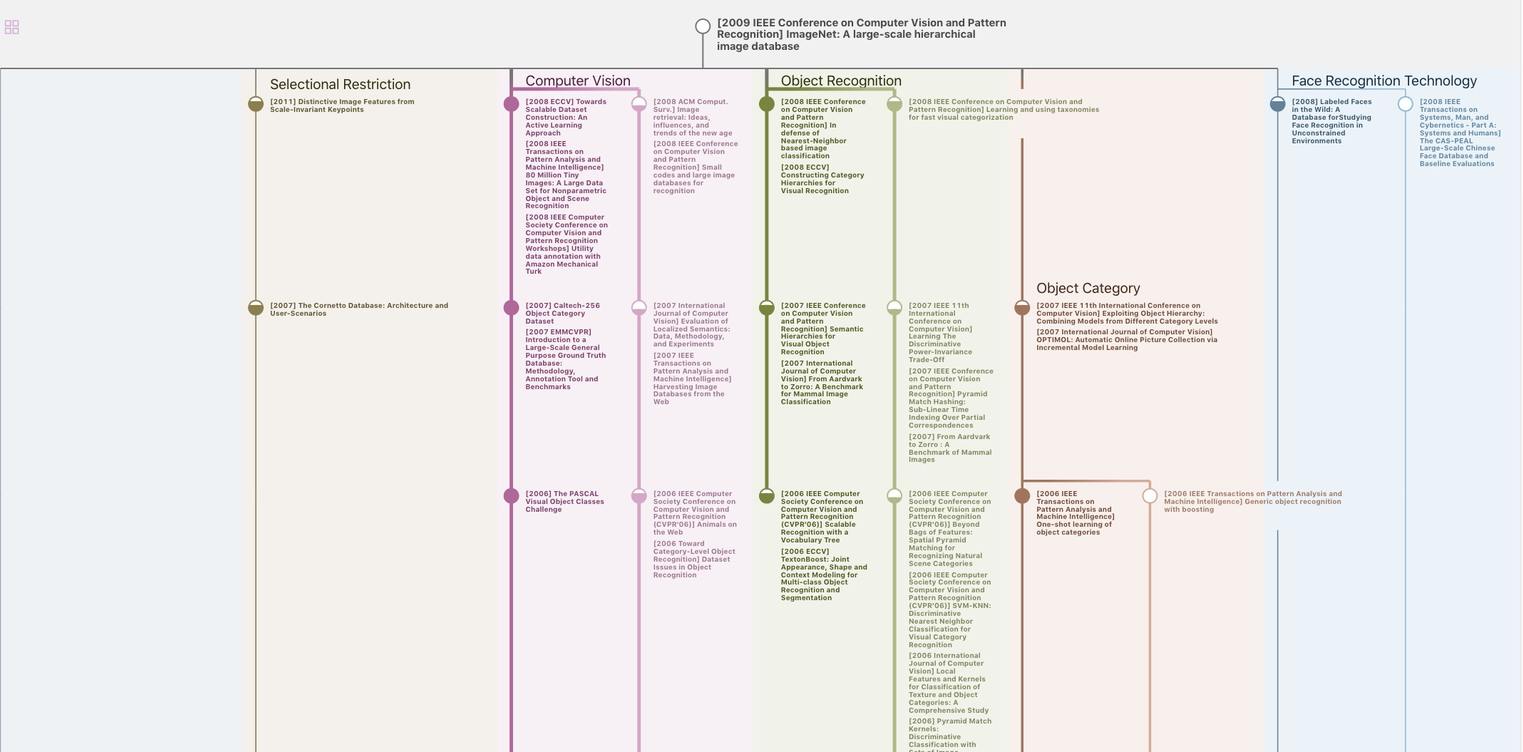
生成溯源树,研究论文发展脉络
Chat Paper
正在生成论文摘要