Fusing 2D and 3D molecular graphs as unambiguous molecular descriptors for conformational and chiral stereoisomers
Briefings in Bioinformatics(2023)
摘要
The rapid progress of machine learning (ML) in predicting molecular properties enables high -precision predictions being routinely achieved. However, many ML models, such as conventional molecular graph, cannot differentiate stereoisomers of certain types, particularly conformational and chiral ones that share the same bonding connectivity but differ in spatial arrangement. Here, we designed a hybrid molecular graph network, Chemical Feature Fusion Network (CFFN), to address the issue by integrating planar and stereo information of molecules in an interweaved fashion. The three-dimensional (3D, i.e., stereo) modality guarantees precision and completeness by providing unabridged information, while the two-dimensional (2D, i.e., planar) modality brings in chemical intuitions as prior knowledge for guidance. The zipper-like arrangement of 2D and 3D information processing promotes cooperativity between them, and their synergy is the key to our model's success. Experiments on various molecules or conformational datasets including a special newly created chiral molecule dataset comprised of various configurations and conformations demonstrate the superior performance of CFFN. The advantage of CFFN is even more significant in datasets made of small samples. Ablation experiments confirm that fusing 2D and 3D molecular graphs as unambiguous molecular descriptors can not only effectively distinguish molecules and their conformations, but also achieve more accurate and robust prediction of quantum chemical properties.
更多查看译文
关键词
small dataset,unambiguous molecular descriptors,chiral stereoisomers,three-dimensional (3D) information,deep learning
AI 理解论文
溯源树
样例
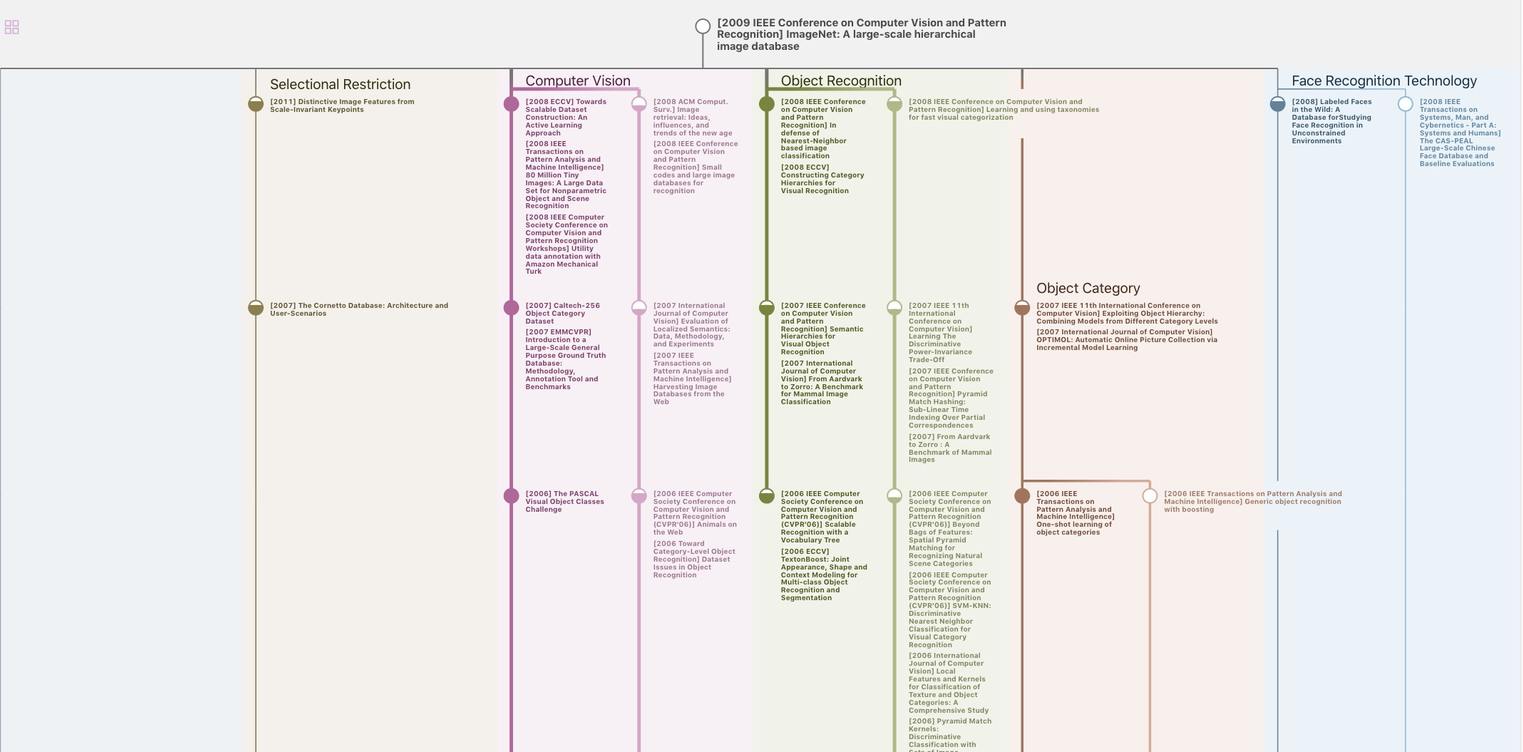
生成溯源树,研究论文发展脉络
Chat Paper
正在生成论文摘要