A fast kernel independence test for cluster-correlated data
Scientific reports(2022)
摘要
Cluster-correlated data receives a lot of attention in biomedical and longitudinal studies and it is of interest to assess the generalized dependence between two multivariate variables under the cluster-correlated structure. The Hilbert–Schmidt independence criterion (HSIC) is a powerful kernel-based test statistic that captures various dependence between two random vectors and can be applied to an arbitrary non-Euclidean domain. However, the existing HSIC is not directly applicable to cluster-correlated data. Therefore, we propose a HSIC-based test of independence for cluster-correlated data. The new test statistic combines kernel information so that the dependence structure in each cluster is fully considered and exhibits good performance under high dimensions. Moreover, a rapid p value approximation makes the new test fast applicable to large datasets. Numerical studies show that the new approach performs well in both synthetic and real world data.
更多查看译文
关键词
fast kernel independence test,cluster-correlated
AI 理解论文
溯源树
样例
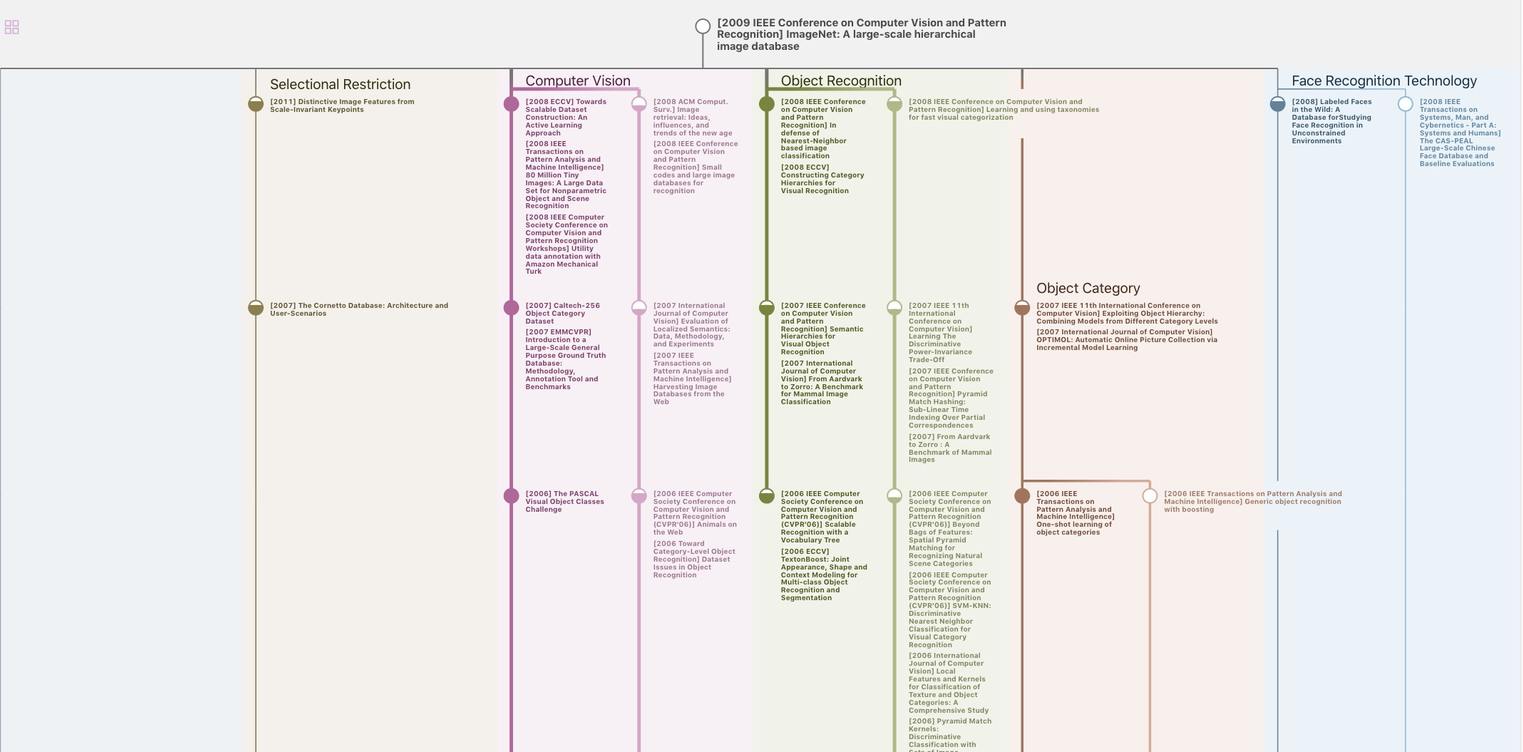
生成溯源树,研究论文发展脉络
Chat Paper
正在生成论文摘要