Reducing the Complexity of Musculoskeletal Models Using Gaussian Process Emulators
Applied Sciences(2022)
摘要
Musculoskeletal models (MSKMs) are used to estimate the muscle and joint forces involved in human locomotion, often associated with the onset of degenerative musculoskeletal pathologies (e.g., osteoarthritis). Subject-specific MSKMs offer more accurate predictions than their scaled-generic counterparts. This accuracy is achieved through time-consuming personalisation of models and manual tuning procedures that suffer from potential repeatability errors, hence limiting the wider application of this modelling approach. In this work we have developed a methodology relying on Sobol’s sensitivity analysis (SSA) for ranking muscles based on their importance to the determination of the joint contact forces (JCFs) in a cohort of older women. The thousands of data points required for SSA are generated using Gaussian Process emulators, a Bayesian technique to infer the input–output relationship between nonlinear models from a limited number of observations. Results show that there is a pool of muscles whose personalisation has little effects on the predictions of JCFs, allowing for a reduced but still accurate representation of the musculoskeletal system within shorter timeframes. Furthermore, joint forces in subject-specific and generic models are influenced by different sets of muscles, suggesting the existence of a model-specific component to the sensitivity analysis.
更多查看译文
关键词
statistical modelling,statistical emulators,sensitivity analysis,Gaussian Process,Sobol,musculoskeletal model
AI 理解论文
溯源树
样例
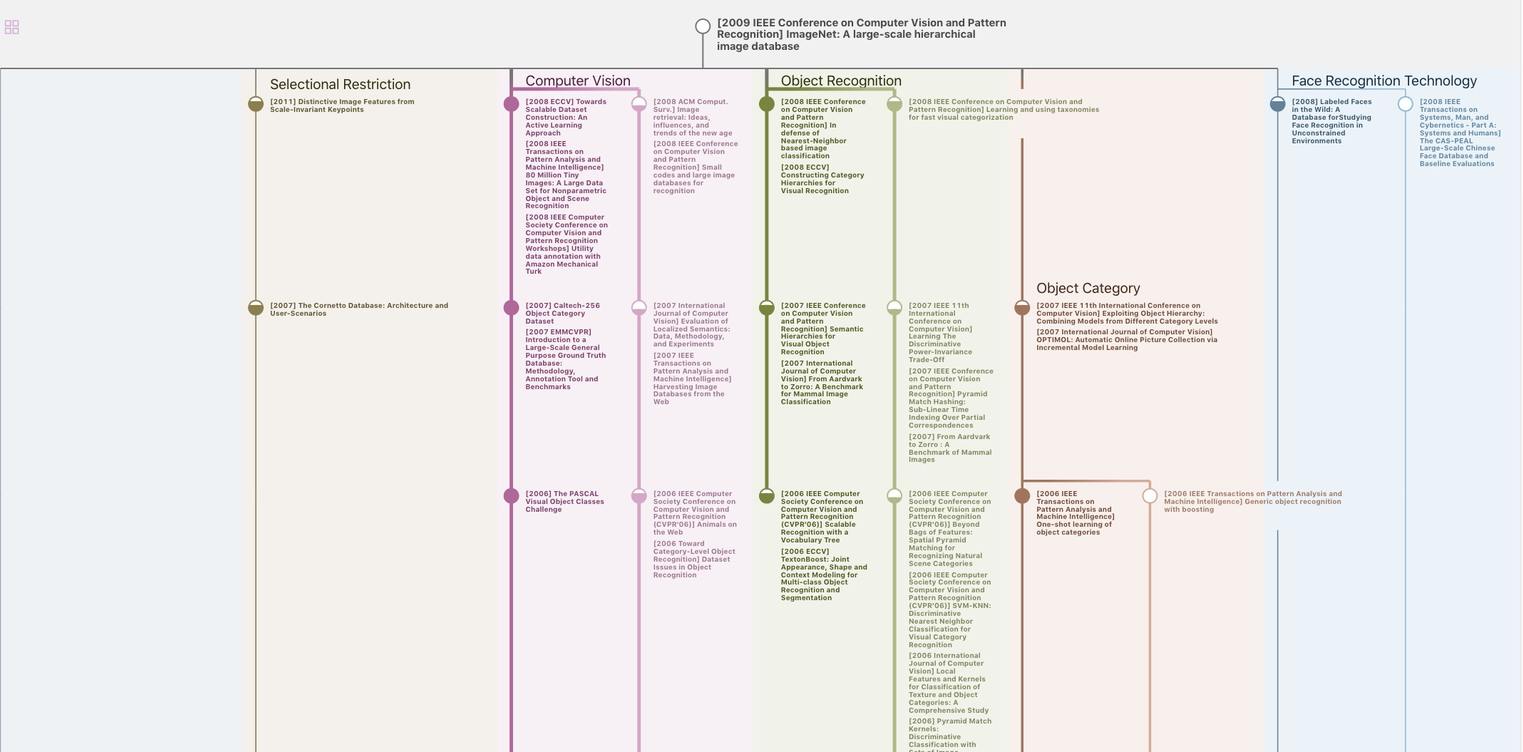
生成溯源树,研究论文发展脉络
Chat Paper
正在生成论文摘要