Optimal budget allocation policy for tabu search in stochastic simulation optimization
COMPUTERS & OPERATIONS RESEARCH(2023)
摘要
Tabu search (TS) is a powerful method for solving combinatorial optimization problems. However, when TS is adopted for stochastic simulation optimization, the simulation noises may mislead the search direction and prevent TS from converging to high-quality solutions. This issue can be mitigated by increasing the number of simulation samples used for solution evaluation, however, real-world applications are generally constrained by a finite computing budget. Therefore, it is critical to reducing the noise effect by an efficient simulation budget allocation, i.e., splitting the total number of samples on solutions. Studies on the budget allocation problem of TS are sparse. Most of the works employ equal allocation or simple policies which are not optimal. Based on the large deviations framework, we propose a new budget allocation policy to enhance the performance of TS under stochastic settings. The proposed allocation policy, provided in closed-form formulas, is asymptotically optimal for maximizing the probability that TS performs the correct move in a single iteration. The efficiency of the proposed method is validated by solving different problems from manufacturing and healthcare scenarios, including an inventory control problem, a throughput maximization problem for the production line, and a physician scheduling problem for the radiotherapy center. The numerical results show that, with the proposed method, TS can obtain better results using the same amount of computational efforts.
更多查看译文
关键词
Metaheuristics,Simulation optimization,Tabu search,Optimal computing budget allocation,Ranking and selection
AI 理解论文
溯源树
样例
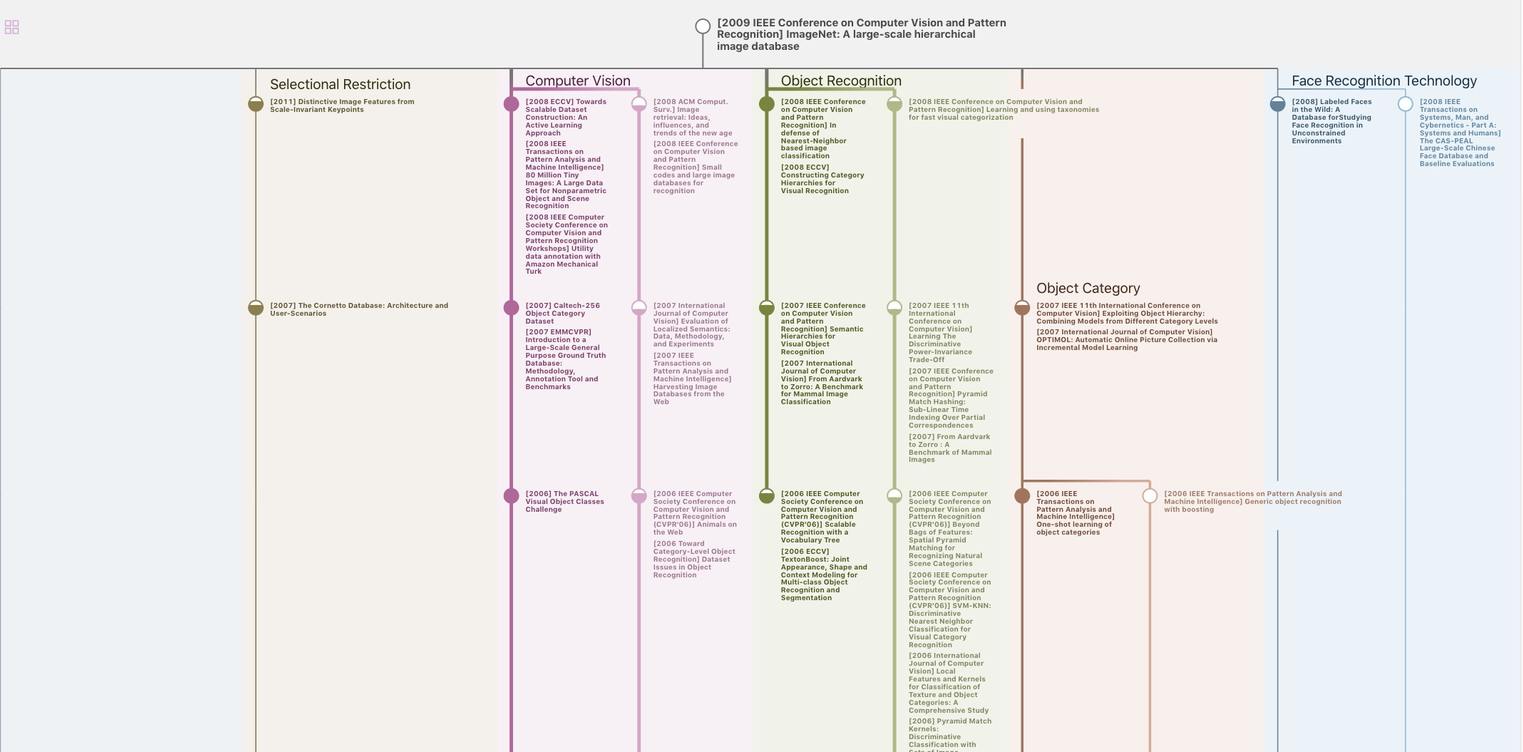
生成溯源树,研究论文发展脉络
Chat Paper
正在生成论文摘要